EP 62: Nick Fornicola from Beusa Energy
0:00 Welcome back. We have been super busy this year. Bobby's, Bobby's been busy. I've been busy, spring break, holidays, all kinds of fun stuff. And so we are now finally back after spring break
0:12 last week. Hopefully we will be. The calendar is looking a lot more consistent. So we appreciate you guys working with us through the holidays and beginning of the year with everything. But we're
0:22 back. Another, another episode of energy bites today. John Calfan, as usual, Bobby's here How are we doing? Doing great. Good to see you. Looking looking sharp. Thank you, Robak.
0:37 If you want to sponsor Robak, we will happily let you. Absolutely. Robak's sweet. Robak and Pancho are basically the GME outfit. That was basically the highway. Yeah, the GME uniform.
0:52 We're here today with Nick Fornacola over at Abusa. He's the director of of data technology. Thanks for coming on, man. Yeah, happy to be here, appreciate the connection. Yeah, glad we could
1:04 make it work when you're in town. Yeah, typically up in PA, right? Yeah, yeah, I spend about 110 to 120 nights in Houston every year, been doing that for the past three or four years with this
1:16 role, so. Okay, so some of those questions towards the end, I can still ask you about restaurants and all this. Okay,
1:23 well, good stuff. Well, I know, you know, sometimes we wanna kick it off for like a current event kind of thing, but I don't know, earlier in the month, it was released like, Mistral has a
1:31 new API that's supposed to be better than anything else for PDF parsing. We can go into any branch of that. I know, John, you struggled with some Olama stuff recently, but just, I think we've
1:41 said it before, PDFs are the bane of pretty much every data person's existence. Yes. Not just an oil and gas, but probably across the board. But just any thoughts there? Well, I haven't used
1:51 Mistral API personally. We do some document parsing with some LOM APIs. It generally worked pretty well, but we were talking a little bit before about just consistency as key in PDFs and
2:09 consistency is not usually the norm, at least in my experience. I'm
2:16 interested to find out more about this and see what it's all about. Well, it's an important topic because, I
2:25 mean, we have been very actively kind of road-showing and talking to potential clients and stuff with Collide and
2:35 for the enterprise side. And we're talking to a number of people who are like, Yeah, we've still got boxes of files that aren't even digitized. It's like how many companies in this industry still
2:46 have boxes and file folders full of important information, whether they think it's important or not, it's still data. And so, as data people, we'd always love to have more than not enough. And
2:59 so it's just one of those things. It's like, first we've got to digitize this stuff, but even then once you do, it's like, now it's a scan page in a PDF, which is the worst possible format that
3:08 you can have. I think that's the beauty of this multimodal stuff now, is like, 'cause we were saying earlier, I mean, I've dealt with marketing settlement statements and I get them PDFs and like
3:17 recently I had a problem because just how they generate the PDF, they looked, to the naked eye looked exactly the same, but you know, they generate, oh, the guy was like, oh, maybe I use print
3:25 to PDF this time instead of the normal way I usually do it and sure enough, now the Unicode error was gone in Tabula, but Tabula should be obsolete in the next couple of years 'cause I should just
3:34 be able to give it my document and say run, but yeah, no, the vision models are just continuously getting better. And it's, I think honestly now most of it is more about, you know, being able
3:46 to detect those kind of outlier, like the scan document use case where you have fuzzy scans or if there's like, you know, faded or bleed through type stuff or stamps, that's another big one stamps,
3:59 just the stamp right in the middle of the, you know, of the important information. And I know you've always loved stamps. Yes. Oh, yeah. Field ticket. Let's get into invoices and field. Those
4:09 are my favorite thing. Yeah. You have to use the stamp. Yeah. That's the most secure way to do it. I mean, what are you
4:15 sitting on Jim's desk? I just had a conversation with a gentleman from a large oil gas operator who indicated that a large number of their documents are still in paper format, which was surprising
4:31 to say the least. It never ceases to amaze me. And normally, it's the companies who have the most money who just have all it's like data debt almost, right? That's just like we've gone and bought
4:41 all this stuff and acquired all these companies. But then you see their CTO at a conference talking about all the cool things. Oh, yeah, yeah. Meanwhile, there's an epic one. They're DevOps
4:51 points. It's been there for three years, scan, scan 40, 000 pieces of paper and log files. And then what do you do with them? Right. Now we've got these tips. Great. Yeah, but I think it's
5:02 interesting too. You know, just the possibilities with, say, any of these kind of multi-modals, you know, things, but like are using it within data pipeline or, wait, I've just got to, I got
5:14 this random PDF and I need to summarize this. Boom, Papa and I can summarize it, or summarization, extraction. Yeah, or just vectorizing, the wind that is just there is context and I can like
5:24 search it like I used to do with maybe the last search if I could get things out of there Yeah, I have OCR, but it's kind of possibilities are kind of endless. It's not just like one use case.
5:33 It's like you can plop these models in the middle of a lot of hardflows. No, I mean, that's the thing, right? People think it's like, oh, I can chat with my document. It's like, well, if you
5:42 can chat with your document, you can also extract key information from that document and then automate that information to go, say into a database, like in Willview or somewhere like that. So it's
5:51 not all manual. And there is so much of that data that we have, the important stuff, whether it's like a DFIT or whatever, that the most important and valuable information goes into a PDF or a
6:04 PowerPoint to die ultimately. And it's like, we can easily adopt these workflows to start ingesting that data, pull out those key values, and then map them to where they actually need to go to be
6:16 useful. And I'm super bullish for that for our industry alone, just that very specific workflow But then, yeah, it's like, okay, well, now I need to categorize these documents based off
6:26 whatever they can do that too. Now, I need to summarize the document or I need to find the kind of needle in the haystack thing of like, well, what was our wellhead manufacturer on that job? You
6:36 know, and it's really, really good at that stuff. I mean, it was even like two years ago, this stuff wasn't really flesh out to where it is. And like, we had a bunch of land documents. We were
6:45 trying to find some producer, whatever 88 or whatever the clause was in like all these things And one of our data engineers did a really good job with like some OCR and like Elasticsearch and I think
6:56 it worked. But like, that could have been a. really quick exercise, if you could just load them all into some of these models, like, yeah, yeah, and it is great as the these, you know, very
7:06 specific and pointed use cases are. What excites me most is these being the enabler for the, you know, very horizontal opportunities that exist that these tools now, you know, right now they
7:22 enable right things that weren't possible before patterns that we weren't otherwise going to see now being pretty harnessable. Yeah. Well, even that, right? Like I've been using a combination,
7:34 I've been using the language model to help me architect and figure out how to solve some of these problems. But like a lot of the time it'll give you, you know, a couple different options and then
7:43 it'll give you like an LLM option if you're trying to, you know, abstract data from a document. But it's like, hey, I've got these documents. I need to know, you know, like what common
7:53 keywords or what common phrases that they have in common, just to start seeing. you know, parallel similarities and stuff. And it's like we can generate a word cloud. People love word clouds.
8:02 People do love word clouds. That was actually one of the parts of the report that it generated as well, which was kind of cool. But it's one of those things where it's like, even like I personally
8:14 tried to not use LLMs for the
8:19 actual execution piece when I don't have to. Because I feel like it got, you know, there's all these opportunities to use them. But if you can do it using Regex or, you know, just traditional
8:32 coding or statistical modeling, which is kind of predictable. Right, yeah. But I think the combination of the two gets really interesting, right? Where it's like, okay, I can use more
8:41 traditional machine learning type stuff or categorization type stuff to improve the quality of how I use the LLMs or how I use my rag or things like that. And there's a lot of really cool stuff. I
8:53 think that's happening in that space as well But absolutely, I think the - You know, the approach that we're taking is this, you know, deterministic or is this generative, right? And you know,
9:09 maybe your use case starts out with just the machine learning model, right? And then you give that model an endpoint, serve it to the LOM, and that's the different sheet, I think. Yeah. That's
9:23 what's going to be crazy in these next couple of years is like, there's all these kind of, there's MCP servers now, there's tools and agents and stuff like that, and people, I think there's still
9:32 a lot of confusion around what agents are, but it's like, you can have an agent that just does one, you know, just searches the internet for you, right? And it's like, I can add, I can just
9:42 bolt that on to you start bolting these agents on, like, and then it's like, oh, well, you know, everyone wants to query their SQL database, but no one knows how to write SQL, at least at the,
9:53 you know, the different parts of the different departments across the company. And it's like, Oh, if we can just drop. text to SQL on top of our SQL and enable the entire company to be able to
10:02 find the data that they want. That's a huge improvement. And I can go pull some of these things off the shelf almost now. And then I can combine that, you know, the SQL database with the language
10:14 part of this. That's honestly where I see like, at least in our industry, a huge convergence. It's not there yet, but I do see it coming where it's like, if we can relate all my tabular SQL data,
10:26 whether that's IoT or SCADA or whatnot, all the way back to the legal documents for the wells that we're monitoring the production on over here, and we can have one single plane of all of that data,
10:39 and it's all related correctly, and it's all available in one platform. That could be kind of crazy, I think. Yeah, it's gonna automate away a lot of the stuff that people hate doing. Yeah, at
10:50 least. But also, some people are clutching too, 'cause that's a good job. That's another very interesting part of this. The message that we are sending internally, right, is
11:03 that it's not a technology project, right? This is very much so People Project. And I think this is probably a common message then. A lot of people are sending their organizations, but let us
11:16 help you focus on the strategic elements of your job and take away the boring stuff, right? That's the true value of Gen AI If you're an accountant, here's what you're actually adding value with,
11:31 like not keystrokes in Excel. It's not manually the from invoices in typing
11:36 PDF that you got invoiced into your accounting system. Yeah, right. Or
11:43 shoring up field ticket invoices with your accounting system and AFEs by reopening them because you didn't get a field stamp in time. But I'll digress on that So that's how I'm about like your, so
11:55 you guys have a gen AI. It's not like initiative or project going on internally. And like what's one of the major focuses there? Yeah, much more than a project, for sure. It's a program and I'm
12:08 very passionate about the differences between the two. Fair enough, yeah. So it is a program we've invested, I would say significant resources, especially from people's standpoint towards it So,
12:20 we basically curated a
12:26 pretty significant backlog of opportunities and kind of categorized them into what makes sense for our business.
12:35 So we have everything from kind of back office opportunities. And you notice I say the word opportunity a lot and it's really a replacement for use case. I'll probably swap back and forth between
12:46 the two, but it's really a pretty good mix of back office opportunities, operations opportunities, kind of spanning across the entire company, supply chain and manufacturing and of course finance,
12:59 accounting, HR, I think is the one everyone kind of starts with. Yeah. But it's kind of a low impact in a way. Like, I mean, like, it's, it's a rag, right? Yeah. It's just simple rag. We
13:10 need a way to chat with some HR documents. Yeah. And I think that one's been probably beat to death, but it's a good way to gain momentum. That's the thing. You need small wins when you're
13:18 deploying any kind of new technology into a company, especially at enterprise or corporate level. Right. And so like, yeah, we could do cooler, sexier things, but it could take longer. There's
13:28 a lot more variants. There's a lot of other, you know, things to it. But like, hey, putting all your HR documents or your personal or one of our use cases with a client or service company is
13:37 procedures, right? It's like, I've you, you were a field engineer. I've been a field engineer. You know that it's like, Oh, shit, I know we have a procedure on this and it's supposed to be in
13:46 this van in a binder somewhere. I don't know where the hell the binder is. I don't know when it was last printed out and of there's no telling. And it's like, Oh, or I could just ask my rag model
13:55 and get an immediate answer with the document cited, right? Like, it's a, no, I think that's where I'm like, I'm super excited about this stuff, especially when you think about the whole like,
14:06 crew change and, you know, whatever the hell oil price you're going to do in the next couple of years. But it's like, you've got this compounding effect, where it's like, everyone wants to do
14:14 more with less. You have less experience in the industry than you've probably ever had. And that's continuing by the day But so how do you upskill, you know, a junior field engineer to a senior
14:24 field engineer or a junior tool pusher to a senior tool? You know, like, how do you do that? And I think giving them the ability to access the data, the information that the company has continued
14:35 to grow over time and the knowledge of the people in the company, both current and past is a huge thing that we'll just aren't, they're not thinking of it that way. And it's like, man, this,
14:47 this little rag project could significantly change my operations, my hiring. has a waterfall effect on most on a lot of these things. Yeah, I'm sure you probably heard it, right? Gen AI won't
14:58 replace people, but people who use Gen AI will replace people who don't. We understand that as tech people, but you can't just go to your organization to say that, right? No, you can, but it's
15:12 probably gonna fall on mostly deaf ears, right? So you have to deliver the message in a certain way that resonates, explain to what's in it for me, for everyone, and then get those quickly. I'm
15:26 at the point where it's like outside of some security concerns, wherever like, why wouldn't you just be enabling anyone who can write some Python or any of that stuff, like in the business with
15:36 like cursor or something along those lines, like why wouldn't you be, I mean, that's a low investment in the outsides returns on and stuff like that, where like, well, that's always been the
15:46 amplification of like people Yeah, those are your multipliers, absolutely. Well, they're not in your department. They don't let, you know, not everyone who knows how to use JAIs in my
15:57 department, right? Yeah.
15:60 So those are your ambassadors, right? And you give these people these tools and let them operate in it with, you know, in a certain set of confines and a framework that
16:12 can really expedite the progression of your program. I mean, you've got people in the business that are still awful at using Google. Much less like, no, how to write a prompt You know, I think
16:21 that's even worse, like even like, and maybe this is part of the program too, where you're trying to build up literacy within the business, like, I mean, are there prompt engineering, you know,
16:30 courses that you're providing to folks in-house? And then also, I think some of it's just the system prompts that you put behind it too, right, that help make what they're sending in better. But
16:39 some people will just ask a stupid question to it. And like, if you give it no context and it's one sentence, like, it could give you anything. Yeah, the fix this prompt is not a good one Yeah,
16:49 come to find out normally. Yeah, there's, I mean, absolutely, the training element is pretty important as well, right? And it's not just prompt engineering. It's like, here's what these
17:01 things will do, right? Right, you got to - They're very confident. Yeah. They're very confident. And if you give it even a document and that document just exists in context of your conversation,
17:13 there's no, nothing saying that it's gonna continue telling the truth after 10 messages, 15 messages, you know, depending on what model you're using. I think that's the biggest thing too, is
17:25 it's like we implemented memory and on the collide chat, I don't know, a while ago. And it's good, right? On one hand, when you're talking about the same thing, but then if you context switch,
17:39 then it's like, how do you make sure that the memory isn't, understands that you have pivoted to a different question in the same chat window and doesn't use the. prior context, because I didn't
17:49 realize that about chat TBT, like between conversations, like it knows everything from all the other conversations. So like until recently, I was like, oh shit, it remembers some things
17:59 from other conversations that I've had with it. So it's, no, which is cool, but you often know that too. No, there's so many of those things, right? It's like it has, does it have memory or
18:10 not? Okay, it does have memory. So understand that it has memory when you're using it. And if you want to get out of that, maybe open a new window, a new session and get rid of that one. Or,
18:19 you know, like, just the prompt piece, right? Like, especially, actually, this is a really great question 'cause you're one of the few people I've talked to personally that is directly doing
18:31 RAG in an enterprise. But it's another thing that I've noticed with our, you know, 'cause we have two version, we have the community version and we have the enterprise version. On the community
18:40 version, right? It's more general knowledge kind of questions, but then the RAG version is specifically on their documentation, right? The questions that people are asking the community model are
18:50 very different than the questions that people are going to ask our model, but then people come into their enterprise model and they don't know that it's just on their documents and they're asking it
18:59 general questions and you're like, No, switch your source and then ask it. That's not what this is meant for or that contracts one that I was telling you about If they ask a very vague, like,
19:12 what was the interest on this contract, it's like, Which contract? It's got a lot of contracts, right? You've got to be specific enough to understand how these things work, but I think the
19:23 biggest thing is just the context, right? People don't understand that the more context you give it, the app, it completely changes the way that these things will respond as well as the system
19:34 prompt and the temperature and the parameters and stuff, those things matter and they make a scary amount of difference It's actually to the point that once you get into that and you're like, Oh,
19:43 now we need to track this stuff. We need to evaluate performance and all that stuff. Yeah, it becomes a lot of overhead really quickly. Some of the challenges that you're talking about, I think,
19:55 can partially be solved through a cleverly designed UI, right? Your
20:01 conversations getting long, potentially switched to a new chat window, or I think chat GPT has a similar type message, or on the free version, it would tell you to, or tell you when your GPT-40
20:15 tokens were out, you know, you're getting switched to GPT-4, which the answers, the quality was significantly different, especially with coding. Yeah.
20:27 Speaking of coding, if you use 37 clog sauna, yeah, it's pretty legit. It's pretty good, yeah. It's, now I use that with cursor. That's my kind of go-to stack right now, and it's, I've also
20:39 used it with eight or a lot. That's another one, it's not as popular as cursor, but I like 'em, I like 'em both for different reasons, but yeah, Sonnet has been. awesome. Yeah, it's been so
20:49 good. I think that's another piece though, that like a lot of people don't realize is that different LLMs are good at different things
20:56 and they're not all built the same. And like if I want to categorize something that I don't care gets into the public domain, I'll use Jim and I flash because it was built for catarization, like is
21:07 so good at that. Whereas if I want something, you know, code right now, in my opinion, the best close source model is Claude. And then now you've got the deep research and the, I remember what,
21:21 what open AI is calling their newest one, but it's focused on like writing and yeah, research type stuff. But that'd be 45. Yeah. Yeah. I haven't messed with it yet, really. So you're talking
21:33 about a bunch of things in my perspective that are the responsibility of us as AI leaders in our organizations, right? So all of these things, which LLM to use. how do you prompt correctly? What
21:50 are, when's the
21:53 right time to go to in your chat window? These are all our responsibility. And the way you approach it is probably unique to you and your business.
22:05 For most, it's gonna be training at least as I see it, right? It's not
22:10 one lunch and learn that you do. No. Yeah, it's like. And you have to, you have to, probably almost needs to start being almost like safety moments where like an AI moment for ever meeting.
22:19 Like, yeah, that's actually a really good idea. Yeah, that's a good idea. That's not top of my head, but yeah. But
22:25 it's like, you have to be very strategic about the order in which you teach this stuff because you're gonna lose people pretty quickly. And for something that's supposed to be transformational,
22:40 it's a pretty risky thing to do. Yeah, AI office hours is something I might have to do that. Yes, so we've done things like that with not specifically with AI, but like an office hour is tight.
22:52 Yeah, yeah. Tight four minutes out of it, Grace and Melo's, you got some really good stuff coming out of that. Yeah, a lot of people come out of the woodworks that never engaged with you before.
23:01 Yeah, yeah. And it's extremely effective for rotational people, right? Like, hey, you're on days off, right? You know, these two weeks, these guys on nights, these two weeks, like, you
23:12 know, you got to put yourself out there and maybe host an office hours at 5 am, but, you know, that shows your dedication to their learning, right? So for other initiatives that we've had,
23:26 we've done those office hours and they've been well received and pretty effective, at least in getting in touch with people that we normally otherwise wouldn't. So let's get into kind of some of the
23:37 architecture, data architecture behind some of this. I mean, just 'cause, I mean, I'm sure you've got just straight up data architecture from traditional data warehousing and BI type stuff. but
23:46 then also now integrating some of this AI stuff. I mean, are you guys, AWS Azure type, you know, the shops or on-prem and then like, how are you kind of, you know, integrating these things
23:56 together? Yeah, so good question. We have a, I think we have a
24:04 relatively clean environment.
24:07 So we actually just spun up our lake house last year with Databricks, which was somewhat intentional going with Databricks over snowflake or, you know, someone else or fabric. I mean, we looked
24:22 at fabric, but it's not there yet.
24:26 Were there any specific or like primary reasons behind that that just because a lot of people are in that boat, right? Like, all right, which one does it stuff like Databricks? Yeah. So no, I'm
24:38 Bobby fabric. It's brand new, it's brand new. Something like an Databricks. It's renamed and then a GCP over here that no, I was touching So we had some. We had some particular insight from some
24:49 folks who we know at Microsoft that basically told us that fabric was being pushed maybe a bit prematurely. And some of the things that we wanted to do at scale, like IOT data weren't really well
25:06 supported. So that was one of the main drivers. So we landed on Databricks in a medallion architecture, I think probably works for 95 of companies unless they need, you know, what is it? Kappa,
25:20 like event-driven type architecture. And we, we actually don't, so in terms of real-time IOT data, would, I mean, yeah, I think about consumption, right? Like these things aren't cheap. Oh,
25:36 no. Yeah, I mean, we'll especially grow out to you. Or out to you. Or out to you or that, I mean, it can rack up quick. Well, so do you all have, is, are things all under abuse, or they
25:43 kind of, I guess they're split up. Bye. company with Envusa, right? Well, role-based access, right? So it's one tenant, which keeps everything. So you've got a ton of different types of data
25:56 too, coming in as well, because you've got full operational operator data, and then you've got full service company data. It's like, it's on the data. Yeah, and all the back office stuff. And
26:07 it gets pretty interesting. Yeah, I can only imagine. Some of the models that, I mean, that we can generate with that type of setup.
26:19 But we, so we have everything kind of under one roof, so to speak, right? And our real time data is, it's just kind of off on its own, not actually in the Azure Cloud. It is, but it's on a
26:36 separate VM, right? So it's kind of a consume as you need it, at least as far as Databricks is concerned. You're not just dumping everything into your data lake, like everyone else, and then
26:48 hoping to figure out what to do with it later. Yeah, it was the point. That's a good idea. I mean, I think I just applied to a guy from Databricks here today. Or actually, I think there's a guy,
26:58 MIM, Joe, or whatever, he's an Australian guy. But he was big at one point, like testing all the different engines, and now he works for Microsoft, talks about the fabric stuff And then Mike
27:09 Cornell from Databricks kind of like, you know, refresher, but it was kind of to that point of like, why are you refreshing this data if you're not using air people are trying to do real time
27:19 stuff? And like, but no one actually needs the real time. Like they don't need it every second. And I replied, it's like, yeah, 2 am. I mean, like, I think it's a big way that we saved a lot
27:27 of money at Grayson Mill with Snowflake was like, I didn't run my dbt pipelines outside of business hours unless there was a use case Like, this is like, I don't need to refresh it five times
27:37 overnight if everyone's sleeping. Yeah, like just rocking up snowflake spend for no reason. And same thing with Databricks now, or keeping warehouses or whatever they call it, compute on, like
27:47 there's no need, like. Yeah, I think there are, in my opinion, is there are very few real needs for like actual streaming data, you know. There are some, but not many. Yeah, and I mean, I
28:04 think that's, like you said, like you said, responsibility of like folks like us, like it's our responsibility to challenge them on that And I don't, it's, you know, I've always tried to stay
28:14 away from being like an IT guy, you know, like, 'cause I mean, I want to enable people, but like you have to, at the same time, challenge people is like, do you really need this? Like, you
28:21 know, and if you do, like what, you know, let's outline this. And then like, if so, how can I help you get there? Or are there better ways to do it? Right, well, it's also, I mean, it's,
28:29 we've grown across it multiple times, right? It's like, oh no, I need all, all one second pressure data. I need every single point for my two month be fit on the plot even though there's not
28:39 that many pixels on my screen. or, you know, and it didn't change. It literally didn't change within a hundredth of a - It changed, PSI, like PSI in two days, and you wanna see every single dot.
28:50 Okay, yeah, just in case someone actually vibrated the wellhead and the window to some more noise, yeah. Yeah, yeah, and I mean, I think to implement those things effectively, you need a
29:04 healthy culture at your business, and to your point, like, yeah, challenge the individual and luckily, you know, abuse is a place where we can have those healthy conversations. Yeah. And
29:15 people generally are, like, hungry for the right solution. Yeah. They might have an idea of what they think they want when they come to you, but, you know, explain it in a way that makes sense.
29:26 Yeah. And you usually can reach a logical conclusion. Yeah, well, it's like,
29:34 you know, what I want streaming data 100 of the time, if it was free from a dashboard perspective Sure. Yeah, but it's not. Yeah. It's like, oh, even now, I mean, like, yeah. I'm starting
29:43 to look at the start of the independent consulting and stuff and it's like what I picked a couple of years ago worked really well, but like five trans gotten really expensive, but also a obfuscated
29:53 like price per. Per connector is different all these things so it's like predictability of pricing is all over the place and it's just expensive in general like what are better alternatives now like
30:02 yeah No, I really I hate that everything is going well, part of me does part of me doesn't but like the whole consumption model thing is. I feel like we're swing advertising or it's a trap. It's a
30:14 trap. Well, you've got it. Tising people from using the product. Right. Well, it's like paper usual. Because I mean, even like when DBT cloud changed their pricing model, luckily we end up
30:23 being grandfathered in. Right. I like to think I had a little bit of credit because I was given Tristan Handy the business on their Slack channel But like,
30:33 you sit here and told me for multiple years, like break your big SQL queries into small components. And now you're going to charge me per model run. Yeah. Now you're just going to force people
30:41 back. into a model that you said was not optimal to start with, but profit. And then that's the thing. I mean, like all of a sudden now they've got to answer to investors, but now I'm even
30:52 seeing things talk to this guy with company Graybeam the other day.
30:57 And they're doing something where like now with like say DuckDB, it's almost like a middleware between your BI tools and Snowflake. And basically identifies, can I run this query with DuckDB? Or
31:10 can I pass it on to Snowflake? So like basically they can reduce yours, snowflake can build by like 50 to 70 because like it's kind of going to sit there and like say, oh, you don't need to run
31:18 this against there. We can just run it for you, you know, right here. Oh, nice. So it's like there's all these kinds of solutions now where it's going to be like, and I think that, you know,
31:26 where Databricks can either the original Lakehouse kind of thought, but now with iceberg in all iceberg tables, it's like, now that you have this agnostic storage layer, and if I want to use
31:37 Databricks or Snowflake and DuckDB or whatever else, you That feature's not far off, like, yeah. And what was that company's name? Graybeam. Graybeam. There's focusing on stuff like
31:49 specifically. Okay. Currently, but it's interesting. Yeah. I think it's interesting. Like there's been so many of these like DuckDB and stuff that have popped up where it's like, hey, we're
31:60 gonna make this a lot easier. Yeah. Not easier necessarily, but way more efficient. Well, we're - As far as like the analytics. I mean, because I mean, it works really well and like what, I
32:08 mean, there was that white paper from I think five trans CEO where like they had all the data from Redshift and Snowflake or whatever queries and it was like, you know, the bell curve was way over
32:17 here, like small data. Like no one's really processing terabytes of data consistently. You know, like not even hundreds of gigabyte, you know, like it's usually like a lot smaller than that.
32:27 And like now that you've got an engine like DuckDB that can run on your laptop that can process that like crazy and it can connect to your object store and do it. Like so, and as we're like, mother
32:36 duck, Please figure out your - ODBC drivers and everything, so I can, you know, connect, you know, better. But like, even there's where it's like, it'll run duct to be locally. But then if
32:47 it needs to go use cloud computer, it will. It's like, there's all these options now where like, you don't have to, or decentralizing. But again, like, but again, like, I expect the original
32:56 point of like, consumption based pricing, now people are finding a way to work around it. Yeah, whatever, whatever pricing model you're going to trap me in where we will find weight. I mean,
33:07 since the beginning of time, Napster, for example, I hated paying12 for you. So you said it was pretty intentional choosing Databricks. I mean, outside of just the fact that fabric wasn't ready
33:16 for prime time. Can you get into that? Because I mean, like, I agree, I think Databricks is them and so like are converging on the same platform from different directions, I think, in a way.
33:25 Yeah. But I mean, I do think they have the most comprehensive, you know, tool for, like, say, data engineers, analysts, you know, traditional BI data, you know, data science, they can
33:35 come and do all that within the platform. So yes, you hit it. right there, right? So the the data science, you know, the natural integration with ML flow, you
33:46 know, it kind of worked out that now they're doing model serving and things like that through their platform as well. Jeannie is pretty cool. I don't know if you've used it. It's their Texas
33:56 sequel, which they actually just released released an endpoint for it. So you can bring Jeannie to your application now. Yeah, that's awesome. That's, that's like, again, it's one of those
34:07 bolt-on things that literally every company will benefit from. It's like, okay, you can have your marketing person query the SQL database without having to ever know SQL and get their information.
34:18 Right. Yeah. Yeah. Democritize. No, I will say that. I think a lot of Texas SQL, because I was talking to, so there got Ian Tracy with turntable and he's working on kind of like a fully, you
34:29 know, integrated like, you know, EL all the way through like, kind of a BI front end, But like, thank you. for the Texas SQL to work while you do that semantic layer between like the data
34:40 warehouse. Like if you can't just have a data warehouse, in my opinion, and then just do Texas SQL and it figures the shit out. You know, like, where it's not gonna do a good job, but if you
34:47 have that semantic layer where you have those connections, you know, established that this is your keys and this is how this all relates, you know? And the ERD, yeah. And that's where those
34:57 things can really, yeah, the quality of your metadata is equally as important to the data quality within the warehouse, right? And that is true for all language model stuff. I will just say that
35:12 there, the difference in you naively going on the chat GPT and throwing in 10 PDFs and chatting with them versus doing that at scale over thousands of documents, the ETL on that data is the most
35:25 important part of it at the end of the day, especially for a rag. And so all that metadata stuff is incredibly important and most people don't have that And that's where a lot of the engineering
35:34 goes into and a lot of these. enterprise type applications or big LLM type applications is that people don't realize that right like they think it's magic they throw a document in there and it
35:43 magically gets everything right it's like no they don't get everything right yeah they don't understand relationships across things and you've got to you've got to do a lot of work to do that so you
35:53 guys serving your gen AI out of Databricks are using Databricks to serve the models or how are you all handling that yeah so kind of um so Databricks is a very I mean it's very powerful obviously but
36:07 it's it's dev-heavy right you know just go in there and drag and drop so no for sure the more complex use cases leverage Databricks but we have a different platform that's a bit more user-friendly it
36:22 makes the the AI engine your role a bit a bit simpler and you know you can kind of serve those Databricks models through this other platform so that's an in-house platform or no. I just, are you
36:37 able to say what it is or is it kind of? If not, that's fine. I mean, I can say, we can always get rid of it, but Abacus AI. Okay, nice.
36:48 Super economical platform. Okay. If you haven't checked them out. Yeah. I mean, I don't know what use for it, there may be here, but very, very legitimate platform. They have
37:02 like, if you wanna use it on a personal level, it's 10 bucks a month, you get, they call them compute points, they don't match one to one to tokens, but it's because they have 18 LLMs that you
37:16 can use, and you get two million compute points, which is like 50, 000 messages.
37:25 They have a route LLM, so if you don't know which LLM to use, it'll just route you to the right one based on, of course, what they think you're out there trying to. And then a web search and they
37:38 have AI agents and a computer agent and you all for 10 books a month. It's pretty loud. And they don't do a lot of marketing,
37:49 which is really surprising. But I mean, obviously there are enterprise subscriptions a little bit more expensive, but and you get more stuff. AutoML, that was big for me when we chose a platform.
38:02 How was that been? I'm curious. I haven't played a ton with it
38:06 We haven't played a ton with it either. My perception of it right now is it's going to depend on the complexity of what you're trying to do. We always have Databricks as a backup for it. I
38:20 think in my head, context clues I can figure out, but can you talk about AutoML, just in general, what a workflow like that would look like for people? I, to
38:31 put it succinctly as I see it, it's kind of drag and drop. ML, right? Rather than import SK Learn and do your test train split, they, a lot of companies have kind of packaged all that up into a
38:46 pretty convenient UI, right? Most companies now, they're actually auto generating all the code to where you can see it, you can accept it, or you can go in there and edit it yourself if you want.
38:59 That's one of my, you're talking about companies generating code that way, cursor, I've noticed now, and their release notes will put what percent of the release update was generated by their
39:10 agent. Like AI generated, and how, yeah, I was like, it was pretty slick, you know, to not only break, to say that it's being generated, but also track how much and publish that. I think
39:21 it's fascinating to see how that trend ends up growing or not, depending on who it is, but it's, yeah, no, I agree with you. I think, like, layering, democratizing it. the LLM stuff even
39:36 further is, that's like, yeah, okay, I can use an LLM to generate code, but how do I productionize this code or how do I distribute this code so everyone can use it? That's a whole nother jump
39:46 in things. And then you've got, there's a bunch of them.
39:51 What is it, anything LLM, there's Flow-I's, there's a bunch of different, there's no code agent-type tools out there that you put in your API keys or you do your integrations and then it's all
40:05 drag-and-drop, GUI-based kind of coding and stuff. And I think that's, and then they have templates and stuff too, right? Well, it's like, oh, I need a text-to-sequel agent. Okay, well, put
40:16 in your stuff here and then it's done. And so like, I think once people start rolling those out within corporations, like, holy shit, the things are gonna be crazy. Yeah, yeah. But then it
40:27 comes back to the whole, you know, the training and teaching them the art of the possible. Like, it's gonna end up still being the same people, but like it's pretty almost. Almost like it wasn't
40:34 during COVID. We're like, Oh, the people with the money, their kids got way smarter. 'Cause they have a small group,
40:41 private tutors, and other kids didn't touch a book for two years, where it's like, the rich are gonna get richer here, 'cause people that are not afraid to play around and know what technology to
40:51 use, they can just, obviously they could probably do five, 10 people's jobs. Yeah, I mean, give them a sandbox, let them do their POCs, and you set it, you still need someone to put all the
41:03 pieces together, but I can create a script, but every time you wanna run that script, you don't wanna have to go into VS Code and run. Yes, exactly. So you still need a number of people to
41:16 orchestrate the deployment, right? However you're gonna deploy it, a web app, whatever it is, it's, as drag and drop as it is, it's not truly drag and drop. Well, and that's the thing and
41:29 it's like, I mean, I use some of those tools for like the dirtiest. quickest proof of concepts just as like we had, you know, we had jobs on collide initially. And I was trying to figure out
41:41 because, you know, we had like, we were scraping 20, 000 jobs a week or something stupid like that. And now that's a data problem because from a GUI perspective, because how do I serve up 20,
41:51 000 jobs and get the user to the job that they're looking for within a few clicks or searches, right? And so it's like, oh, I've got 20, 000 jobs and that's a lot, you know, we just had like a
42:02 generic table Well, I wonder what this will look like if we just dumped it. If I very naively ragged this and just played, you know, did it through an LLM. I was like, oh, shit, this actually
42:13 worked pretty good. I did no metadata, no, nothing. Just naively dumped it and tried and it was pretty decent. And so I'm like, oh, and I did that through one of these loco tools within like 30
42:24 minutes. You know, it's like that to me is, I mean, that's how we kind of do RD here I script something, I'm focused on one thing and whether it's. a loco tool or cursor or whatever, I come up,
42:36 work on the solution, and then I go to my dev team and I say, okay, here's kind of where I've gotten with it to whatever point to completion or not. And then they take it, productionize it, and
42:47 roll it in. And so it's one of those things, it just enables that pace of development or idea to POC so quickly. Yeah, yeah, and the thing that, like the thing that you're always gonna need are
43:01 the curious people, and
43:05 the people with the know-how, or at least the awareness of what they wanna do, right? Just because anyone can come in here and drag and drop a forecasting model or something like that, like they
43:18 have to know what they need, they have to know what exists, so that everyone's kinda concerned about the technical skills that are gonna be eroded because of stuff like this I don't know.
43:31 necessarily think so. I mean, I think probably a little, but people said the same thing about Python versus C, right? I mean, like, who cares? Yeah, no, it's an evolutionary development,
43:45 right? Like, it's, no, but I do think like the people who understand them and who are using them and who are, I mean, it took me, like, I want to say it was a week or two where I actively had
43:56 to focus on, okay, I've got this problem. Instead of doing it the way I normally do it, I'm gonna go through the language model or cursor or whatever and see if I can solve it that way. And like
44:06 just getting in that habit of, 'cause that's how you figure out what these things can and can't do and what their capabilities are and where the boundaries are or how to better prompt it and all of
44:15 the nuance behind these things. And if you don't play with them, you don't know. Well, and it's like, some of those problems that could be solved, like, there's no reason for you to be spending
44:22 an hour banging your head on certain - Right. If you haven't tried to ask some kind of LLM or something for that. Like, yeah. Yeah, there's, there's. countless times I, I just got hung up on,
44:35 you know, one, one, one part of a project and, you know, it was before JA I or whatever. And yeah, you just think back to those times, you're like, yeah, now you gotta go, put it on Stack
44:44 Overflow. And yeah, hope that someone gets your answer. You could, uh, you could put a picture of you know, as a beautiful lady on cyberflow. And really all the nerds will come out and give you
44:54 answers. That should have been our stories at the beginning. I linked to John someone put that on, uh, LinkedIn is it was later a couple of days ago, but it was what they were part of the same
45:04 company, right? Yeah. And this guy, the developer created this fake stack overflow account with a pretty girl's face on it and would basically pose as like, uh, naive. Oh, I don't really know
45:15 what I'm doing. Yeah. Please help me. Like, here's the problem I'm having and found that that had like thousands of people like flooding in giving answers. The cycle, the psychology tricks there
45:24 Catfish. Yeah. Beautiful.
45:28 It's hilarious. Um, well, tell us a little bit more about You know, we've talked a lot about the AI side, but what else are you guys working on, you know, between the companies that on the,
45:38 the technologies I know the AI piece is a very small sliver of, of all of the stuff that you guys do, especially when it's involving data, right? Yeah. So vibrations, a big, a big initiative
45:52 right now. A lot
45:56 of, a lot of companies outsource that. Yeah. We, when we can, when it makes sense, adopt the, the build versus buy approach and we did that with vibration
46:08 So we design those. Are you all using your own sensors? Are you buying those? We're buying. Yeah. Those the KCF ones? No. No. Okay. No. That is a company that does that. That is a company.
46:18 I've seen a vibration paper somewhere in heart or SBE or on the internet at some point on frack equipment. And I was like, Oh, that's interesting. Yeah Yeah, paying attention to the stuff. Yeah,
46:29 yeah, I mean, KCF, they're very.
46:33 prevalent in pressure pumping, for sure, but no,
46:35 we're
46:38 not. So they're a buy approach. Yeah. They install their equipment, and then they have
46:46 like full SaaS type. Right, yeah, they tell you what to do.
46:51 You don't wanna do that because you're the, oh yeah, basically. Right, right, so we, like I said, we take that buy approach, especially when it comes, or sorry, build approach, especially
47:01 when it comes to insights into our equipment. Yeah, that makes a lot of sense. So we designed the system, and we're kinda designing the workflows right now, and deploying those workflows around,
47:15 not just, hey, you have high
47:21 RMA or VRMS on this fluid end, but what does that mean? 'Cause no one knows what VRMS is not that they should, right? a different job, we should know what VR messes, but you have some high
47:37 forces on this fluid end. Here are the potential reasons, and then here's what you should do about it. You don't get that when you rent vibration equipment. You just get the, here's the vibration,
47:50 here's what we think you should do. And then the communication gap between a vendor, unless you really integrate that. I don't know your business. Right. I mean, some in a way, right? Yeah, I
48:02 mean, they have broader industry knowledge, but unless you really integrate that into your business, there's always a communication gap, be it like a physical one where they can't reach someone or
48:14 just the lingo with that company.
48:18 So all that's eliminated when you build and much more economical, kind of just to put a cherry on top, so to speak. So we got that going on, that's going pretty well. There's a lot of excitement
48:33 about that, especially from guys in the field. They are very into vibration data, believe it or not. And I get it. I get it. I was going to say if they understand it and it can make their life
48:47 easier so they don't have to do maintenance in the middle of a stage or, you know, whatever, that. Why not? Right. Right. I mean, you know, vibration data is a subject matter and a discipline
48:59 in and of itself and
49:02 you can get, you know, all your certifications to analyze vibration data. But what it represents for those guys is like a physical measurement rate of what's going on, not just pressure and
49:14 temperature and rate ratio. They're bombarded with, you know, across, across pad, you know, everything's got a pressure temperature and rate and RPM. And, you know, you can do some powerful
49:23 analyses with those types, those data points, but
49:28 vibration is not all the contents, right? Vibration takes it a step further. Um, and you know, you can get into the audio analysis of that, you know, depending on what sensor you get, right?
49:39 You can get audio from them. And, uh, you know, we've all, we've all put our ear up to a fluid and, you know, heard it. I mean, I know we even already asked for doing some cool stuff. Oh,
49:49 yeah. There's the shoot in the fluid levels and like getting like the frequencies back. And there's all kinds of cool stuff with acoustics that we, I feel like is distributed across the oil field,
50:01 but know what, like, there's not a central like acoustics or sound vibration conference or anything like that before the oil field, which is interesting, because there are so many different
50:12 applications for it, whether, I mean, we were doing pipeline acoustics, we're shooting pipelines for miles, right? Sound travels pretty fast, but you can also use it for fluid levels in a well,
50:23 or you can use it for vibration monitoring. Like there's so many different things there that's, it's a, but yeah, It's yet another dataset, so then you have. do something with it, which I think
50:32 that's, I'm not going to be really high frequency. Like if you're capturing that, I mean, to get back to the data. I don't like hundreds of Hertz or like how frequent I have no idea. Yeah, it's
50:41 thousands,
50:43 but the, the FFTs are done on the edge device. Yeah. So it, you know, we get the transformed data. Yeah. Um, and so we're not processing, you know, yeah, 20, 000 data points a second. You
50:57 mean, you don't want to put that in your cloud story. Yeah. But what if you need it? What if you need it? Yeah. Um, yeah, so if you can, you're not plotting it on a real-time dashboard. Yeah,
51:07 if you can, if you can derive something out of raw vibration data, you're someone special, you see color, you, you hear colors. Right. Yeah. Who's the dude that was on Joe Rogan? He's, he
51:19 was from, uh, he's actor. Oh, Terence Howard. Yeah.
51:25 That was interesting. Uh, yeah, his whole podcast podcast. No, that's
51:32 That's that's what have y'all have any interesting insights that y'all have been able to kind of clean from that you can talk about or
51:41 I don't think anything I can talk about
51:45 But you're seeing inside, but yeah, I mean are can you talk about the winds like the results of it or yeah? I mean, I think the I think the obvious one is condition based Monitoring in maintenance,
51:57 right?
51:59 Again, you can do some of that with pressure and temperature and right things like that But it doesn't really work until you have that yeah vibration measurement
52:10 and You know when we chose the the equipment that we did right the requirements where it integrates really well with everything else that we already have Yeah So because it does that like it fits into
52:22 a lot of our other workflows Yeah, really nicely in terms of like how we alert people and how they visualize made and so, you know when this turns red, stop,
52:35 and it's all just kind of a very natural fit to what we already have established. That's cool. No, I'm glad. It's so like the service side is we just have the worst moral, but I feel like from a
52:51 technology perspective because it's like we need technology because we always need more people or we're always trying to do more with less to squeeze another fraction of a penny out of the dollar
53:00 because we're always at least for the last what feels like decade. It feels that way, right? Service side has always been the operators always had the upper hand on the negotiation and pricing and
53:12 stuff. But then on top of that, it's like, okay, well, I have to improve my op-ex and my cost, my maintenance, because if I can't, then I can't make money. Yeah, but there has to be an hour
53:23 high, you have to spend the money to save the money, But then how do you justify spending the money
53:29 if you're trying to cut heads? It's a tricky, it is, and that's why, so we talked a little bit about data monetization
53:39 beforehand, and I kind of look at it. So there's a book, it's calledData's Everybody's Business.
53:45 Recommend you read it, if you haven't, you'd like to read. Yeah. And it's, I mean, I'm not doing it justice here, but there are effectively three ways to monetize data. You can improve with
53:57 data, you can wrap with data, and then you can actually sell data, which, you know, what everyone's mind goes towards when you say monetize your data, and that's actually selling actual data is
54:09 the most difficult thing to do.
54:13 But, you know, you can improve your business with data, and there are some kind of subsets of principles that go beyond like, you know, are you inspiring action and things like that? You can
54:24 wrap your current product with data, right? So as an all field services company. have a customer offering, but you can wrap it with data and insights, insights and things like that. Yeah, what
54:37 we had Liberty Frak on and it's like, right, I mean, they Frak Wells, just like, you know, Albert and Ernie Wells, but then it's like, is there some gravy you almost that you put on top of
54:46 that, that makes it. Right, and there's, I think even more, or just as much opportunity on the power generation side, you know, because evolution's only part of Buse's business, right?
54:55 There's just as much opportunity on the power generation side for those improving and wrapping initiatives, right? And then, of course, the selling part of it is very difficult to do. I mean,
54:56 some people build businesses around it, of course, but where we sit, it's probably, you know, probably the most difficult thing to do. Yeah, I agree. So kind of shift gears a little bit,
55:28 We'll be running up on time here a little bit, but one thing we'd like to talk about too, is like the journey, your personal journey, like into like data and stuff like that. So I mean, like,
55:37 how do you first get into data? Obviously, I mean, I think it looks like you had an engineering degree. So I mean, naturally you're playing around with data, but like how did you kind of start,
55:44 go from like engineer to kind of more data professional? Yeah, 'cause you've got an interesting background being working in the field, both onshore and offshore, it sounds like, and then back to
55:53 onshore. Yeah, yep. So maybe a little bit of a career history. Graduated college, Penn State in 2012,
56:03 and had a job with Chevron working offshore, amazing opportunity, right? Especially for
56:11 a new guy coming out of school, right? The first thing you do is you go out, go out offshore, right? And I had some internships, so I've been exposed to land rigs and land ops and things like
56:22 that. Gulf of Mexico is totally different. Yeah, well you mean the Gulf of America. Excuse me. Yeah, the goa So work there in a couple different capacities Until 2015 and hit that Layoff's cycle.
56:38 I have an ag background So I went to I kind of left the industry and thought thought I'd get out sure as everyone does right and that's siren calls me back Yeah, and100
56:51 oil Spent
56:54 a little over a year at an ad company testing harvesting equipment, which is a cool job I got to travel quite a bit and see some cool places, but ultimately wanted to come back Came back as a field
57:08 engineer for keen at the time and then her devolution was getting fired up in Northeast so put my application in and you know luckily lucky enough to land the job and uh was a field engineer which I
57:25 think the role was actually called data van operator, because they, I mean, we were expected to rebuild pumps and help with rig up and stuff like that. So they kind of considered it more of an EO
57:36 type position, but in any case, I was a field engineer.
57:41 And I started to notice as I
57:46 was kind of traveling around to different data vans and looking at our logging setups, like just kind of started to notice there were some inconsistencies in how our tags were never, all right? How
57:60 are each crew labels each field, each channel in the van differently? Yeah, imagine that, right? Why would they do that? Thank God. You know, just started noticing inconsistencies and things
58:10 like that. So just kind of took the initiative to fix it, right? At least to the degree with which I could. Sure and soon. Some people in the office took notice. Actually, the gentleman who is
58:26 my boss now reached out to me. He's actually been my manager since I moved into kind of the data role and has really mentored me to get to where I am. Yeah, very fortunate.
58:41 He took notice and he actually started all the data technology initiatives at the company and was kind of building his team and had remembered what I did in that regard and asked me to come on to his
58:55 team and jumped at the opportunity and worked really hard at it and just kind of progressed, I'm not going to say slowly because it was pretty rapid, but it's been, I mean, a wild ride to kind of
58:59 think back gradually and then all of
59:11 a sudden kind of thing Yeah, you know, eight years
59:16 in the field and, you know, overnight it just kind of kind of.
59:22 went vertical. That's cool, man.
59:27 I think that just kind of hammers home the point we were making earlier where it's like the SMEs are the ones that can really change things for companies, especially when you can empower them with
59:40 the right tools to do so. There's so many people out there at every business that are like, Hey, if I could solve this little thing that makes my life a pain in the ass, I would be able to spend
59:52 two more hours doing the things I like doing that are actually important. And it's just, Right. Why does VAN set up take me two and a half hours when it could take me 25 minutes type thing? Well,
1:00:04 how do I fix that? And it just so happened to be the right thing at the right time. Yeah, that's cool. I mean, if someone was any formal education in there around data or anything. Yeah, I have
1:00:16 some, you know, some certifications, data science and and things like that. And those are really just,
1:00:25 you know, as you step into a new industry, like you realize what you don't know, right? So what's the quickest way to become proficient, right? Sometimes it's certifications like that.
1:00:36 Sometimes it's just Google it and do
1:00:46 it. Yeah, no, I'm really on both sides. I mean, a lot of the more has been like, almost like self-taught just and just prove value. You know, they're doing it. But I think if you find a lot
1:00:49 of it that is then as like vetting like those certifications, some of them are total, right, dog shit, they're not worth anything. About some of them are, you're truly hold weight, you know,
1:00:58 when industry is going to understand those too. Yeah, yes, it's a good way to familiarize yourself at minimum, right? Even if whoever is hiring doesn't give a shit about the cert, right? Like
1:01:08 if you're, you know what SQL is, but you've never been exposed to, you know, if you know what a database is, but you've never written SQL or been exposed to it, Like, that's a good way to get
1:01:17 some kind of foundational understanding of it, right? You could also go on YouTube and watch some of these things now and may or may not have better. It was just, yeah. Even like, I mean, Udemy,
1:01:28 I wouldn't post my Udemy certificates, actually, I don't think if I ever truly completed a Udemy course.
1:01:35 But, like, but I mean, like, I think it's great if you just want to learn real quick about something, like, you know, I think I used one to learn SQL back in the day, you are up to upskill on
1:01:43 SQL, like, it's something just like, I need the content and I've started format Yeah, I mean, I think what you get out of those certifications or any training is really dependent on what you're
1:01:55 immersed in, right? Like, if I just, like, I'm curious about what SQL is, like, I'm just going to go Google it. Yeah. But if I need it, if I need it because my job success depends on it,
1:02:08 like, not only am I going to, like, learn it as quickly as possible, I'm also going to put it into practice as often as I can. Yeah. You know, like, if I need some data and I'm trying to learn
1:02:20 SQL, I'm not gonna go to Power BI, right? I'm gonna go write a query to see if I can do it and at least reinforce the fact that I learned something. I think that's some of the best advice is like,
1:02:33 'cause I'm the worst student as far as a lot of that shit goes where it's like, I've started so many Python classes online, or not just Udemy, but like the guided ones where you can do it by
1:02:45 yourself or the ones that are gamified and all the stuff and it's like, but I got to the point where it's like, I understand what classes are, I understand what functions are, and I know what we,
1:02:54 like I know the basic foundational stuff, I just need to solve this problem. And so it's like understanding a foundation but is good, but in the real world, you're trying to solve problems. And
1:03:06 so the easiest way to solve those problems is to just go try and solve them, right? Like to figure out how to do it in Python or SQL or whatever it may be is just to go try and do it. And when it
1:03:16 matters to you, and when you understand the context, sway better than figure out, how many widgets did I sell? Right, no, that's the biggest thing, right? It's the same thing. I got my
1:03:23 master's while I was working after being out of school for however many seven years. And it was a completely different educational experience because it all had context, and I could immediately use
1:03:35 or understand where I could use everything I was learning versus when you're in undergrad, and you don't know shit about anything, and you have no concept of what it's like to work in the real world
1:03:44 And you're like, why do I need to know how to do this differential equation that I still haven't used, by the way, Professor. But
1:03:53 it's just very different, right? And so that's where I think you truly learn the thing that you're trying to do is by doing it, right? Like you can sit in a classroom so much, but until you're,
1:04:04 'cause then you have like the boundary conditions and all the outliers and all the, you know, well, it has to work in this environment, it has to be compliant with that. Like that's very
1:04:12 different than the simple example they give you in whatever class and. Yeah. And then, and then every once in a while, it becomes really rewarding where there was like a business. Yeah. And it's
1:04:22 just happened recently, like there was a business need
1:04:26 for an application.
1:04:30 And, you know, everyone's tied up all the time. And like, I oftentimes feel really bad about going to my developers and being like, Hey, can we have a sprint schedule?
1:04:41 Do tomorrow. Yeah. Yeah. And it ends tomorrow. But
1:04:47 seeing, you know,
1:04:49 in the position I'm in, I don't get to do, I don't get the code like a ton. So I just, you know, I took it on and it's like, it's helpful for the business. You know, it reinforced, you know,
1:04:59 this learning path I took was the right one. Yeah. You know, all those kinds of things that kind of all come together every once in a while. So it's all very rewarding. Yeah. No, I think that's
1:05:09 the underlying kind of clip of advice there is, you know, just be useful. Like, be as useful. If there's a problem within the company that no one else appears to be able to solve or is focusing
1:05:20 on, but you know that it's going to impact, figure it out, like, whether that's writing code or, you know, something else, it doesn't matter the, but that's how you, yeah, get sticky in a
1:05:31 company. Yeah, yep, yep. Solve people's problems. Solve the right people's problems, so. Absolutely.
1:05:37 I'll get into the speed of that. Yeah, this is, this hour flew by.
1:05:41 But it's been a great conversation with you. Yeah, I agree So our speed round is just random questions that we'll ask you about all kinds of stuff and just short answers to get to the movie a little
1:05:53 bit more.
1:05:55 What is your preferred cloud? Just you personally.
1:06:01 Still Azure. Azure, yeah.
1:06:04 They're all good. They're all good to agree with. Yeah. That's, they've all got, they're. I imagine most people answer people. Answer people, right? AWS is normally. Really? I think most
1:06:16 like if you're a software developer for AWS, most of the time. Well, I mean, a lot of more of the enterprise type folks like Azure. But again, you can achieve most of what you need to do on both.
1:06:27 Yeah, 'cause all those free Azure credits, Bobby.
1:06:32 All right, so AssemblyX went about a 30 year year in Houston or so. Yep. So what's your favorite food in Houston and then what's your favorite food back home? That's a good question. Favorite
1:06:43 food in Houston? Simple answer, taste of Texas, steakhouse. That's a good one, it's a good one. It's the right mix of quality and price and all that. If you wanna go around the corner, there's
1:06:55 a really good Uruguay and steakhouse over there. Salam Pimienta, probably one of my favorite restaurants in Houston. Okay. And order from the steak board. Okay. Bring your checkbook, bring
1:07:07 a client. That's what you mean. Yeah, yeah, but it's very good. But now taste of Texas, hard to beat Favorite food at home, I love smoke and brisket. Okay, yeah, so that's the thing I'm PA.
1:07:18 Or just like, that's what you do at home. It's just, you know, middle-aged man. That's kind of like - Yeah, of course. Yeah, you smoke weed. 100, yeah, absolutely. Look at World War II
1:07:25 stuff. What's your favorite? Because you're in Pittsburgh or you in Stake College? Stake College. Okay. Well, dang, I thought you were in Pittsburgh. What is your go to, or just
1:07:38 what's a good, they don't have to be Python, but what's a good library that you've used that you think other people might be, at least in the kind of data space or energy space that you might be
1:07:49 handy? Yeah, it's a hard one, but everyone on the spot with that question. So I'm gonna answer via Python though, Flask. My name is not necessarily the library, as much as is a framework, but
1:08:01 - Yeah, I mean, that's a good - I think it's very powerful for developing web apps quickly. Yeah. You mess with a fast API at all, or no? I haven't, I think it's got a Flask on steroids.
1:08:14 It's supposed to be faster and type safe, whatever, but no, I mean, I've used Flasp before too and just to deploy things like APIs quickly and actually when you're doing ML or AI or, you know,
1:08:24 something like that where you're serving models, you know, it's, it's just always comes back, Python's that Swiss army knife and all this can just glue it all together. Yeah. Yep.
1:08:39 Favorite database,
1:08:43 well,
1:08:45 I don't know that I can give you a good answer because of only use that or SQL databases. Okay. So SQL Server kind of, and I guess it has its own SQL flavor too, I guess. Right. Yeah, your
1:08:56 delta tables and things like that. Yeah. We used quite a bit of MongoDB though. Okay. They're pretty good. Yeah How have you enjoyed MongoDB, because I get mixed reviews on it, it's really
1:09:06 flexible, but then like it can. So yes and no. Yeah. Yeah. Yeah. I like it because of its flexibility, but you got to make sure you need it. Yeah. Right.
1:09:18 And you don't need relational data. Yeah. Yeah. Always use the tool that you actually need, not the sexy shiny one, right?
1:09:24 What? God, I just had a good one and I lost it
1:09:31 Usually ask a board game or oh, yeah, that's what it was gonna be. I was gonna ask you either book favorite book or board game Bored or a video game. We can go don't play video games, but so
1:09:44 Favorite book I might actually go back to that data is everybody's business I'm gonna add that to my list because it sounds like a good one. Yeah written by a couple MIT Staffers, so yeah, pretty
1:09:57 legit. I wouldn't consider it an easy read I mean, it's not over. It's not overly technical, but it's not all I see is my wife looking at me when I showed her Some of the stuff I was listening to
1:10:08 on our drive to the spring break and her being like what is wrong?
1:10:16 Few hours to I mean, yeah, what nine hours to orange beach from here in long drive, but it's one of the things where it's like she's or I'm Unfiction almost. And she is almost exclusively fiction
1:10:28 and we both think the other one is crazy
1:10:32 Yeah, I can't fathom sitting like dedicating time to reading if I'm not going to learn something Yeah,
1:10:41 but my wife see exactly Yeah, last one It's a favorite vacation destination. Oh, I
1:10:51 have a new one Hawaii. Yeah. Yeah, we just went a couple months ago while last month nice and which island Maui Maui. Yeah Yeah, cast Jan on all those hotel points. Nice. I get so yeah, it was
1:10:59 pretty good Yeah, I
1:11:07 think I'll end up there in the next couple years. My brother's getting moved with the Coast Guard. Oh, I oh cool And June's got a good excuse. Yeah. Yeah. Yeah, I know that's on our shortlist,
1:11:19 but It's it's the whole it's like my wife wanted to go to you know Tahiti and stuff our honeymoon. I was like, we don't have a month. Like,
1:11:29 even Hawaii is still a hell of a flight. Like, that's, yeah, you got to make it worth it. The travel, right. I'm not going to go for a four day trip. It's got to be a decent amount of time.
1:11:40 Well, cool, man. Where can people, you know, find your reach out to you if they have any questions or yeah, LinkedIn is probably probably the best place. I usually keep up with messages and
1:11:52 stuff on there. I'm going to go tag you on collides so that we'll on a collide post. So we'll get you more engaged over there too. Yeah, force me to force me into it. Yeah. No I, mean, that's
1:12:03 the point, right? There's people that we get all kinds of random questions from operations to technology type stuff on collide all the time. And so it's, yeah, I was the idea behind the podcast.
1:12:14 It's the idea behind the platform just to distribute information. Yeah, I'm going to tag your ass when you got any answer.
1:12:22 Well, cool, man. Make sure everyone likes and Hit the like and subscribe button. We appreciate you guys. I have to remember to do all the social influencer stuff, even though I don't consider us
1:12:33 either of those things. But we appreciate it. We will, like I said, we'll be back soon and we'll promise to be a little bit more consistent. The remainder of the year, appreciate it. Thanks
1:12:43 again, man.
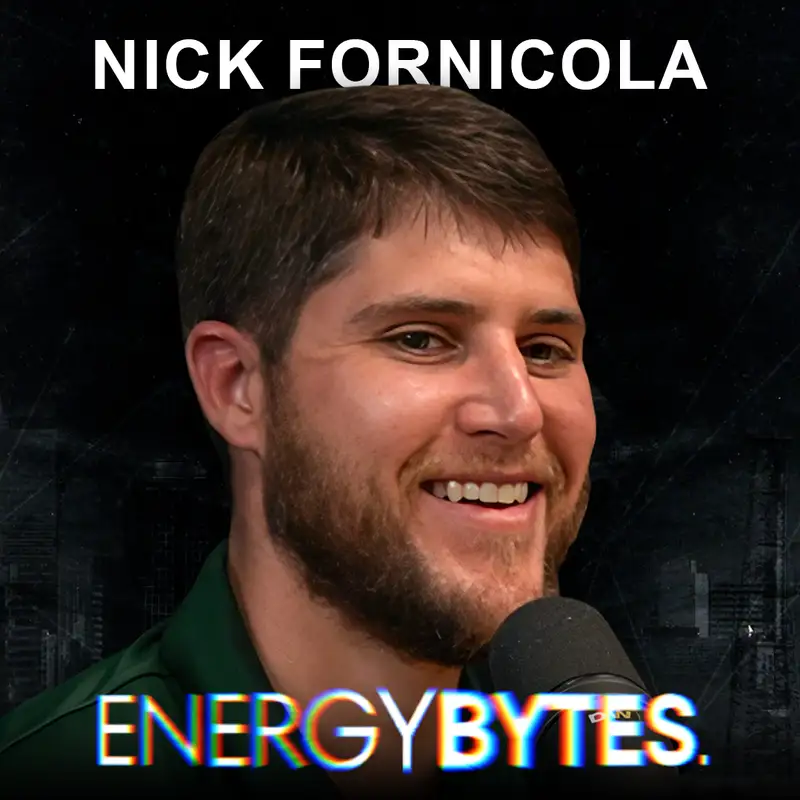