EP 57: EZ Ops and Detechtion
0:00 Welcome back to another another fun episode of energy bites. We've got we've got Bobby back today. He's done dealing with the the merger and acquisition. Well, I've never done dealing with it,
0:13 but you're through the the hard part, so to speak. So happy you're you're back today. Nice to see you, man. I'm here, same here. Enjoying the beautiful, beautiful weather we're having today
0:26 Finally, I won't sweat out here. Well, I'll sweat, but not that bad. As I say, it's still a little warm. And then we've got Rashida and Brandon from detection and easy apps with us. Thanks for
0:39 thanks for joining us today, guys. Thank you. Thank you
0:44 for having us. It's really good to be here, excited. I was good to have some Canadians on fun. Yeah, we were just talking about it. I was like, they've already got snow and it's 85 degrees here,
0:53 but little, I told them, you know, in Houston, you never know if you're going to need a coat or sandals for Halloween.
1:02 Or actually this year, it looks like we're going to get rain, which we haven't had in like two and a half months. And it's going to pick the one day all the kids want to rain, but it'll be fun.
1:12 Anyway, why don't you all introduce yourselves and kind of tell us, you know, just high level, what you guys do.
1:22 You want to go ahead, Brandon?
1:25 OK. Oh, OK. From my internet, it's OK
1:28 Yeah, Brandon Ambrose, founder and CEO of EasyOps. And then EasyOps just recently was acquired by detection. So strategic advisor to detection.
1:41 Yeah. Very cool.
1:44 Awesome, yeah. So typical for Canadians, right? Fighting over who can go first. Me into flight.
1:52 Hi, I'm Ruchita Rosario. I'm originally from India, moved to Canada seven years ago and four of those have spent with easy ops now a detection company and I started as a lead data scientist and I'm
2:05 currently the data science supervisor. So anything and everything that we collect from the field data capture goes to my team and then we sit together and talk about gaps in the industry and the
2:19 products that we can put forward to meet those and mitigate those gaps
2:26 Awesome. I'm not excited to have you guys here. Yeah, again, I was saying before when Tim was with us that I was familiar with detection, but I think now seeing as you guys were acquired as part
2:37 of that, I don't think I was actually tuned in to this side of the business. So I'm definitely interested to hear what what easy ops is and just kind of how it started and then we can probably get
2:48 into the technical bits of it, but I don't know, Brandon or Rashida wants to dive into that, but.
2:56 Yeah. Yeah, for sure.
2:60 Yeah, EZOps is an augmented intelligence platform for blue color workers. So basically consolidating data overlaying with machine intelligence and then interfacing with blue color workers so they
3:09 can increase their performance when they're out in the field. Yeah, I level that's what EZOps is.
3:19 How did you come up with the,
3:22 you know, the idea, the company, the product, so to speak?
3:27 Yeah. So my first business was a company called Reliance Production Optimization. So it's an asset management company up here in Western Canada, where boots on the ground managing new only gas
3:40 assets And just kind of had a unique eye for understanding the lifting cost, understanding the production, and also pulling the wrenches, so just having that business sense on the front line. And
3:54 I was looking to scale that services business, but it was really difficult to transplant my brain into the team and scale that. And that's when the idea of kind of easy ups, you know, we had
4:06 everything all digitized, get the right information at the right time for other right people Yeah, that's kind of where it came from was to internally for my services business. but then realized I
4:17 could have more of an impact on the oil and gas space, licensing software,
4:23 and post it. So what was the main challenges and problems you were trying to overcome, or that you faced in the field? I can absolutely relate with the field data capture side of things, 'cause
4:33 that's, I think, the vein of most people's existence the of services any side on work that
4:40 of the business.
4:42 Yeah, it was super cool once we got, you know, Ruchi on the team, and we were able to actually start quantifying some of these pain points that we were physically experiencing. But field teams,
4:53 they're in control of just a ton of different variables, you know, whether it's, do I adjust chemical rates, what wells are down, which, you know, is, which liquid production's down? Do I
5:01 need to inspect things, send pigs, preventative maintenance? Kind of there's a barrage of different things in a large geographic area. So the challenge was,
5:12 first of all, for field people understanding. how they should prioritize things and then and then acting on that yeah I can dive into more
5:29 No, I think that's a good starting point because I think that's, you know, the Bobbi and I both have worked quite a bit on the data side, you know, ingesting field data or data from the field or
5:41 data from sensors in the field and all of that stuff. And,
5:46 you know, people think it's as simple as throwing a gauge or a sensor out there and then just pushing the data, but it's definitely not, not nearly that easy It's not nearly that easy to even just
5:56 get by and to try and go do those projects. But even if you do have the raw data, the big thing with the field is you don't have any of the context, right? And so I think what you guys do, you
6:07 know, the efforts that people have been putting around contextualizing field data have come up because people finally realize that, hey, we've got this data that we don't really know what to do
6:18 with it or how to use it Or if we have this other piece of data in conjunction with it, it would be a lot more useful. Yeah.
6:27 to that point is everyone wants to run a green operation now, all of the producers and I feel that it's 2024, everyone has also realized the power of digitization and data and all of that owners is
6:40 coming on the operators. It's just so much data points in such disparate places and there are two, eight digitized records. So now they move from pen paper excel to into software like us, field
6:54 data capture platform like us. And then there is also that component of now that you're putting in data in the system, you also at the start of your day have to make sense of it, let alone the
7:05 extreme conditions that you're working in and the fact that you're overworked. And also meanwhile, make sure that you run a cost efficient operation and OAS, it should also be green. There is just
7:17 so many moving parts that eventually boil down to a frontline workers decision and what best performance is. Yeah, one of them, the real tricky part is making sure that however you capture that
7:31 stuff and whatever that workflow is, isn't interrupting or causing the guys in the field who actually use it and who are actually inputting the information more of a headache than it's worth without
7:42 using it. And so I think that, I mean, there's a ton of companies that have come and gone, but I think that's one of the big things that kind of shows the test of time with these companies is the
7:53 ones that get that right are to stay around and have longevity to them. And so I'm very curious how y'all kind of approach that because
8:04 putting an app out there and hoping that people use it is very different than having every single pumper field tech, whoever using the same service, the same systems, the same processes every
8:16 single time and doing it consistently and all the time and not just saying, well, that's cool, It takes five extra minutes, so I'm not going to do it because I don't have five extra minutes.
8:28 So I'm curious how y'all approached that, how y'all were able to kind of,
8:32 you know, achieve that. Yeah, kind of some fun numbers. So there's about 55,
8:41 000 operators or pumpers in North America. And on average, they're in control of around85 million a year. So like7 million of that is the commodity that they're pulling out of the ground about a
8:52 million and a half of that is all effects that they're spending on behalf of the producer, like in some form. So they're in control of a ton of money, but a lot of them are like me, you know,
9:03 maybe they have a high school education and really we're putting the onus on them to be absorbing that data, then synthesizing that data and then taking action on that data. So there's a lot of
9:13 opportunity.
9:16 I don't know, do my internet cut out? There
9:20 No, you're from the, like I said. It'll buffer. So even if it looks like it's pixelated on our end or anything like that, the finished part would be fine. No worries. Beauty, yeah. Yeah, so
9:32 yeah, complex. As soon as Ruchi started looking into this and we started looking at that data, every morning a typical operator could have like almost 600 million different scenarios of actions
9:42 that they could be doing. And the, an operator, I think as they grow over time, they get an algorithm that they develop in their head as they get more and more experienced on how they should be
9:54 taking action. So what we needed to do is day one, be able to have people making the best decisions and taking the best action.
10:04 One thing that we found was really interesting as well. When you interview clients and you ask the question, you know, what does high performance look like to you, you get a barrage of different
10:14 answers. So it's like, people don't even know what good is. And that's
10:20 what we found pretty fascinating. Yeah, so to us, it's high performance for everyone in a producing company is maximum production, minimum cost, well-maintaining safety and compliance. So
10:30 everyone takes these like microactions to try to drive that. You know, they can be weighted differently based on the producer, but you know, that's what they're striving for. So we're just
10:42 helping everyone take the best actions. Right. I think another aspect also is to be able to represent every aspect of their job into a single piece is not having to go to a different place to finish
10:59 your inspection forms and then a different platform to maintain your begin schedules. And then there is a different platform to look at how your production wells are doing. And then there's just so
11:10 much disparate data there. And that is one of the key points that we try to focus on is kind of represent every aspect of an operator's life our platform is, you truly cannot tell someone that this
11:23 is the planet you should follow to be your best without knowing every aspect of their job. And that is something that we are truly always striving towards is how to incorporate these external data
11:34 points, be it weather or distance data or road bands, all of these complex ideas into this box that churns out an easy and palatable list because it's hard already, right? Yeah, tab overload,
11:50 right? It's like, that's, I think that's such a overlooked kind of, won't call it a piece of innovation, but that's an overload kind of area where people are just like, hey, if both of these
12:03 things lived in the same platform and talk to each other, it would be a lot better. And so there's been a lot of companies, I think that have become really successful because they're in users at
12:16 the start Okay, hey, I have to go click through four different tabs on my laptop to finish this one job. And if I could put all of those in one and half the time they all need the same data that
12:29 I'm repeating the same entry for, which is insane. Also leads to human error across all of this stuff, right? And so all those things just compound.
12:41 Yeah, I mean, so I think Brandon said probably my favorite AI earlier, which is augmented intelligence.
12:49 'Cause I think it's one of those like, we always hear like, you have to do more, people want to do more with less, but I think people are scared of, yeah, artificial intelligence like that.
12:58 It's going to take my job, it's going to replace me. But doing more with less isn't necessarily replacing someone. It could be just taking your existing people and making them twice as effective or
13:07 three times as effective or whatever the multiplier is. And so you're still getting more for the same, which is more with less in that
13:17 way. As far as the platform goes, is this like where you guys, the one platform to capture all or are you kind of like a hub that you can pull from like other different systems into easy ops and
13:31 then kind of, you know, make it like a single pane of glass for them to look at? I'm just kind of curious, I mean, maybe it's a little bit of both, but.
13:40 Yeah, and just to kind of even finish up one John's question there. So I think what differentiates us and why we're, you know, it went through the test of time and we're still here. I started
13:52 Reliance, which is my asset management company in 2007. And then in 2015, that's when we started building easy ups. And it was like, you know, it was in the user's hands from 2015 to
14:04 2020. And to your point, you know, getting rid of those tabs, getting that feedback. How do we really augment and how do we help people on the front lines? and then between 2020 and 2024 now I
14:17 think it's 27 of Canadian oil and gas production is utilizing our platform right now. So it's like, we've had kind of massive growth and that was because we're just, we really understand the
14:28 importance of helping people on the front line. And then that's how you get that adoption. And then you get the field kind of pulling it off the shelves instead of head office kind of pushing it
14:39 down.
14:41 But yeah, that augmented piece is really important I kind of, when I'm chatting with the team and I guess when I was leading easy ups, I was talking about, you know, in 10 years, robots are
14:52 gonna be doing a ton, especially with some of these, you know, cool stuff that Elon's doing now with Tesla. Well, between now and then we need to get people performing at their best. We need to
15:02 augment them. You know, back in the day when I started, it was 10 to 15 wells you were managing. Now you're managing 100 to 150. And it's an exponential problem on,
15:14 Absorbing that data, synthesizing that data and then taking the right action. So we're kind of like, the technology is lagging, which should be the problem exists. People can't perform at their
15:24 best. And now, we've created that technology to
15:29 feel that impossible void that they're in or all that problem.
15:35 Right here. And to Bobby's question, we, a little bit of both, would definitely be the answer We do try to capture most of their roles and responsibilities like
15:47 inspections or maintaining those pick schedules, machine schedules, taking tank readings, pump readings, all of that. But then on top of that, we also then overly external data. Like we have so
15:59 many integrations because every producer manages their production information on a different platform. So the idea there truly is to have that one stop shop where an operator in the morning logs into
16:11 the product. And these are the. 10 key things that you need to do, which are agreed upon, and that clarity flows through from upper management to you on what best performance is. And kind of
16:24 creating that alignment. I don't want to, Brandon and I actually worked on a survey a couple of years ago, where we looked at the
16:33 total revenue, I think, or a success metric like that, and correlated it with a survey that we took on how aligned the frontline workers with the upper management. And we actually found out the
16:45 more the answers were aligned, the better the company was doing. And that's essentially a gap that we recognized is, I think people are telling the frontline workers to work at their best, but I
16:57 don't think anyone has defined what best means. Right.
17:04 And they don't know what the target is. It's hard to work towards it, right? Yeah, absolutely And you know, one thing, the. I think the reason why we've been successful is because we started
17:15 building this back in 2015. 'Cause if you're gonna prioritize someone's life, you need to understand every aspect of their life. And that means that, yeah, we're gonna absorb whatever we can get
17:25 the data, but there's a lot of data that's not digitized. So that's where we needed kind of this broad platform to be able to absorb that currently not digitized data. All of those analog inputs
17:36 that are need to come in.
17:39 And so that's, you know, it's been a journey and all of those different modules to have the breadth needs to interact really, really well with the user. So it was certainly a front-end loaded
17:51 project to get an interface to start digitizing the data that we could then start optimizing.
18:02 So, I know you've got some stuff, obviously, that helps with the day-to-day and all that, but then like, how does capturing all that, does it improve, say, when they want to do well reviews or
18:13 stuff for the operators where they want to see how they perform over the last quarter and all that, like, that will make it easier for them to kind of have those and kind of have that idea of any
18:23 reporting, I guess, side to that, where they can check the last quarter year to date, all that kind of stuff I think the biggest benefit there, like, we don't kind of, you know, we don't want
18:35 to be the best kind of analytics of, uh, of well performance, but we want to be able to then, or what we do, then now we have all of those micro actions that have happened to get that end result.
18:46 Um, so, um, that's kind of where our, our focus is, is, is on the front line. It's with the guys utilizing the tool and then that can then be leveraged Um, you know, why did the well
18:58 performed better or worse? Now you have all that. data to capture daily and so on. So then you have that context.
19:09 I didn't know how many could say this is why Jim is well to performing better than Joe's, you know, like two routes over, you know, and then like maybe I guess that's where they can learn from
19:19 each other and say, all right, you're doing something different with chemicals or water, you know, we are improving LOE
19:25 or whatever
19:29 and it is day to day as well on all the aspects that helps in having a platform that is as wide, but it is also futuristic in the sense that all the readings that the operators are collecting in our
19:42 platform. We also then provide futuristic recommendations on, say when they should do chemical hallings on based on all the reads that they have provided that. These are the 10 tanks on this field
19:55 and they are consuming at these many liters per day.
19:58 And then we recommend the package that needs to go out in say 15 days from now based on the data that they have provided. So it's also day to day, but future looking as well.
20:13 Okay, great. What are some of the, what are some of the, well, I've got two questions. The first one, what, because there's a lot of, there's a lot of people in the field, in the oil field,
20:24 right? And there's a lot of low hanging fruit or just operation, things that could be digitized or someone has an idea for an app that, you know, is targeted at field personnel. What is yours
20:36 advice to, you know, other entrepreneurs, other people that are out there building software that has that field element to it? And what's your advice around, you know, how to do that properly?
20:47 Because I think we've all seen companies that have a really shiny, cool looking thing, but then once it actually gets deployed, it never gets adopted. You know, it's deployed from the top down
20:57 normally. from management because they're trying to do something. The field folks typically don't have any idea or maybe not even any input on any of that stuff. And then they're just kind of left
21:08 with it. What are some of the lessons learned that you guys have from building field facing specifically?
21:18 Yeah, well, I think you nailed it. It's the adoption on the front end. So if you're not helping the person that needs to use it every day, they're gonna throw it in the bush. And you won't get
21:30 any feedback. You'll just get, you won't even get constructive feedback to be able to tweak. Like you need to be going in with the solid intentions. Like I'm here to help. I understand that
21:40 you're put in an impossible situation and we wanna make your life better. And as a byproduct, we're gonna help your boss and we're gonna help your company. First is how do we make you look the best
21:51 way possible? And so just kind of simply that direction. kind of found worked. You said simply, but I think that's, that is just an under-appreciated thing. What you just said is if you can make
22:07 the end user, a champion, look better, perform better, make their life easier, like you have to think of the root user, even if management wants whatever algorithm or thing in the background.
22:17 If the root user isn't improving their, it's not shaving time off of their stuff, but not getting them home earlier to their kids, they're probably not gonna use it Like if it, especially if it is
22:27 adding to their existing workflow or taking time away from what they're already doing, that's where apps go to die, right? So I completely agree with you. When we were building our, go ahead,
22:32 Randy. Go ahead, go ahead, Richie. I was just going to say that when we were building our not store on what we want to
22:47 do or where we want this product to take,
22:52 Bellows and one was monetary savings, another one was time savings and the third and key pillar was peace of mind is integrating this software into the system, how much peace of mind is created by
23:06 making sure that you have logged everything properly and what you do. And when there is that turnover day, does micro shift have all the information required to do their job successfully So peace of
23:18 mind has always been such a key pillar into kind of trying to gamify these product changes. Absolutely. And just to build that
23:31 from a handoff standpoint, from one pumper to the next, you know, like when they're working shifts, just keeping some consistency all the way through is super important as well
23:42 One thing that
23:48 I've found in the field is that there's a lot of there's Because everything's so dispersed across large geographic areas, there's kind of some pumpers or operators that pull all the weight. And then
23:60 there's some guys that just kind of they just fly on the next shift. And you know, so it was really good for one shift. It kind of carries the next shift. And so to helping the frontline person,
24:12 this creates that transparency of, hey, this one's really doing really, really well. And this person is not really doing that well So you'll find that the good operators really want it because it
24:23 then shows that they're actually pulling all the weight for the team. And then whereas the, maybe the not quite as good, don't really want it. And then it kind of helps for even hiring and firing
24:35 and stuff like that to let's less, not the strong ones.
24:45 So, if we take us a little bit different direction, because I like to get into the weeds a little bit on the technical side, so when you started this in 2015, Brandon, was this mobile first or
24:55 were you, was this a web app first that, you know, and then just kind of like cloud on prem, just kind of some of those design decisions, just kind of curious there. Yeah, we needed to have
25:09 offline capabilities, so we ended up building a progressive web app kind of for early on so that we could be iterating after end. And yeah, and then I think initially we were just using servers,
25:22 we had to migrate to the cloud. I think we did that in like 20, 2018 or something like that, but yeah, but what was the decision point there? Where did you run up on that? You decided to want to
25:35 move to the cloud because like we obviously cloud versus on prem is a never ending debate
25:41 Yeah, it was just. It's just the future scalability of multi-tenanted environments that we just needed to be on the cloud.
25:56 Nice. Yeah, so for people who don't know what a progressive web app is, but I don't think we've had that come up yet. Can you explain or I mean, I can get into a little bit, but yeah, feel free
26:07 to jump in. I am not the tech guy early on. I don't know if I heard the echo people, but yeah.
26:15 Yeah, like a progressive web app. And again, I'll probably butcher it if someone actually really knows what they're talking about. I heard me talking about it, essentially it's like, I think
26:22 people probably have seen it because I think Starbucks had it at 12, but it's like, basically, you can go to the website and it's mobile. It's a web app. But then when you, I think they're doing
26:33 some buttons and you can actually kind of save it as an app like on your computer or your phone, right? It's like it would look and feel like an app, but it's actually running through the browser
26:44 kind of in some way. But again, to your point that it still has some of that similar functionality where it can the offline capability and Thank you. Yeah, it's kind of an in-between thing, but I
26:54 think you can write a web app, but it works as a mobile app almost, so. Yeah, that's right. And then you can publish, we can iterate way faster. You're all, we can't wait for Apple or.
27:07 It's, I'm glad you pointed that out, 'cause people don't, it's something that you don't know what you don't know. And as someone who we've collived, we've had out as an app, I think, for almost
27:18 a year now And yeah, it's like, you can have everything buttoned up from a technical perspective, and then you submit it to the app store, and then Apple says, No, it is missingthis one very
27:29 ambiguous thing. And
27:33 you're like, Well, I don't even know. And it's all automated now, which I've found. You get, they have some kind of bot that goes and does some kind of random tests on the platform.
27:44 Automatically, we saw it through our traffic, And then they just sent you an automated. message that says, you need to add this or that. But it's just like the most ambiguous and big thing in the
27:56 world. I waited like a week one time, had to get on the phone with someone in Cupertino to talk through. I don't even remember what it was, but you know, we had to have a specific flagging
28:08 feature for users or something like that. And it's just like, you really realize how much control Microsoft and Apple have of the digital space on the app side when you have to go, everything has
28:19 to go through a stupid app store. But I also want to point out everybody who thinks Bobby did a terrible job of explaining that. Please go tell him about it in the comments because I know he would
28:29 love to read those later, so. I would probably learn something, so that's fine. Yeah, educate us, please.
28:38 So what was, what did that original stack look like? Did you have any kind of, kind of what were your decisions going through about what front end to use, which database, back in and all that fun
28:49 stuff. Yeah, I guess, yeah, I can tell you, it's just hiring good people. So our chief product officer was also our CTO at the time. So he was just, you just promised me, this is what we need
29:02 to do and this is gonna work well. So I just trust him. So I wouldn't even have you get an answer for you. Unless, Ruchi, you wanna jump in on some of the early day once, but it's all day right
29:16 now. I am not sure if I will be,
29:19 if I will justify the devs and I will have a hard time later in our meetings, we do some PHP, some React, a lot of Spring Boot, Java, like just depending on what kind of Solver we want to be
29:36 using and then any language that supports that. Yeah, we have an extensive team now. that kind of does those researchers. And we're also in the middle of moving and transitioning into a more
29:49 stable code base. Now with our recent acquisition, we definitely have more resources now to kind of look at the bigger picture and see what is the best part moving forward for these mobility
30:02 solutions.
30:05 Awesome. Yeah, so it sounds like almost, if you have all these different kinds of things going on, potentially it might be like more microservices, things that we're using the best tool for the
30:14 job on certain parts of the app. But again, I don't wanna push you guys too hard, if you're not the most technical people on the team, other routines on your data scientists. So let's get into
30:26 that maybe. What does that look like being a data scientist versus a developer at a company like that? Are you helping
30:33 prototype certain algorithms and then they're productionizing them within the platform or are you kinda curious how that works? No, yeah, that would be accurate. I do a lot of data visualization
30:45 and analysis into Sigma and Power BI. And through Sigma, I have snowflake connectivity. So if I need the quickest way of kind of querying the data and seeing how it is spread out and doing some
30:57 early analysis, then I use that. Generally, the way the workflow works is that a couple of the product team gets together and we kind of hatch out a pseudo-code that is done through testing I
31:10 generally test on a Jupyter Notebook in Python and the intention there truly is not scalability. It is to see if we kind of come with an algorithm, decide the way it's the heuristics that should go
31:24 into it and then based on a fixed set of input, we generate an output within go back to some of our champion operators who help us look at that data and verify almost like a reinforcement learning
31:36 model, but a manual one. And then we verify if you need to adjust those weights or adjust some conditions on there. And once that cycle is solidified, then we create a G-rot ticket that goes off
31:48 to the dev team. And then there is those technical decisions on what is the best way of implementing these. Okay, so you get to kind of recite, you know, that's pretty cool to talk about that
31:59 lifecycle of how you are, you know, getting that information from the field and like utilizing the data that you already have to amplify the platform further. Correct, yeah, no. Especially
32:12 because so much of this is RD and gamifying every action, how does it affect our KPIs? It's a lot of research and a lot of subjective information then which then translates into a line of code. So
32:27 the only pieces of brainstorming is done in Python super scrappy format. Let's get to the answer first and see if we're happy with it and then it goes through. though, how will we now make it
32:39 scalable for the clients? I think, okay, go ahead, John. No, I was just going to say I appreciate the shit out of out of people that people, especially with new development, new products, new
32:53 stuff, they, you had, I included, you can get caught up in like over analyzing how, how to perfectly architect it or all this stuff ahead of time. But some of the best, like I'm, Colin pushes
33:08 us and I need that to, to do stuff faster, to go and prototype, to go and test. Like it doesn't have to be perfect. It doesn't have to be 99 accurate. Just go and go into collab, go into a
33:19 Jupyter Notebook. It doesn't matter and start testing. And, and, but you'll get to a point where taking it from that to production, like you can iterate so much faster in those notebooks or in
33:30 these small scale things that you can come up with so much more so quickly than if you were going to sit and have like you know, I'm sitting at a giant conference table behind me. I was going to
33:39 have this whole conference table full of all of our devs and all of our architects and all of our data science folks. And then you're going to architect it on the whiteboard. It's like, even if you
33:46 architect it out, you don't know if all of those libraries work together. Or if there's an update to that library that makes it in combat, like there's all these things that would pop up once you
33:56 started to productionize it But if you, just by doing the prototypes and stuff, I'm very bullish on just that concept of prototype quick and fast and dirty, and it doesn't have to be, the end
34:10 result is going to be different, and that's okay. You might have to redo it multiple times. That's going to happen. But with the speed at which you can do stuff with these basic tools, I think
34:21 it's overlooked a lot Our senior product manager now, who is our CTO, then Nick always says, fail fast, learn first, and that has constantly been our strategy
34:34 Yeah.
34:37 That's cool. So you mentioned a couple tools there. See, it sounds like you guys are using snowflake on the analytics side. Is that something? Are you guys replicating from your product stack
34:46 into there so you can do analytics against it? And then on top of that, it sounds like you're using sigma. And I don't, we may or may not have brought up sigma in one of our previous episodes,
34:55 but could you speak about sigma? I'm fairly familiar with what it does, but I'd like to hear from the horse's mouth, someone who's using it, you know, kind of what it is and what it does And just
35:07 kind of that whole snowflake, analytics, you know, side of easy ops. Right, so most of our back and historical data, and it is truly, we now are a combination of four products I think, easy
35:21 ops itself. We
35:24 welcome two products on board it, and now we are a detection company. So we truly have like a wide range of products. So most of the historical data is hosted in true snowflake. through different
35:35 server buckets. And then the people from the BI team then get access to these queries or that data. And Sigma truly is the plugin play version of Power BI. Super easy to create that first level
35:50 view, super easy to set up something, embed something, super easy to export something. It's almost, when you do build a table in there, all of those operations look very similar to Excel So they
36:02 put, I believe the UI and the ease of the product in front and center. Of course, Power BI does have that benefit of being a legacy platform that has been up and running for 15 years. So the
36:15 network on that is extensive, but I believe that Sigma is focusing on how to ease those analysis and it's plug and play. Like you can very easily connect to database, create a bar chart, look at
36:30 an outlier Yeah.
36:33 So I think that's, you hit it on the head, especially on the Excel side, which is what I think Sigma's going forward. It's a spreadsheet like interface on top of Snowflake. I don't know if they
36:41 can connect to other, like, yeah, where I was like, big gray, but I know they're for sure on Snowflake. Yeah. But like, for someone like John, you can write an Excel formula type, or type
36:51 formula within the platform and everything compiles down to Snowflake codes, you know, behind the scenes, and it's running everything against Snowflake and super fast 'cause I think you can run
37:01 Sigma within Snowflake like their marketplace now to it, where it's almost like right on top in the same server room, so, you know, reducing latency and all that stuff. But it's definitely an up
37:11 and coming platform. I've heard a lot of people utilizing. I know a lot of accounting departments love it because of the Excel
37:17 component where the accountants love their Excel and their pivot tables, so they feel right at home. Correct, yeah. I mean, I shouldn't have to write 20 line code to find an average of a column,
37:29 right? And that's what a lot of these like.
37:35 It's so complicated to do a simple thing and I think Sigma is trying to eliminate that. That being said, pros and cons for short, like every platform has. Yeah.
37:46 No, but you're right. Just the ease of the
37:49 user side of it is important. I mean, I was talking to Bobby yesterday about trying to extract this one field from this really weird, I think it's like a select a list that's in a CSV. And you
38:03 know, I was doing it in R. And like, I like R. It's very straightforward and easy to use, but it's like, I've got to go write a code. I've got to go right, you know, a full script in order to
38:13 do some very basic things because that's how it is instead of just like, Oh, I could just pull this into Excel and click a button and then it'd be done, but it doesn't codify it.
38:42 All right in my back. Sorry. I think are we good? I like I cut out. I think I could let's do it. We're good
38:55 Okay, so all right, all right, so like now let's get into that So obviously you guys or there's data being replicated to snowflake and many this is a good part of talking about like how How do you
39:06 share data from the platform? I am sure companies want to be able to take take data out of you the ops and then pull it into their analytics platform So is it are you able to share data using
39:15 snowflake data shares or do you expose API's and just here? I how you all share data with with your customers Yeah, we you you said it right there. We have a data share Policy of where we can
39:29 create specific views or calculations that are client-like and then they have access to it through data share we also do some exports as well. If there is a certain accounting team or a certain band
39:41 of leadership that likes some KPIs like the total tickets created or the total inspections that failed in the month of September, then we also do those automated exports that go at a regular cadence.
39:54 So they can get access to the backend data as well, but we do also build
40:02 reports embedded into our platform just to support our vision of let's keep everything in one place and kind of ease that access of information.
40:17 Awesome.
40:20 I'm curious. Brand early. I'll go ahead, John. Now I was just going to ask about kind of
40:28 the buzzword, AIML stuff. One, what kind of, what does that look like under the hood for you guys? What kind of general things are you all looking at aggregating over windowing all of that fun
40:44 stuff? And the second part of that is,
40:48 you know, what are some of the, like, really interesting, do you have any interesting kind of use cases or like wins that you guys have had over the years? Or it's like the simplest, you know,
40:59 it could be the simplest little thing. But because they started tracking it, they're like, oh, we didn't know that. And then it just completely changed something or provided a bunch of value to
41:06 them.
41:08 Yeah, no, I can definitely speak to the AI aspect It truly is personal. buzzword 10 years ago when I was trying to get into my majors for my master's. It was the new world then and things have
41:23 changed so much. Everyone is talking about AI, everyone has charged empathy at the tip of their fingers but we truly do try to take a step back and see if it truly is required to implement something
41:37 sophisticated or if the pain point truly is to get someone to collect all the data together and apply the simple heuristic and present information in an easy to read format. So we do have two
41:50 parts of data science components that we have life. One uses a heuristic model which essentially is a thumb off rule business constraints that we already know like Brandon already has so much
42:02 information in his brain about what the ideal window is to complete an inspection or if between if I have to pick between an overview. beginning event or an overview inspection event, which one is
42:14 given a higher priority. So we try to kind of take all of these roles and compile them into these heuristics. So the operator optimization aspect of our product is based on the heuristic model,
42:29 which is not a sophisticated AI model. It essentially what you said, John is getting information together and applying those business constraints and then applying routing on top of it to make sure
42:40 that there is also GHG reductions. And the second aspect where we do chemical trucking optimization that actually uses an optimization software called Optoplanor, which is really good where you
42:57 define a universe for Optoplanor In our case it is tanks, the tank reads where these tanks are situated and are hard constraints or please do not let any tank run out. because if a tank runs out,
43:10 then it's not pumping, methanol in the pipeline and the pipeline is freezing. So we kind of set all these do's and don'ts into the system and it will come out with a plan to optimize cost per load.
43:24 So those kinds of things, heuristic modeling and opto-plan or based optimization is where the product currently is at. And I think I will defer to Brandon for sure to share some exciting wins I'm
43:37 sure he has had many. Yeah,
43:45 one thing that we found,
43:50 and actually I'll throw this back on you 'cause I'm a little bit rusty on the numbers, but I think it was something like 50 or 60 increase in performance when you augment people with operator
43:58 optimization. So it was super cool. We knew that it would be valuable. We knew that they're an impossible situation. We knew that they couldn't possibly synthesize all of these different pieces
44:07 but actually seeing the outputs of optimization. You have a super goal. I don't know if you have those handy or oochie, but that's always gets me excited when the vision actually works and you're
44:20 helping people.
44:24 It's just one of those things you don't know, you know, like as the
44:29 end user, you have this idea, right, and you're like, I know that this is valuable because I spend so much time doing this or because it leads to so much error on this or so much rework or
44:39 whatever. But until you, like, it's that chicken and egg thing, right, like, I need the money to go prove to you that it's gonna work. But then, of course, they don't want to spend the money
44:48 until you can prove to them that it's gonna work. Or, you know, eliminate costs or increase or reduce downtime, all those fun things. And so it's even that, right, like, I think that's one of
44:59 the reasons I asked that question is because I knew it was gonna be some very simple, like, thing, but very impactful at the same time, because there's just so much, there's still so much of that
45:08 opportunity in this industry, where it's like, what you said or shoot up, it doesn't always need to be AI or ML. If it's just a basic nested if statement, and it works, then that's all you need,
45:22 right? We
45:24 don't always have to use the sexiest thing. You need to use the most robust thing given the conditions. So that's really cool to hear y'all say that First benefit analysis is what you obviously is.
45:36 How long is this going to take to implement and how much are we actually impacting the users by doing this really sophisticated model that will probably take three months to even clean the data and
45:47 the implementation and the scalability. And before you know someone else has done it. Well, that doesn't generalize. Like, you know, you could train an AI model and it works, you know, in this
45:58 part of the matinee, but then you take it over to somewhere else and it doesn't hold water. Um, you know, so it's just kind of, again, you could put to your point, you could pour a lot of
46:09 effort in and it still doesn't give a robust answer.
46:14 Yeah, the 80 20. Yeah. Works for. Yeah, yeah. Always for radio principle. It just comes back all the time. Um, so I kind of brought up. Mottany or like, so Brandon, Brandon, you said you
46:25 guys have like 27 of the Canadian market. Um, are you specifically in Canada currently or did you guys break into the US yet or is that part of this? Protection, um, you know, merger as well,
46:36 maybe expanding that footprint. Yeah, that's part of the detection piece and yeah, happy to dive into even the logic of why we ended up there. But yeah, no, yeah, easy ups before detection was
46:47 just in Canada. We're basically bootstrapped, raised a bit of money, um, but bootstrapped. So, um, just really cost effective in one expanding.
47:01 Congrats on that, by the way. No, that's not bad. No, bootstrapping is very hard. And then it's of course always against the trend, right? Everyone sees all the sexy VC and private equity
47:15 money flowing around and then they think they need that. And most companies in my opinion don't. And there's all kinds of worms and follies that come with that as well. But
47:28 I wanted to ask in that line, what, who is y'all's like target kind of user? Is it mid-stream, upstream, downstream? Is it all at the above? Does it matter as long as you have an asset? It's
47:41 going to need maintenance. It's going to need people that people work on. What's the target user historically? And then also kind of moving forward with this,
47:51 with a new merger acquisition? Yeah, you bet. Yeah, so typically it's upstream because it has complex operations over large geographic areas with human decision making.
48:04 that needs to be augmented. That's where we get the best bang for our buck. But we also get it pulled off the shelves as well for the mid-streamers. They still need to manage their PMs and they
48:15 still need to, you know, they still, it's just in a smaller, same problem, it's just smaller in geography. So it doesn't have quite the exponential problem, but it's still a problem that's
48:25 there. And then, yeah, just building on that. So with the reason why we, you know, when I was looking out into the future, there's going to be winners and losers in the space in five years. So
48:36 I want to make sure that you see us as a winner. So looking for the best partner to make sure that we would be successful. So looking for partners that had the cash to expand into the correct
48:48 geographic areas and to invest into the team to solve some of these more complex problems to get beyond the 8020 rule. And then also complementary products. So in the oil and gas space, it's pretty
49:01 difficult to get data sharing. You have to have agreements in place and fucking this and that. And so having everything on their own one umbrella allows for one MSA and now you can transfer the data
49:14 and you can actually solve some of these problems. Whereas if you had lots of other companies out there, now you just have too many roadblocks to share the data and get the results you're looking
49:27 for. So we wanted to find a partner that had a complimentary product And then also, I think I mentioned before we kept on here, I dropped out of high school. I don't think I'm the right guy to
49:37 take over the world with
49:40 augmented intelligence or blue collar workers. So to me, it was really important to find a partner that had an awesome leadership team that could take this and had a very similar vision. So that's
49:50 what I found with detection. And that's why it looked like it would be a really good fit. But what detection does on the compression side of things, It's funny, actually, I used detection back in
50:02 2019.
50:05 with a producer and wasn't that keen on the interface of the frontline worker of it. But now they've done a lot of work in there and they know how to integrate with the frontline team. So we're
50:19 pulling that data in through, whether it's data systems or field data capture and having compressors run at their best is one of the most important things you can do When I look at a field
50:33 holistically, you want to have your plant inlet pressure as low as possible so that your gathering system as low as possible, that your tubing and surface resilient pressure as low as possible and
50:44 then your reservoir, you're going to get the most production, you're out of the hydrate curve. Like having your compressors running properly is there's so many valuable pieces to that. Obviously
50:56 it's the increase in production, but then your op-x is dropping significantly per BOE And.
51:03 Yeah, so I really understood the synergies there and how valuable our data would be to them and also their insights back to the frontline teams. So that's what we're working on now is being able to
51:15 push that data, those insights back to the frontline so that they can take the right actions. It looks like we have bad valve or volume pockets that need to be adjusted or
51:28 things like that
51:31 Cool that y'all found a complimentary partner like that and it wasn't like a, you know, I'm a competitor, I'm just gonna come buy you out and show of you because I don't like competing with you.
51:42 That's,
51:44 I hope the industry continues to have mergers and partnerships and stuff like that where we start unsilowing all of this data and realizing that, hey, everybody's better if we share this data
51:56 together and. Yeah, and rising tide, let's all vote Mm-hmm. But, I mean, to that end, I mean, protection, like you said, used in 2012, but they've been around 20 plus years, at least,
52:07 right? So we've got some staying power.
52:10 Yeah, absolutely, yeah, they're market sharing. What's your stronghold? Yeah, they're global. They have a presence in Australia, North America, I think, even in Europe as well. So, yeah,
52:25 good land and expand strategy, and good and diverse insights on the oil and gas market So, that even really helps from a product perspective. So, when you do build something that's scalable to the
52:36 entire world, you know, there might be small adjustments, but at least you have the insights from the difference from the Middle East, but I want me to walk in, yeah. Yeah, absolutely. I guess
52:51 I'm into the whole world as kind of a big geographical area then, right? I mean, for easy option scale
52:57 Got lots of room for opportunity there. I wanted to ask about the gamification part that you touched on because I think we're actually working on gamification for collide right now, but I think it's
53:09 a very overlooked thing in our industry, even though the benefits of it are, you know, you can look up a number of case studies across every industry, basically, and you can see the benefits of
53:21 it. But I think that's something that our industry has been slow to kind of adopt for whatever reason. So I'm curious what, one, what was the idea behind that? Was that something y'all started
53:31 with, or is that something y'all kind of added over time? And then two, what kind of, you know, performance or benefits have you seen from it? You know, I love that intersection of like human
53:40 nature and technology where it's like humans are generally competitive, right? So you throw a leaderboard up for, you know, all the pumpers in a region. And now they're instantly incentivized
53:52 just because their human nature wants to be, you know, to move up the list or whatever. And so it's just, it's so simple, but. you can also do it very poorly. It takes a lot of nuance and stuff.
54:05 So I'm very curious about that.
54:07 Yeah, maybe I'll just dive on a little bit of vision stuff. So one thing that I always thought forever is that people should be compensated based on the value they bring to an organization. I think
54:20 that that's a there's lots of people that could compensate it too much. And everyone brings value in different ways. It doesn't necessarily need to be in logic. Like it's a team, but that's the
54:28 complexity of compensating people based on the value they bring to an organization because there's tons of different ways. But I really think that the current system of paying people per hour to do X,
54:39 Y, and Z is really archaic. Whereas there is that I kind of see in the future, people should be paid based on the value they bring to an organization and the platform like easy ops where you can
54:51 actually see what needs to be done and what has been done. And the end outcome of that, you should be able to start actually integrating compensation. So. when my team at Reliance utilizes easy
55:02 ops, there's a pretty heavy bonus structure that's tied into the usage and the value that's being generated in the back end. And it's still kind of in its infancy, but does tie that gamification
55:16 component. And then, yeah, that reward performance management, I guess.
55:25 But maybe I'll throw it over to you. I'm going to show you. Like with your gamification, yeah Right here. And in
55:30 terms of data science itself, you cannot truly define a problem without defining what clear performances or what goals are. It's essentially like having a target variable. Why, as we call it in
55:46 the industry. And it is so hard to correlate all of these different aspects of an operator's life to the KPIs directly is, if I do miss a big, that is to be sent daily today, how many decks of
55:60 production am I using? There is no clear answer of that, especially because the impact is not instant, the impact is delayed and the impact is not quantified for all of these different events. And
56:11 when you do actually take a holistic view on a problem like that, your step one is identifying what is the impact of every action that you're doing on what your goals are. And our goals were
56:22 maximizing production, reducing operational expenditure and kind of leaving the field in a safe state So we needed to correlate every input and every aspect that we considered to back and tie those
56:34 back to our KPIs. And what better than numbers?
56:40 Yeah, no, I agree. I also think you're spot on brain and I think like there's the whole web three kind of movement or whatever, but the root of that is returning control to the creator,
56:52 essentially to the person that's actually doing the work. And I think that is absolutely gonna happen, especially all the way, you know. and industries like this where you have so many hourly or
57:03 contract type workers and things like that. And it's like, well, it's not just commoditized on the price, like your price should be dictated by your performance. And we also know, you know, how
57:14 long it should take to do these things. And so it's not just, okay, when you get paid by the hour, you worked eight hours today. Here's your money for the eight hours. It's, you know, you
57:25 impacted the business X percent Therefore, you get some percentage of that impact directly as an incentive to keep making the business better. And to do, you know, we're also talking, you know,
57:38 especially with the last, what are five, 10 years, this lower for longer oil price, commodity price cycle, they want to do as much as they possibly can with as few people as they can. And so I
57:50 think things like that also line up perfectly with that concept. So that's really, really interesting. But yeah, I wholeheartedly think way more software companies in our industry should be
58:02 looking at gamification. And regardless of what it is,
58:09 it's just way more engaging and a better user experience in most cases. Well, what we hear similar with
58:16 Ryan Rice, with resmenai, and I think, and again, the genesis of that was back to what rice, rice, energy, rice, petroleum, whatever it was. And then I'm sure I'm pretty sure Toby and then
58:25 we're using it over EQT, but when they start off with a sales force, but just, and I think, again, Brandon, you said earlier, this platform is targeted at blue collar workers, and I think they
58:35 aren't incentivized that money, but I think a lot of them are also incentivized by it, knowing that they did a good job and a job well done, you know, they worked hard. And then when I go home,
58:43 they want to know that what they did was impactful. So I think when you get that feedback looped, and I think it's just important for pride morale out there, 'cause otherwise they can just be out
58:51 there, you know, just like that turning wrenches, do all these things, like, all right, I guess that mattered. I don't know.
58:57 So I think it's having that direction. And again, I think like you said that something that can flow from the top way, what good looks like or what winning looks like. Well, it's also one of
59:05 those things too, where it's like when Bill and Bubba go get bar or get drinks at the bar that night, Bill's gonna talk shit to Bubba because he's higher on the leaderboard than him. And that's
59:16 probably gonna incentivize Bubba to do more, right? Like there's this an internal psychology that happens from it that's just so fascinating to me Because at the end of the day, like all of this,
59:27 all the stuff we're doing ultimately gets put into the hands of an individual and it's up to them to use it, use it as effectively as possible, use it as much or as little as they want, right? And
59:37 so having these little psychological, I don't wanna call them tricks, but triggers maybe is the right word to just incentivize people to be competitive, right? Like competition tends to work
59:48 really well most of the time
59:51 Yeah, early on we actually had a performance management coach. involved in the product, actually. So he had gotten to come in, behavioral scientist, to kind of, you know, run in, like, what
1:00:05 do we need to be considering here to be driving performance? So it's always kind of been on the helping guide our vision. Yeah, worker happiness is huge, and it helps with retention, and that
1:00:17 compounds on itself from corporate performance, as well, when you can keep your retention
1:00:24 team members And when you do expand, not needing to hire as many people, now you have less lines of communication because you don't have as many people involved, which is a good thing. Last word,
1:00:34 more streamlined communication. You can do more with less.
1:00:42 I think, Johnny, I think more, you want to jump in the speed round or? Yeah, let's jump in the speed round. I think, I mean, I could talk all day about a lot of this stuff, so it's not a
1:00:53 problem. I think I'm going to ask Brandon, Canada specific questions for the speed round. It should be fun. What's your favorite Canadian sports team, Brandon?
1:01:09 If I didn't say the Oilers, my wife would kill me, but I'm not
1:01:15 a hockey team. But Sheila's hockey and, you know, being an Alberta, Northern Alberta, you have no choice.
1:01:24 But there is some rivalry. We have, you know, one of the easy ups. I think actually our Calgary team is larger than our Edmonton team, so I, you know, definitely kept my thoughts to myself,
1:01:37 but there was a little bit of rivalry over there.
1:01:43 Rashida, what's your favorite open source library or analytics library
1:01:50 or tool? Okay, I'll try and keep it broad. We've asked, I don't want it to be too specific 'cause that's really hard to pick most of the time. Yeah. I mean, I swear by psychic learn, they have
1:02:01 everything you need under the umbrella to just get started, you know? Just build a tiny predictive model to predict if an image is a cat or dog to doing something real with healthcare data. You can
1:02:14 actually truly get started and that's why I love Jupy to Notebook is you can get your hands dirty. Just have a 10 line CSV in there and start learning. Yeah, yeah, 100. There's so much that's
1:02:30 just so easily available now, right? Like,
1:02:34 you made me think of Owama when you were talking about that just now, but like, Owama is just a, it's an open source. tool that lets me deploy local models, local language models on my computer,
1:02:47 but the Owama install is literally two lines. And then to start it, it's Owama, whatever the model name is, enter. And then I've got a language model deployed on my laptop instantly. And it's
1:03:01 like, this is nuts. Like, this is crazy how simple some of these things have become. Yeah, I agree.
1:03:10 What you got, Bobs?
1:03:13 We're going to go to Canada Pacific for Brandon.
1:03:18 What do you like better? Tim Hortons or ketchup flavored potato chips
1:03:28 in a possible situation. Yeah, our chips are pretty good up here, but yeah, I'm not actually much of a ketchup chip guy, and I'm actually no longer much of a Tim Hortons guy either. So, So.
1:03:45 If I was gonna come up to Alberta, where should I go? There you go, that's a good question. Where should he go? Oh yeah, the Rockies for sure. I think you guys checked out Tammor when you're
1:03:55 here, but you guys just keep going on in there. I'm on that day, yeah. Yeah, Alpine hiking, as soon as you get above the tree line in the Rockies, it's just gorgeous. So whenever you get the
1:04:07 chance to get some long hikes and it's good It's like, hey, it reminds me of video games, literally, like you're just driving down the highway and you're like, Holy shit, where am
1:04:22 I?
1:04:25 All right, and then,
1:04:28 Ruchi, what's
1:04:31 your, well, you mentioned it before, you know, you can answer that or not, but like, what's your favorite podcast or book that you read lately?
1:04:41 I like bad friends a lot for a podcast. It's because it's just two people talking absolute garbage and after a long deal of hope that's exactly what I need. Yeah, you don't need to, you know,
1:04:54 turn your brain off a little bit, but just kind of, yeah, let it go or it takes you. I'm right there with you. Bravo is my, my outlet for that with my wife every night. We watch some, some
1:05:07 stupid housewife show or,
1:05:11 yeah, we're on love is blind now. We
1:05:17 just finished love is blind. They can't get better than that.
1:05:22 Oh, man, um, one more.
1:05:29 Actually, I'll, I'll pivot Brandon. What's a, what's a either good book or podcast or follow somebody that you like that, that you would recommend for someone either,
1:05:40 that you would recommend for someone that's looking at either starting a business, running a business, building a business.
1:05:46 Yeah, you bet. I'm pretty sure everyone kind of listens to the online podcast already, but that's a good one And then one thing that I guess was the most impactful for me is Patrick Lynchione,
1:05:56 just, you know, creating a healthy organization advantage or the ideal team player. Like, just the culture that we created was what allowed us to kind of do what we did and with the efficient team.
1:06:13 So, yeah, just having a healthy organization and making sure you have a line values But, yeah, those are the biggest things. And then from a product perspective, like Marty Teigen and Silicon
1:06:24 Valley, product group and stuff.
1:06:29 Yeah, no, let's see how he's good. We've read a few of those right, John, at RDS, like think five dysfunctions of a team and death by meeting and some of those. So yeah, he's definitely, you
1:06:39 guys have just written the book, he's written a few. Yeah. Yeah. Yeah, and they're all really good. I'm right there with you on the culture side. I think people overlook culture as far as like
1:06:53 keys to success for startups or companies as a whole, but culture plays a huge part in so much of it.
1:07:01 Yeah, we had a really rigorous hiring process that we didn't care about credentials, kind of at all. Like the first things first is it was, I don't think anyone knew out of the time, but it was a
1:07:12 15 minute quick connect. And really it was, you know, does these people have the same values as us? Or is there any anti-values? Just like this very simple binary yes or no. And we were just
1:07:22 like hunting out-nose And if there was no nose, then it kind of got kicked into the technical side just to make sure. that they could believe. But yeah, a lot of great interviews I've left in
1:07:35 feeling like, man, if there was a war, I'd want to be in the war with this person, you know? So you know that that's a great purpose and bring on the team. And that's another thing why we were
1:07:46 successful. I think was just that that got intuition, which was alignment of values.
1:07:53 And then just protecting that in the team. Yeah, Colin made a post. We're trying to hire a Ruby on Rails dev here in Houston. He made a post earlier this week. Just like the salary, I want to,
1:08:06 you know, badass coder that is going to got equity all the stuff. But I need someone that's willing to dedicate, you know, extra time and all this stuff. And there was all kinds of comments on it
1:08:18 and stuff. And one of them was something to the effect of, well, it sounds like you're, you know, cutting out a lot of potential candidates just by doing it. for them only being fused and based.
1:08:28 And Colin was like, our goal is
1:08:32 to cut as many people as possible. We're not looking for a general person. We're looking for the best fit for this very specific thing. And I think that's how you said it is a really good way to
1:08:43 kind of talk about it, is you're not necessarily - you don't want anybody. You want the right fit, right? And so you are actually culling. The idea should be to cut as many people as you can to
1:08:54 get to the ideal candidate Not find a bunch of people and then try and figure out if they fit or not.
1:09:01 Yeah. Yeah, absolutely. Yeah. One more, Bobs.
1:09:10 Hi, I asked Brandon where I go if I went to Alberta. Richie, where would I go if I go to Toronto? What's the best thing? What would you recommend? I mean, Toronto truly is a melting pot. So if
1:09:22 I were to recommend, it's definitely going to be a food place I really like. Thai cuisine, so pie is one of my favorite places to eat, yeah, a good, yeah, there's a lot of good Asian spots,
1:09:34 but Thai is my all-time favorite.
1:09:38 All right, well, we're gonna take you to some Thai food when you come down to Houston, because we have a really good Thai food down here too.
1:09:47 The real question is, do you get it Thai hot or do you get it normal hot? It's been seven years, so I have been whitewashed
1:09:59 Yeah, so no Thai hot for me, no.
1:10:02 A guy Bobby and I used to work with would take us to this hole in the wall. It was a Thai grocery store that had just
1:10:09 a Thai restaurant inside of it, and he would get the hottest, and they would ask him because he's just like, you know, this. this white guy from South Texas, you know, like has no business
1:10:19 ordering this. And they ask him multiple times, are you sure? He's like, yeah. And he's, you know, sweating and crying and stuff. And I'm like, I have no desire to go that hot. I don't
1:10:28 understand how people eat stuff. Yeah.
1:10:31 That's another level of hot, for sure. Oh yeah. Yeah, yeah. I'm not tasting food at that point. My tongue is literally being cooked as a meeting.
1:10:44 Awesome. Well, we really appreciate you guys joining us Yeah, thanks. Yeah, I know it can be a little tough remote, but you guys did awesome. And, you know, I'm excited to see how it kind of
1:10:54 comes together with detection and seeing you guys break into the different markets. So. Yeah, where can people find you guys if they want to reach out?
1:11:03 Cool. I can, yeah, and I can drop my detection email.
1:11:10 Cool, so both on LinkedIn and then what's the detection website? Oh, it would be. RZARIO, R-R-O-Z-A-R-I-O at detectioncom.
1:11:23 Perfect. And then just detectioncom if they want to check out the page. Yeah, that would be right. Yeah. And the detection comes with a little surprise. It is a detection. T-E-C-H.
1:11:38 Tech. Got it. Why did I not pick up on that? I appreciate that I've
1:11:46 read the company name. Yeah. I've read it a dozen times. I mean, I'm a
1:11:51 dad. I love dad jokes and puns. So, sign me up. And John, you know, naming hard. We were sitting there trying to come up with Avid and all those. It's just like naming it so hard. Or you can
1:12:02 overcomplicate it if you're analytical like us. But yeah.
1:12:07 That spreadsheet that we had Bobby was pretty epic. It was Yeah, no kidding.
1:12:15 We weren't doing the real grunt work like that.
1:12:19 Well, on that note, we appreciate it guys. Thank you so much for joining us. Everybody, please go check them out. Detect it, detect TECH,
1:12:28 IONcom. We'll see y'all next time. Thanks. All right, thank you.
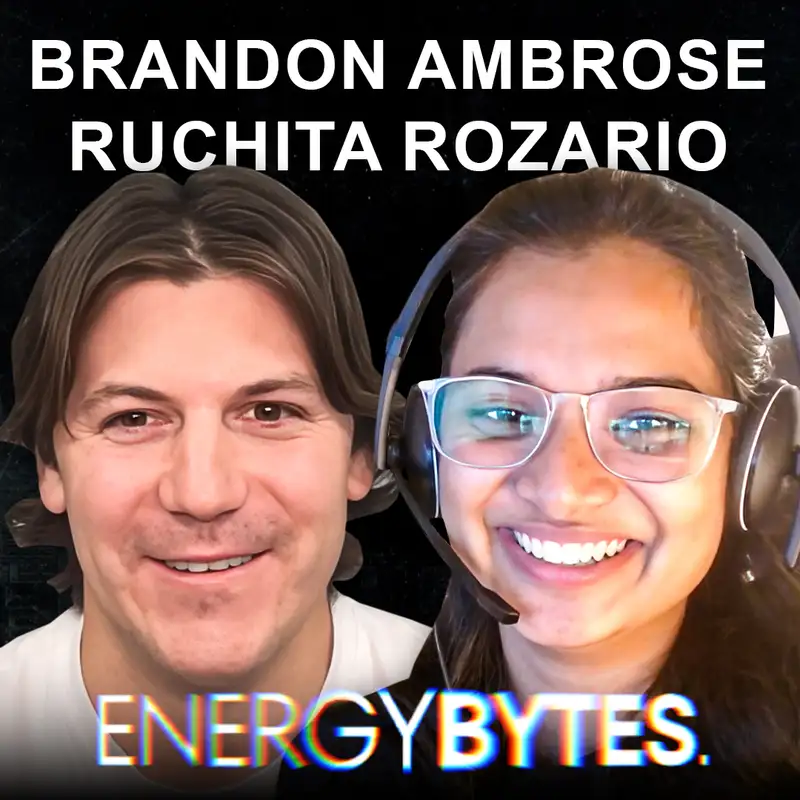