EP 56: Spotfire: The Ultimate Guide for Data Scientists
0:00 Welcome to another episode of energy bites. I'm Bobby Neeland. Got the rad dad here, John Calfan. What's up, man? I'm happy you're you're here. I know. Finally. Gone through your transition.
0:10 Well, let's let's be careful. What kind of transition we're talking about here? Job transition. The job, yeah, which isn't even like is still also a deceptive thing. Because it was just, yeah,
0:20 it's just not anything. Yeah, I was like, oh, today, I'm my domain changed. Yeah. Yeah, that that well, and it didn't, but that's a whole other story Yeah,
0:31 but you know, who picks who are you, right? Yeah, buddy. I'm wearing my DMACC shirt today because we somehow squeaked out a win. I went to bed and I was like, wait, that happened? That, well,
0:42 yeah, it's weird. So now my superstition, we didn't watch it. We were at the Fort Bend County Fair. Highly recommend that next year. I saw you guys went to Zack Top. Went to Zack Top. That was
0:51 nuts. I heard the traffic was insane. It was like two hours to get from Needville to Rosenberg Yeah, that we were, fortunately. not on that side of the parking lot. So we didn't have it quite
1:01 that bad, but there were a ton of people at that tiny fairground. So it was really, really fun, really awesome. We ended up taking the kids down there on Saturday and still just watching the
1:09 football game. And so now I'm like, well, do I need to just quit watching the games and probably keep winning? And we'll see. I'm very superstitious when it comes to the Arkansas football. Sweet.
1:19 And then I'll let them introduce themselves, but I'm excited to have some of the folks from Spotfire here. Thank you
1:27 My name is Ethera Latar. I'm a principal data scientist now. My background is a mix between petroleum engineering. I had masters from
1:35 LSU and data science from Georgia Tech.
1:43 My experience is being in the oil field for the, let's say, kind of like the first part of my career. Now
1:52 for the last, I guess, eight years
1:57 Oil and gas, but like the data science aspect of it have been in mid-stream and have been with SpaFire for almost, I guess, years.
2:07 Dealing with all the oil and gas customers. Thanks for coming on.
2:12 All right, my name is Joselea Yire.
2:16 I am a telecommunications engineering, engineering that's my background and then I did a master's in computer science. And I've been working with a spot fire, not with the company, but with the
2:28 spot fire itself for many years since 2005 or something like that. I got another 10 years on me on that. Yeah, I mean, on and off, not full time, but it's always a tool that I always admire.
2:43 And I worked for Shell for 10 years as a contractor. Then
2:50 we did, I became a consultant, independent consultant of a spot fire as well. then one of my customers hire me. So it makes sense. And then I had the opportunity to move to Chile as a manager of
3:05 HSC and reporting analytics and assurance. So I had two teams. I didn't know anything about HSC.
3:12 My interview, they asked me, Do you know about HSC? And I said, No, I don't know nothing. I don't know why I'm interviewing. And they said, But we don't know anythingabout reporting and
3:23 analytics So, but your team knows about HSC. And I think that's a good match. And you don't have to know anything about HSC. You're just gonna be managing those things. And it was a great
3:35 opportunity to be an expat in Chile. And then that was during the pandemic. That was also during the social unrest. Okay. And I mean, it was a good experience overall. And then he was time to
3:49 come back and how we're back home. Very nice. Cool Yeah, I think that's a fun thing about that. kind of the data world to those. Like you learn about all these other aspects of different
4:00 businesses. And I think it's a chicken or the egg thing we talk a lot about, you know, you need the domain knowledge to be an effective data, you know, professional data scientist or whatever.
4:09 But at the same time, if you just have that aptitude and willingness to learn, like you can also learn what you need to know about the business. And then if you have those data skills and then are
4:18 able to apply them to those domains, like it's, you know, that works equally as well, I think. I think it's just that willingness to learn Absolutely, that's a,
4:28 I think it's hard for anybody in this industry to kind of fail if you have that mentality. I like, it's one of my favorite parts about our industry, generally speaking, is, I mean, there's
4:39 plenty of egos, don't get me wrong, but if you come in with this willingness to learn and kind of an open mind and not, I know everything because I have this degree or whatever, people will help
4:50 you and people are very happy to because they understand the longevity. We need more people in the industry. Yes, I like to bring a quote from Charles Darwin. I went to a botanical garden not too
5:05 long ago, and I caught my eye, some quote that said the species, because he was the oldest plants and everything. You see how they evolved and how they adapt. And
5:16 one of the quotes was the species that survived is not the strongest or the smartest, is the one that adapts. Adapted, yeah, 100. I mean, that was probably my favorite thing about Grayson Mill
5:29 coming in. Because I'd always done the data stuff on the subsurface kind of side, but especially with reservoir engineers. And then when John and I worked at RDS, it was a lot around the frack
5:38 side and all that. And I knew that domain pretty well. I worked at University Lands and got a little bit more exposure, but I never worked land in accounting or any of that. We came and built a
5:47 data warehouse, and we pulled a lady on my team who was a reservoir tech, so it made more sense to give her like a lot of that subsurface domain and then it's like, all right, well, I guess I'm
5:55 taking land and accounting. Let's figure it out, let's understand how a GL works. And I got to understand what a division order is much less like how this whole thing fits together. But that was
6:06 probably one of the most gratifying things just to see how being at the core of it and seeing how an oil and gas operator operates and just how integral the data working between all these different
6:16 groups was. So
6:18 yeah, it's a fun profession. That's the thing that's why we're all in here talking about it Yeah, especially nowadays, it's very important to kind of have this willingness to learn how other
6:29 things work. It's
6:32 no longer the time that you focus on only one skill because especially domains
6:41 like data science in other industries, they're evolving very fast. And I think you can see this old that's craziness of the gen AI I was gonna say that's like that augments people to the fact, to
6:54 the point that can be more, like if you're curious and you want better to use new things, you can, right? Like,
7:03 I don't know how I've been in the data side for so long and still never really learned how to write SQL, but I don't know that I have to anymore because GPT can do it really well for me, right?
7:15 Like it's a weird thing, but it's also one of those things that's like, as a mechanical engineer, I had no idea what would ever be writing SQL to begin with. So it's this weird augmentation, but
7:27 I agree with you that the future businesses are gonna rely on employees having a certain
7:36 step change in output due to AI tools, digital tools, AI augmentation. And one of the ways that they're gonna do that is by making people wear multiple hats and do different things across the
7:49 business line. very interesting to see how all of that was interesting. There's an interesting thing also, whether it's somewhere on LinkedIn that, I will not take your job, but an engineer who
8:00 uses AI will. Yeah, no, I completely agree with that. I
8:05 mean, at this point, we have to be aware that the AI tools are like, most of those tools are called co-pilot because they are not the, they
8:20 are not the pilot, they are driving So it's just a helping hand and I also rely that because I do a lot of programming. So if you put like a whole requirement of what you want and the wish list and
8:34 everything, it's probably gonna tend to hallucinate. So you have to be very, you really need to know what, you really need to know what you are asking. So you have to know your stuff in order to
8:47 ask pieces by piece. Okay, now you do this, now you do that. It's way better if you build up, especially around development, at least in my experience. But that's another thing I tell people is
8:58 it's just like, if you're worried about it or you're not getting good answers, force yourself to keep using it. And I promise you, you will learn, right? Like I did a talk this summer at SB
9:10 Oklahoma City on basically what language models are, what they are not, how they work, what they're capable of, all of this stuff, because the general public, I feel like, looks at them and
9:20 thinks, they're in one of two camps. They think they're magic, right? Like this is insane and incredible and it can think and understand or they think, okay, it's like a search tool. Who cares,
9:31 right? Like I'll just Google it. But the reality of it is like somewhere in between that, right? And so Google and Bing literally give you the AI, you know, answer at the top. Now you get your
9:43 results, you know, but it's one of those things It's like if you force yourself to start using it like I've been using this IDE called cursor, which is just VS code fork that has AI enable met.
9:54 And it took me a few weeks of like, okay, I gotta force myself to use this, but now it's like, man, I can't imagine not using it, right? Like it's crazy how quickly it can become like part of
10:07 your daily or weekly workflows once you force yourself to do it. But just understanding that they're effectively predictive text. And so if you're trying to search for something and it's all about
10:19 the context, right? So giving them as much context as possible, giving them the document, that's one thing that like, hey, you're trying to get it to write an API call for you, give it the API
10:27 documentation and ask it to write, like give it as much context as possible and it does a much better job. So it's a, but it's one of those things that you've got to play around with and learn
10:36 yourself or you're just going to be kind of scared of it. It almost seems like, so I think to jump in on some Spotify or stuff, you were saying how fast Everything's evolving in like the data space.
10:49 I want to start there, but even just higher level, what's new and exciting at Spotify and work back through different use cases and whatever else. So the way I look at Spotify,
11:24 it's a data science platform or like a hyper-converged platform where we try to bring all the steps of data science project in one place and all the, let's say, personas of all this data science
11:42 process So you start by collecting data or maybe like even before that. by connecting to multiple data sources, and then cleansing the data, visualizing the data,
11:59 start doing some modeling, maybe like EDA, and then collaborate with other teams, ending with, let's say, reporting your findings and then sharing your reports
12:16 and everything in between There are a lot of things also added to that. We have, for example, automation services, in Spark where we can automate like different tasks. We have alarming platform
12:25 or alarming extension with Spark Fire, where you can set up alarms based on, let's say, defined parameters. The other thing of Spark Fire that I really like is the extensibility, where you can,
12:46 if you cannot find something about the shelf. you can simply write Python script or like iron Python where you can interact with the product element. I don't know who to say. Yeah, well, I have
13:00 the definition right here. And it's, and that's what we always say is in our doctor's potfire monthly live meetings. It's, a spotfire is a visual data science platform that makes people smart.
13:07 That makes smart people smarter by combining advanced analytics and
13:21 industry-specific visualizations to solve complex business problems. So in my own words or in my
13:29 history with a spotfire, I've seen that, and I think that's my personal belief, that the spotfire, it's ahead of its time. And it's not just a tool, it's a platform. It's a platform that you
13:39 can extend in so many ways, there are different ways to extend it. And the different languages that you can use to extend its capabilities. But I think that Spotify has always been ahead of its
13:51 time. And now it's exciting moments because AI is more and more popular. People are more aware. But that a scientist uses Spotify
14:03 to analyze data. When I say it's ahead of its time, it's because many people, especially end users, use it as a reporting tool. I mean, there's nothing wrong with that It's just that it's like a
14:18 second nature of a Spotify to the reports. And people tend to compare that to other tools. Yeah. And it kind of forgets frustrating because
14:29 when you try to push to the limit, the capabilities of other tools, you can see that Spotify just is very natural. Can handle millions of data points and a map, for example. And things that we
14:41 take for granted, The marking, when you create multiple dissolitations, dissolizations, you can just click something and immediately use, you know, where it's coming from, and well, you know,
14:51 how it is. Yeah, no, I mean, I, I agree 100. And I think that's like, you talk about your frustration and my frustration kind of pulled over during COVID. And I actually like, because I think
15:04 Tableau came out and was like, Oh, we can put multiple layers on a map. And like, and like, I went on a rant on LinkedIn and I don't get like, it was Mark Palmer at the time, or whatever, I
15:14 reached out to me directly And I was like, why are you not marketing your tool better? Like, I mean, like, you've been doing this since I've touched the product in 2014, probably since 2005.
15:24 Like, I mean, you've been able to listen to people acting. This is a revolutionary new thing. And these people have so much market share, but like, you know, I just kind of called out the
15:32 marketing group and everything. And he's like, man, you really ruffled some feathers. You know, I was like, well, I'm sorry. And it wasn't for lack of, I mean, like in your predecessors,
15:39 like Neil and Rob, like they were doing a great job, like doing like their content and everything like that It's just like it was frustrating for all of us and I think it's frustrating for a lot of
15:46 us on the ground floor in oil and gas, 'cause if you don't have the right IT department, again, they're just comparing you to Power BI, that's free with their E5 license or whatever. Like, and
15:59 they're not even comparable tools outside of, I can do a pie chart or a tree map or whatever. You know, whatever. They both have dashboards is basically the kind of ability. Yeah, but like,
16:08 yeah, I can write some pandas in a data pipeline in Power BI, but it's not even close to the same of being able to call a data function and I can create a button Like, I would almost, I mean, and
16:17 you can tell me where I'm wrong here, but I would compare Spotfire more to like a shiny or a stream lid on steroids because like it makes it so much easier to do those things. Like it takes it
16:27 beyond just a basic BI, you know, if you want a basic BI dashboard, sure there are, there could be other tools there, but that's one of the things I think why Spotfire is such a staple in oil and
16:40 gas specifically is because we have so much ridiculous, large time series, large. I'll put that in quotes. We have a lot of large time series data sets, and it's very hard to visualize thousands
16:53 of points or millions of points across days, weeks, months, whatever. Tools like Power BI are terrible at doing those things because they're BI tools. They're not intelligence tools in that
17:06 aspect, right? And so just like, 'cause I went from spotfire to Power BI
17:11 and that, there were all these things where it's like, Well, I can't do that, I can't do that And so it was like going backwards, it was. It was like going from my current gaming laptop to the
17:22 laptop I had in college, 10 years, 15 years ago, forward. And so it's like, that's a whole 'nother, yeah, they kind of can do the same thing, but one is way better at doing a lot more things
17:34 than the other one. Yeah, so before I joined spotfire, I've been a customer or like a user. So yeah, from a user perspective, The way I look at it is. If you are an engineer or like, or if
17:48 your task is kind of like an engineering based task, I don't think you can do it any better than, I mean, with anything else, I don't think that's power fire. If you're doing, I don't know,
17:59 simple reporting or like a simple visualization, then yeah, you can do it with the tools. But once let's say the task gets complicated, like the agent as you said, yeah, I think tools like spa
18:12 fire
18:14 provides you with a lot of functionalities, especially just like the,
18:20 as I mentioned, the interactivity or like the brush linking, they call it between multiple elements where you can, for example, select something from a table and then then you get highlighted on a
18:29 map or maybe like a bring something like, oh, that will trigger data function. That will bring something else from an API data point or so. So it's that, like, changing your, like, yeah, yeah.
18:39 Lots of layers on a map, actually. Yeah, I mean, I mean, the big thing is like, anytime I hear someone, it's like, it's the GIS. I mean, like the, I mean, you guys are so far ahead on the
18:49 GIS side, you know, in that space. Like, it's just, even if, you know, again, if anyone has to use it, like, it's like, I can't do that. I can use other tools, like, oh great, you have
18:59 an Esri extension that's terrible and I need a GIS professional to expose it,
19:04 you know. But even, we could probably go on and on about different use cases, like sensibility, but I mean, I think I've got a medium article about there where, when I was at University of Lands,
19:13 they didn't want to use an SDE and our GIS got big on web feature services, like WFS, and it's like, well, I didn't have that connector natively, but, oh, go find an R script, pop it in and I
19:24 was able to, you know, connect those, bring it in, and, you know, now we've got a connector. And I mean, like, so if you don't have a connector, you've got a connector 'cause you have the
19:32 full Python library at your disposal, you have pretty much the whole R, You know, it's slightly different because you guys are, you know, doing a little bit different with the with tear versus,
19:41 you know, but you can still do vanilla R as well. Right. Even just the built in stuff, right? Like that was the first time I did a regression analysis. The spot fire had it built in and it made
19:52 it incredibly easy for me to do regression and clustering and like, you know, some of these basic analytical functions where it's like, I know what these are, but I don't have the time to go write
20:02 a full script to do this because I don't even know what I need to be doing it on, right? So being able to explore the data before you start narrowing in on certain things, it just makes it so easy.
20:12 And it's really, really great. You have a ton of data, right, being able to visualize, yeah, like millions of data points on a map or millions of data points on a frack job or a drilling rig or
20:23 all of this stuff. And some of these other tools, you know, when you put something like that in Power BI, it either doesn't work or you're limited to like a 15 minute window that you have to slide
20:33 the entire thing across, or you have to write your own. Python dashboard, and it's like, this is a dashboarding tool. Like, that's the point of this. Yeah. And even when it comes to streaming
20:43 data, you can, for example, bundle products like Spafire streaming. We have one of the use cases that data comes directly from the well-pat. And you can do, like, on-the-fly analysis cleansing.
20:57 And then you hook that up in Spafire, very simple. And then you can use mods or, like, Spafire native visualizations to
21:06 look at live operations. So let's go to Jose to talk about some of that, like, the JavaScripty side, like, mods, and, like, even just a text area, because, I mean, that's one of the most
21:16 powerful things, too. I mean, that's enabled multiple companies to literally build and sell startups off of it. I mean, like, Q engineering, I mean, utilize the hell out of text areas and all
21:26 that kind of stuff. But then we're still able to give people some native functionality that felt good in their hands But yeah, let's talk about that side of it. Sure.
21:37 The text that are very powerful are like a mini, mini web page that you can use used to put some documentation. But it has also
21:49 the power of script in JavaScript, which are web technologies. And then all you have to do is write your own code and do the magic with the text areas. Many of that code is already available out
22:03 there. So you can just copy and paste the code And that's it. You have to just copy and paste some of that code and you're ready to go. You can have pop-ups or sliders. You can beautify that
22:16 experience, that analytical experience to maybe get more real estate and you can hide stuff, panels, controlled filters. So yeah, that's the power of the text areas that you can do that And mods,
22:31 for example, that's also very powerful because you can extend it. Also with JavaScript technologies, custom visualizations. So let's say you need a specific visualization, very specific to the
22:43 industry, then you can program that visualization. But you don't have to know programming because there are many other mods or visualization mods available out there through the exchange, through
22:56 the community, that you can just download. For example, the web
23:01 app. Yeah, we've been doing a lot of energy specific mods.
23:07 We recently released an enhanced version of the web log mod where you can create multiple tracks, add multiple curves, you can also create a categorical track where you can, for example, highlight
23:19 the geology or the formation.
23:23 And then we also released
23:26 the gun barrel mod. That's right, yeah. That's the one you were talking about, energy forum, right? Yes. I was at Chevron, was working with you guys on that? one of those I think show from
23:38 Yeah. And then there is the combined 3D log, which shows
23:47 the formation tops and the well trajectories within each formation with all this, say the color coding that the trajectory falls within the zig formation, then
23:58 we'll be colored with that color And also there is the well schematic log. Oh, nice, yeah, and you can also with that, I think you can
24:11 show how, for example. The Okay. moving batch cementing,
24:16 moves, and then we'll kind of like, you can see like when it gets to kind of on an hour section, it will move faster. Oh, wow. Yeah, so yeah, we'll be moving the load up. That's suspicious.
24:25 So, real quick, I just want to give kudos on the gun barrel thing, 'cause I think, Everyone across the industry is a you've always had to start with just moving around circles on a power point,
24:36 and that's, that's immeasurable. But I've probably also coded my own gun barrel solution at Conoco, and - So that's the CME and all, I mean, everywhere. And like, one thing I hate more than
24:46 anything is a bunch of really smart people in the industry replicating efforts. Same thing. Like, if someone is like, just share it. That doesn't produce more oil. Right, right. Like, but we
24:58 don't need multiple people making2, 000 a year, working on the same thing Like, let's just like, you do it once, do it right. And then, again, if it's some pseudo-open source, then we can
25:09 collaborate if we need to enhance it. Yeah. I think there's certain things, yes, like their proprietary, whatever, but like, there's other things that's like, why are we fighting this right
25:17 now?
25:19 But two, like, can you guys kind of dive deep, talk a little bit, I think it's a good segue into about kind of how the structure at Spotfire has changed. 'Cause now it's just Spotfire, it's not
25:29 typical Spotfire, because you're your own business unit Um,
25:35 And then specific to energy industry, how you guys are really leaning into that. 'Cause I mean, like, and you mentioned, like you have now energy specific mods. And I think even maybe on the
25:43 data science side, if there's, I don't know if there's certain models and stuff that you guys have done, but it seems like you're really leaning into like, you know, helping push the industry
25:50 forward on those things.
25:53 Yeah, so TIPCO was,
25:58 well, Spotify was acquired by TIPCO in 2007, something like that And then TIPCO was acquired by V-stack with the partners in 2014 or something, I don't remember where, when, and ever since it's
26:11 been evolving. It's, it's stable for a long period of time with TIPCO products, but there were everywhere. There are different products from TIPCO and Spotify was one of those products. And then
26:24 V-stack with the partners bought Citrix and
26:28 it became, and TIPCO became like, like a new organization. that was in 2022, when it became cloud software group. Sounds right, yeah. So our CEO Tom Kraus is split it into different business
26:42 units. We have spot fires and independent business unit and then
26:47 TIPCO is another independent business unit and Citrix also is a business unit. And that gives us more independence and more freedoms and be more agile in that regard. So it's good to be I mean,
27:01 we're still settling because this was so recent. Sure. But we're very excited and very looking for what's coming next. I think it sounded like at the energy forum, some of the OG spot fire people
27:15 were coming back, people that had been there in maybe the
27:20 2010s. I think one thing is it's almost got a cult following. People love spot fire. I think at some level, if they moved out of the industry or different directions, or the IT department told
27:31 them Otherwise it may be like they got pulled away from it. but they all want a piece of it, you know, like they love the product. It's gonna say, it's hard once you use it and then you try to
27:39 use something else. And you're like, oh, this is a lot crappier. Yeah. Or it's like,
27:44 you know, the way that they go up, like bins, for example. I remember trying to figure out how to do bins on Power BI. Like the way that this is set up is so dumb, compared to how easy it is to
27:54 do in Spotfire. Just like the native, like the navigation of the UI, right? Like they do the exact same thing functional to the user. I can bin this data however I want. But how many steps it
28:08 takes, like those are the things, especially when you're a daily user of tools. Those are the things that add up and you just get like worn out by. Even if you know exactly what the flow is, it's
28:18 like if it takes me five more clicks to do it over here, every single time I have to do this thing, that's really obnoxious. Or like, yeah, some of the, again, Most of the time I'm using BI
28:29 tools, generally speaking, kind of time series data stuff and like doing that. How are we? We also need to remember that the origin of Spotfire. It was part of a research in the University of
28:46 Maryland. Yeah, with, yeah, and Snyder, he created this
28:53 tool. It was basically a scatter plot. The very first version of the Spotfire was a scatter plot, but it evolved So
29:02 Spotfire always has always been visualization and data scientist, data science tool, or platform, over the years it became a platform. So we're not a marketing company or a UX company. We're
29:18 basically the core of the businesses driven by the data science team and all the interaction that we have with the customers Hey, so we want this, we want that, we listen to the customer, through
29:29 the doctors' program, and then we - We give that information back to the product team, to the data science team, and all that feedback from our customers, evolving to maybe a new visualization,
29:45 more data functions, article use cases, as operators, things like that. Yeah, I feel like a lot of the other BI tools kind of, they almost try to safeguard the user to an extent, right? Like,
29:59 you know, our BI defaults a date or a date timestamp to a categorization or a categorized date instead of just a continuous date. And it's like, but those are my only two options, right? And so
30:13 it's like the little things like that where it's like as an
30:16 analyst, I want more control over how I visualize this, how this is laid out and you don't have those knobs unless you wanna really get under the hood with some of these other tools or they just
30:27 don't exist at all, right? Especially, I was thinking about it while you were talking, My only guess is very unique in the fact that you have all these different literal layers of data, right?
30:38 You've got subsurface, which has well schematics and surveys and logs and all this other stuff. Then you've got all the surface data, you've got the GIS data on top of the surface data, you've got
30:48 the real-time operations, like we have a very energy, like lots of data coming from all kinds of different formats, right? So it's a, yeah, like I said, it's one of those things, once you use
30:60 it and you understand how to use it, it's very hard to go use something else, because most things are inferior. And to talk about, to your point, that this has been for data scientists, probably
31:10 before data scientists was even like a broad term, because I mean, I get what, 2012 was like the Time magazine, they did a sexiest job, and I came in the industry at 2014, but even when I
31:21 started, they were actually some spot-fire developers we had at Conoco that were using S and a lot of people that don't know, S was the language that actually is what R was
31:32 So, I mean, you guys were utilizing, you know, the precursor languages to what everyone's using now in like data science and even like a name, I imagine you probably still can use S plus if you
31:43 wanted, you know, I'm not sure if it's supported anymore. I think you can't, I mean, for our, for sure, I mean, you can still use our insights by far, but my, let's say my point of view, I
31:57 started seeing like once we released the support for Python. I think that was, I don't know, a couple of years back. Yeah. Yeah. A lot of views that they started like moving to Python. Yeah.
32:08 Yeah. Away from our, uh, yeah. I think, and you guys, I think in the most recent release, at least with maybe Spotify or 14, I think it became even much easier to deploy those two, like the
32:17 web service to, like, because you still have a kind of hat, need to have like those spot statistics services. And it was kind of hard, but like it became, and I can tell, you know, pretty
32:25 trivial to. you know, move like those libraries to the - And the data canvas also has been evolving a lot. So now we can
32:35 basically look at the function, change the parameters, like back and forth between tables, like on both tables and output tables, and then look at the script, everything from the data canvas.
32:44 Yeah, you can see like on the whole linear - Exactly, and after the project. The Python engine, I think it's been, I mean, the speed of the execution has been enhanced So do you have, is it,
32:57 is it something, 'cause I know like Tare says his own kind of enhanced engine, like is your Python engine enhanced, or is it using GitHub? Yeah, yeah, yeah, yeah. Got a really random question.
33:08 And you guys may not even know the answer, but what like portion of Spotify user, how does the breakdown cloud versus like on-prem or self-hosted, self-managed? This is just a complete random data
33:21 question don't know I. Yeah, the answer for that, But I have a feeling that it's more.
33:28 It's not cloud, but the, yeah, on prem, on prem because of many, many reasons. I mean, that might be on cloud, but the, and maybe also the server, but the, but it's more in prem is more for,
33:43 because it's much more enterprise ready. And like now I feel is more like, you to get started and some of the, some of the companies, they have their own like proctor, like their own Python
33:54 packages And then they don't want to say, can I publish that? So, I mean, I've seen a lot of, I've never seen
34:04 a use case where there's like a large user base, the user, the clouds, the clouds, yeah, everything goes on prem. No, that makes sense. They'll, they'll give them a lot of control,
34:16 especially like when it comes to, to version control, or the code and like, like to use specific libraries from Ui5, yeah. No, I always like to see the server. I was like to see how the, where
34:30 the industry is at as far as how comfortable they are with cloud versus on-prem and stuff. And again, some of it, I mean, it's not a knock, but like it just, and I think it's gotten better,
34:39 like more peri, but for a while, like cloud was not a one-to-one to what was available to on-prem, like the feature set and everything. And like you said, like when you guys upgrade and in the
34:50 SaaS product, it's, you're getting moved, you know, 'cause this is where we're at. You know, whereas like, if we want to be on 140 LTS, you know, and we can feel comfortable that anything
34:59 that moves is not gonna break, so.
35:03 So when you talk about the mods, you know, like the library and stuff like that, but can you talk more about like, I'm struggling to remember like the exact name, but you have like a data science
35:10 library of like the different scripts and everything. Yeah, so we have our exchange, which has, you can download mods, you can download data functions. We recently started releasing
35:25 library called DSL. That's what learning is. And then this library has around like 180 functions and all the areas of machine learning and data science, cleansing, smoothing, modeling, time
35:41 series. Oh, you like read and score us and all those different things. Exactly, so you don't need to kind of like write a function inside your data function. You basically just call that the SML
35:52 library and then use like whatever data functions within that So that's also like you would install that Python DSL library in like your - Yeah, and your server deployment and I'm not sure if it will
36:07 come like pre-deployed or probably will come pre-deployed in the next version. That's pivot-soluble? Yeah. So I mean, like, now like I'm ADD, like if I was working on the rabbit hole, if I was
36:18 working in ZVS code, I could leverage that potentially. Yeah, it's a
36:25 PYPI library.
36:27 So if I feed it a pandas data frame, it can do all the things. Yes, exactly. But instead of, let's say, writing a long code, I mean, you can just simply - for example, if you have a missing
36:37 data issue, then you want to do imputing based on
36:44 regression or based on mean imputing or so. You can simply just call it data frame, identify or give it the column that has the missing data. And I know we'll do the rest for you So we'll save you,
36:57 like, we'll shorten the code. And I'm going to get a function. Why are we writing? If this is a solved problem, why are we creating the wheel? Yeah, yeah. One of the nice features about
37:06 Postfire is the data functions. Data functions are super powerful because it builds a lot of the UI for you. You don't have to do anything of that. You just put the code there. If you find it
37:18 somewhere else, or even the - we didn't talk about the copilot extensions. But I wanted to get into that, for sure, yeah. Maybe you can generate that for you, and then all you have to do is
37:28 define the parameters, and sometimes you don't even have to do that, and then define the output. What you want, you want a new data table, and you call them, and then you have your data right
37:39 there.
37:42 The action months has another thing. Yeah, that's a nice
37:48 thing that came up in recent version of the Spotfire, that you can automate things in a contained format. So you can also download, just like you do with data functions or mods, you can also
38:02 download predefined actions. And maybe you wanna write back to database, or maybe you want to automate some feature. For example, it's basically a wrapper around Iron Python, so many of the
38:14 things that you can do with Iron Python, you can do it now in an automated way. So you just, you have your action, you define, again, your parameters, when you maximize your window, you want
38:25 to also maximize the table, because if you have a big screen and you maximize, all the visualization will maximize, but not the data table. So maybe you want to go to that manual process of
38:36 resizing the columns, or you can optimize that with just a click. Nice. And for example, if you have, or like if you're looking at a rough job, and then you sew on a normally, and then you
38:49 wanna kind of like create a quick reporter that with a screenshot of that chart, maybe the name of the well, time, and everything, and then export as a PDF, and then share it with the team. You
38:60 can bundle all of that in an action mod, and then add that as like a simple button on the chart. That's pretty awesome. Just that, and we'll do all these steps for you. Yeah, yeah, that's nice.
39:09 These are like way off. Yeah, no, these are the UI like touches that I'm talking, the interface, the interaction touches that I'm talking about, and these are the things that like truly separate,
39:20 like an okay product from a great product, right? I mean, everyone does these, right, writing back and forth is changing resolution based off the screen and all this stuff. And so it's just like,
39:33 I love tools where we codify this, a lot of the simple repetitive things. So you don't have to do that every single time, but then you still have control to do everything else that you want. And
39:46 you're not just crammed into this low code box that you can't get out of I think that's where there's a lot of opinions on the low code stuff, but in my opinion, the best use of low code is
39:58 something like this, where it's standard, repeatable, everyone does it. We don't need to reinvent the wheel every single time, but you still have extensibility and control around everything else
40:09 that you want to do with it. Yeah, maybe you want to modify your own chain? Yeah. Because even like me, you mentioned running back to the database and I hadn't thought about that, but I mean,
40:16 I've forced it through an hour. data functions, but now like he said, like the action mods make that a lot cleaner because I mean, because I remember you've got a you guys both have a ton of
40:26 videos. So if you guys, you're listening to this and you want to, you know, see and hear more from these guys, they have a lot of really good content. Plug in plug it right. Yeah, as a doctor
40:35 spot fire spot fire, just look it up. It's on YouTube. And it's it's awesome. But I think you have one of those about like the writing active database, because is it even like, can you do it
40:44 like on a cell by cell basis? Like can you could you like you could go into like a cell edit a cell and write it back? I don't want to over promise them to deliver. But like, I know there was one
40:52 where I think it was something similar that way. Yeah, there was a there was a use case where you click on a cell and then you will open a little pop up window where you can change that value and
41:00 then write it back to the database. Or maybe you want to make a selection of, okay, these are outliers or I have to put a comment on these records, you hit a button and then right back into the
41:10 database and tags that with maybe your username or some additional comments for those records. back in the database, back in the source. Yeah, the thing is like with the different functions,
41:21 they're all like everything can be parameterized. Yeah. So, and then that parameterization can come from document property or like a highlight or a marking. It's like
41:33 up to you, how do you design your logic? So for example, if you wanna just click on something on a table and then like an action mod, I will write that back to like a database or this I mean, the
41:45 possibilities are endless. It depends on how you design it. Again, it's like, hey, we've parameterized some things to get nice and easy. I'm a big fan of customizing. Yeah, around and beyond.
41:57 It's like, that's the optimal thing. I feel like for most people, right? 'Cause in most of these scenarios, you have, I can use the word like citizen scientist, data scientist, right?
42:08 Engineers that aren't necessarily data scientist or people that aren't doing a lot of stuff Someone to define something for me. Right and so like I'm being it even just being able to understand what
42:18 it is like click and do it. Look at the formula That's good for most people right just like we're so ingrained in understanding all the all the bounding engines There's also something to to remember
42:30 Sometimes when you want to add an extension or some other blogging into your Solar tool you will have to go through these Corporate process and tailgates and everything Here it's just like a file.
42:46 It's like who's going to stop you for loading an Excel file into spot fire The same principle is with the custom visualizations who's going to prevent you to add a new visualization You don't have to
42:58 go to i3 to all that process or go to all those approvals and that was one of the The use case is one of our customers here. You are like but it's actually they didn't come to us they came that was
43:13 a story of one of course something that said
43:17 He saw what they were doing in the spot fire. He said, wow, that's amazing. How do I get that into my machine? Oh, yeah, here's the file. Just load it. I don't have to go to IT at it. No,
43:30 no, just right now you can start using it.
43:34 Yeah, 'cause even like say earlier, I brought up to like Streamlit or, you know, shiny or something like that. But like even those, especially in the bigger work, like you'd be lucky if they
43:42 let you install Python, or even the version of Python you want or do even like fight with, you know, to wrestle with those kind of things. So, and again, it's just, even those then you have to
43:51 be much more of a code for it, person, even, and then probably take even more time, even if you were good at the code to, you know, that time to value is always a huge, huge deal. Yeah, it's
44:00 designed to be a self-service and a critical visual artist on the platform. Looking at what the automation and parameterization, we recently added a couple of functionalities to our automation
44:13 services. So now they added this feature called for each, where you can basically loop over and create multiple, or like execute multiple tasks. So I've been using, before I joined Spotify, I've
44:27 been using the automation services a lot to generate PDF reports. And then the use case that I was really struggling with, if you have, let's say, a list of 100
44:43 employees, but those employees are divided into five groups. And then you wanna loop through all of these emails. And but, for example, the first group, you wanna send them report A, send group
44:53 report B, and then each report. So each report is different from
44:59 the other one. With the four each, you can basically do that with, I don't know, three steps. Basically just like, yeah, parameterize, you just like point it to the table that has all these
45:09 emails And then the second table is basically the report templates. five templates or so, and then that's it, and then
45:19 just like the power of parameterization.
45:23 I think it's another one of the things to highlight, and I think it's a blessing and a curse, because it can be really confusing for people that step into projects that they're not aware, but is
45:31 the data limiting options you have? Because I mean, you have filters, and you have filtering schemes, markings and different marking schemes, but then you have data living by expression, but all
45:42 these things, but
45:45 having all those levers, I mean, there's the possibilities are endless. So I mean, if you're intentional about how you build them out, I mean, because I think I'm one of the coolest, but
45:53 probably most dangerous one is create details visualization. And all of a sudden, people have like 80 different, you know, marking schemes in their tools, but like, I mean, makes a power user
46:03 or not a power user pretty dangerous quickly, where like, oh, I've got this bar chart, and then all of a sudden, I can see the details of that, or I can, you know, because, you know, if you
46:11 didn't know what you're doing, but you knew what a details visualization, you could create three. things and all of a sudden you could drill from this pie to these bars to this answer, you know,
46:18 in three clicks. Yeah, no, it's super. But again, like, but then, but understanding kind of how those all work. And well, I filtered here and didn't work on this page. Well, yes, a
46:26 different filtering scheme, but again, I think once, I think it is one of those, like, to your point, maybe it has something for everyone that wherever they're at, like. There's a new feature
46:37 also came in a couple of versions ago to, so you can visualize where your markings are being applied And maybe you have orphan markings because it's a lot of time, you have to figure out what
46:50 marking is these being used, and then you can just simply remove them and click them. You can know exactly where it's being applied. Oh, nice. Wanna hear more about the co-pilot? Yeah, as the
47:00 LLM guy. Yeah, so the co-pilot is basically an LLM agent. And it's a spot fire now? An extension, yeah. Yeah, you can install it as an SPK and extension.
47:14 in any DXP that I open. Yeah. Okay. And I do have to install it for each different project I open, or it's just - Just fill the one on the server. Once and then anytime I open it, it's available.
47:26 Yeah, it's gonna ask you if you wanna update the client when you connect to the server, and then you say yes, and then you
47:33 go ahead. Let it happen. It has a couple of, I think,
47:38 to the moment, I think it has six intents, like, say six main tasks You can basically ask a how-to question. So that will, for example, how to bring a mock spam fire. Oh, I'll give you all the
47:51 steps. And then as we talked, it will bring all the citations below, so where this information is coming from. You can ask, let's say, a data-related question, data-entering question, so, for
48:05 example, in table A, what was the, let's say, average gamma ray reading in this step.
48:14 from depth A to depth B, what was my average porosity thing? Or, for example, you can ask general questions like, what should I look for in my data? And it will give you like a whole summary for
48:29 your table, explaining all the trends, any, let's say, unexpected observations, any basic understanding from, so for example, I noticed that
48:42 the core palette is able to recognize, when I write gr, it was able to recognize that this is a gamma ray. Or for example, when I, one of the things that you can also ask is generating data
48:55 functions, which I think is like the most helpful one-hundred feature because yeah, a lot of people like, yeah, kind of like hesitant when it comes to writing data functions in Spotify. But
49:06 writing data functions through the core palette, basically it will add the data function within the like the data.
49:14 We'll not give you the code only. We'll add that for you. The only step left is for you just to map that to map the input and output, basically, to your real input and output. That's awesome.
49:24 And we'll give you the
49:28 function with all, they say, the commenting, all the description for the variables and everything. And uses the actual parameters from your data set and not just a generic domain. No, no, no,
49:39 yeah, basically, yeah
49:43 I was testing, for example,
49:46 write a data function to smooth the gr from this table and calculate VSH, just VSHL. And then, yeah, to my surprise, the copout was able to figure out the formula, the VSH, and then added that
50:02 and all the commenting. The function run, I mean, there are only, basically only two steps after the function is run. You need to trust the function. And then map the variables to like your real
50:12 variables
50:15 Then some testing also with kind of more complex use cases like reading data from a DLIS file. It's not a binary, it's not like an ASCII like the last file. And I was able to generate the function,
50:28 I would say 95, I just needed to change one or two lines, but the rest of the function was able to generate that and was able to extract
50:41 with a video card.
50:44 I think that's
50:48 funny because if you talked to someone that was really into the data science side or the ML side, they'd say, well, 95, it's not good enough, but then that's my argument. Well, exactly. But
51:01 that's my argument for these things is it's like, if I can get 95 of the way there in five minutes or less, the amount of output, the speed at which I'm going to be able to do things is just
51:11 tremendous. Yeah, I don't write PowerShell, but if I wanna do some simple thing on top of a file system and Windows, PowerShell's the best tool for the job. So just write it for me and I know
51:22 coding enough to read it like that fits, yeah, that looks right. And boom, it runs in two minutes, I did something that would have taken me 20, 30 minutes to hack away at, if not more. I mean,
51:32 that's the thing is it's like, people think that this is like some new kind of way of doing this, but it's like, I can't tell you how many stack overflow or them threads that I stole code from and
51:42 then modified to fit my use case before LLMs came out. It's the exact same thing. It is the exact same thing. Give me a starting point that is well understood and then I can modify it as, you know,
51:55 a pseudo user or whatever to make it work for my use case. And it's like there's all these instances across tech where that happens and it works really, really well, right? Like there's a trading
52:07 platform that I use for stocks Bitcoin and stuff called trading view if they haven't editor called pine editor. It's just like a little Python editor. And there's a marketplace and people put their
52:16 scripts out there. And I can go in there and copy the script and edit it to however I want. And it's great. It's a, I am curious though, you mentioned something. So it has, it obviously has
52:29 access to, you know, the underlying data and stuff. But it sounds like it also understands the data tables and the structures and the skin and everything. You can also generate, generate charts
52:40 for you. Do aggregations and all that. Let me explain charts to plain charts. So it's a plain, you can give it the name of the chart in a wall. Yeah, that's awesome. Like, tell you some worry
52:52 about trends in the charts, observations. And you can also, they have this feature called topics or modes, which is basically switching to a different index. So, let's say you are an oil and gas
53:07 company, and then you have.
53:10 index only for your leases and contracts and then another index maybe for like your reservoir engineering team and you want to ask something about or like something contract-related, you can simply
53:19 switch to that index, ask a question and then go back to the main index. Yeah, no, that's awesome. That's really cool. I think that's kind of the next big or one of the big next kind of
53:32 iterations on the language model front is it's a universal problem. Everyone wants to say, hey, language model, give me this aggregation and this sequel table or whatever table and language models
53:44 are language, not numbers. And so they can do math, they understand equations and they're getting better at it by the day. But it's such a weird thing because they understand SQL like they
53:55 understand how to write the code, you just have to connect. So I very much so believe that the next six, 12, 18 months, whatever it is, there will be kind of another groundbreaking step change
54:07 and how language models are able to interact with. traditional structured data, it's weird, right? Like, you've got this cool, sexy thing, but it's really mostly good at unstructured PDFs,
54:20 text data, whereas historically, we've always wanted really good structured, clean data, and so, but you can see where this, there's gonna be a convergence of them at some point, and then
54:30 that's gonna be crazier, you know? I've been saying, I went to the stabilization and oil and gas event here in New Zealand I've seen an interesting case about using LLM
54:49 to extract information from HSE documents also. And they were kind of like using that to, so whenever you wanna, let's say create a new HSE document or try to help you to like list of requirements
55:02 for an HSE job, what do you need to perform this job? Yeah, no, that's one of the things we're doing is, you know, you're paying people six figures to go right, the same 98 procedure for the,
55:18 you know, the procedure is literally 98 the exact same text, the exact same format, and that's what language models are really good at, the same repetitive thing. So it's like this natural
55:28 evolution of like, hey, why the hell are we paying these people to do this stuff that they don't even like doing, and they hate doing anyway? Hey, this makes a lot of sense, right? No, I'm
55:39 super bullish on the LLM stuff, and it makes me excited that it's slowly starting to seep into the data side of it, because you can really see like, and this is gonna be some powerful stuff if as
55:50 these things converge. And it's one of the, one of the questions that pretty much every single person asks on the enterprise side. They're like, we've got all this IoT data or this state of data.
56:01 We wanna be able to ask, you know, what's the, what can I do to fix my, to increase my pump hours, and I'm like, that is not gonna be a. going to give you a good answer today. That's not what
56:13 this is all that need to be indexed in it. Right. That's a mission. And also the other thing of it, it's domain knowledge should always be there. Yeah. And it should always be coupled, like at
56:24 least used to validate what you get out of the LLM. Sure. Like using LLM output without any domain, which is very dangerous. Yeah, 100. You can't. That's the thing. Use them, but don't give
56:40 them 100 of your trust, but verify 100. Yeah, because say I'm cannot go to jail, you think? No, we've talked about that before, though, just why AI progresses and if more things are automated
56:53 or cars drive themselves, like, then where does that liability? Yeah, that's it. Like, whereas, you know, who needs insurance, the person that's sitting in the car, or the company whose
57:03 model is driving the car, you know, like, it'll be an interesting legal landscape I really am not looking forward to the software world overtaking the auto industry, but it seems like that is kind
57:17 of inevitable at this point. I mean, I don't get it because of the whole right to own, right to repair, I think is the law or whatever, but like, how are you gonna charge me? I saw a Hummer
57:29 commercial. It was a really good commercial. And then right at the end, it was like, oh, free, I forgot what GM's blue cruise, I think is their GM's self-driving stuff For three years, and
57:41 then there was this big disclaimer at the bottom, and they get a free trial for three years, and then if you want it after three years, you gotta subscribe to it. And it's just like, man, this
57:49 is gonna be terrible. They're gonna make every, like, heated and cooled seats. I think Volvo did that. And that will be, it was BMW. It was BMW, yeah, it's like, yeah, you need to subscribe.
57:58 To P2C, it's ridiculous. Like, no, man, that's not how this works. It's my car. Exactly, it's like taking a basic, and then make it like subscribe to your microwave to work at home. No,
58:10 it's my microwave. I bought it. I own it. So before we get, you know, I got towards the end, I think I'm just kind of curious like whether it's, you know, again, you've both been using it as
58:20 customers and now you're obviously like, you know, where I've been a lot of part of a lot of projects at Spa Fire, but what's like a project that you worked on or that you've seen that's kind of
58:30 near and dear to your heart or something that was like maybe one of your favorite projects, you know, just kind of comes to you like, you're like, man, that was pretty cool. Cool use cases or
58:37 another one. Like, that's my big thing is we don't talk enough as an industry, but we also don't share like the cool, even you don't have to tell me how you did it, but just talk about what you
58:47 were doing, right? Like, we used the data from this in this way, which no one else is doing led to this. I walked on
58:57 a project
59:01 Well, they say the main. The theme of the project is using a technique called dynamic time warping. Dynamic time warping basically is a way to compare two sequences. So this sequence can be time
59:17 series or can be log data index by depth.
59:23 And in the use case was you have a bunch of wells already drilled And then you're trying to add or like you are currently drilling any well. And then you want to use the existing knowledge from these
59:40 wells to hopefully let's say increase your rate of penetration on this well, at least like to kind of like use the best practices from
59:52 the best. So the dynamic time warping what it does is it basically tells you according to a pre-selected parameters, for example.
1:00:03 like if you choose, let's say the gamma ray, or like any other indicator from this well,
1:00:10 and then you compare it with the existing wells. We'll tell you like which one is the most similar one
1:00:16 to use, and then you will kind of like, let's say,
1:00:21 you can consider that well as kind of like a twin to this well, so you can basically model the ROP using these like historical data, and then use that model to kind of like predict when you will hit
1:00:35 the reservoir on this existing well.
1:00:39 The nice thing is
1:00:43 with SpaFire
1:00:47 is we were provided with a lot of flexibility to kind of like quickly select, okay, I don't wanna let's say go by the camera, I wanna go maybe by another indicator, so you can quickly select that,
1:00:59 And you can also quickly - change your
1:01:04 clustering size. So for example, I wanna look at the, let's say, the creep, the three closest wells, like the four closest wells. And then we were also looking at things like, do these, so
1:01:16 for example, let's say in this area, we found out that the
1:01:22 flow rate is the, let's say, is the main factor that affects
1:01:27 the ROP But does that change as you like move in the rise of war? So coupling the mapping and all these data functions that you trigger when you select something. So made it very easy to kind of
1:01:40 like try different scenarios. Right. Yeah, this was very close to my heart. Yeah, that's a really cool use case and it makes a ton of sense, right? I think that's one of our biggest problems is
1:01:51 we do so much, but we don't, we're not very good at taking the learnings of the past applying them for actual things that we're doing in the future or today, right? It's like, we document these
1:02:05 things or we have all this data, but you know, half the time the data is junk or it's not structured or it needs to be cleaned or whatever. But it's like, once you do have it, look it, and then
1:02:16 that's the thing is that you are. Yeah. So I mean, with all these previous wells, you have like, uh, hundreds of DDRs. And these DDRs like definitely have a lot of, let's say issues that face
1:02:29 problems and then what did they do to solve this problem? So harvesting all this knowledge and then kind of employing that in your current operations. That's like a direct line to revenue cost
1:02:41 saving. Yeah. It's not just about a harvesting knowledge, but also data that can become knowledge because with all that information, then with the LMM principles, you can just turn that data into
1:02:55 knowledge and they say that data
1:02:58 is a new oil for the reason. Yeah, no, you can, I mean, that's the thing I think people new frame, especially operational data, right? Whether it's Sketa or Frank or drilling or fallback,
1:03:12 whatever. It's literally the system telling you what is happening. Whether that fits your hypothesis on what was going to happen or not is a whole nother story, but it's telling you something,
1:03:23 right? Like, I know Bobby knows exactly what I'm thinking about right now because of when we work together, the downhole gauge story. But, you know, this, Bobby and I have seen it. We did this
1:03:36 long-term well test. We had two independent gauges. They both, a client did not agree with the data. It was like a six-month downhole bottom pressure gauge. Bottom hole pressure gauge, they
1:03:46 didn't agree with the data, but we had two redundant gauges that we then sent off to be certified and recalibrated and make sure everything was good, completely cleared by the manufacturer that
1:03:55 everything was good. But because it didn't fit what they thought was supposed to be happening, It was bad data. the data is telling you what is happening and we need to change our hypothesis. And
1:04:05 that's always one of my big things like data science, like you have a hypothesis, you are testing it and if it
1:04:14 may not. Right, well, that's why oftentimes if you're doing real science, it probably won't fit your hypothesis. Right, I think it was one of the, I think it was Friedman, one of the
1:04:24 economists said something to the effect of, you've only just proven a way not to do it You've no ever proved something flat out. You just prove all the different ways that don't work. Exactly,
1:04:34 yeah, and that itself can be like, let's say a valid information. I remember my
1:04:40 supervisor at the Master's time, and when I started my research, and he said, Look, by the end of the research, what you're doing might not work. And that's still a valid information to provide,
1:04:56 say, the next one who will do the research So to avoid that, bye. That's one of the key things also spot for us to offer when you when you distinguish between analytics and reporting because
1:05:08 reporting will give you answers to your question you have a question you put the data and okay that's the answer but when you're doing that analysis just get all the data you start slicing and dicing
1:05:19 all the data you play with the x-axis y-axis let's see how at these these other one correlates with these other ones and then you'll see all these things and then you have even more questions right
1:05:31 questions that you never yeah let the data drive yeah they will drive yeah they will drive the questions yeah
1:05:39 and any any projects that we're kind of been near and dear to you or anything that comes to mind well i remember a project that was about getting a pi data okay i would say i like yeah i'll say i pi
1:05:54 and and they wanted to write back to the database and as easy as just selecting records, put in comments and that was a good project that I was involved with. The thing that you did with the map
1:06:11 charts where you bring a scan. Yeah, that was cool. That's another one, yeah. So the map charts, you not only can use TMS layers or different maps, different geographies, you can also put your
1:06:27 own images So this company was using these templates that are standard. They have like a curved scanned from a text book, like from a standard, from an API document or something, and they want to
1:06:39 kind of like project that project. So they want, because the map chart is like a scatter plot and they want to put those data points into that, overlay that image behind it. So, yeah, you
1:06:54 provide the data, You created a live function to, to. you define what
1:07:02 your, because your image have x, y coordinates, pixel coordinates, zero, zero. This is not an image laid over, or is this laid over an actual map, or is it? It's a map. Okay. And you use an
1:07:14 image as a map, and you have these pixel coordinates. So then you want to - The image is your map layer. Yeah, there's no terrain map or something Isn't that how you originally did some ternary
1:07:29 diagrams? People do that? Yeah, like that. Yeah, yeah. And then you can just put the
1:07:36 parameters in. You basically calibrate
1:07:40 your data with your image and then do the data points. That's a smart way to do that. Yeah, I think that's actually why I did one of the gun barrel implementations. We had a template to put behind
1:07:53 it, and then choosing if this is in this zone, and it goes at this, you know, x, y.
1:07:58 coordinate and like in spreading out across the just put it in a PowerPoint. Well, that's not without me now, but that's how they were building it out. But I mean, that, that gun barrel project
1:08:08 way we were, it was actually like a planning project before. Well, it was even created in areas, but then they were actually doing some stuff in Excel and putting in like a first and last take
1:08:18 points on all these things. And so they can move them around and then I should, our data function that was drawing the lines on the map using the SF, but then like once they got it where they
1:08:25 wanted it, it also created the gun barrel for them And then they would just grab that and put that into the, like the, um, into like their proposal, like, you know, for the, whenever we go to
1:08:35 like an oil and gas customers, we ask, how do you do the gun barrel? We wrote our own. That's it. Yeah. And they all do the exact same thing. Yeah. We're kind of like pushing. K let's, let's
1:08:46 release day and we have a lot of, we have a big responsibility because we see what our customers are doing, but they don't know what their competitors are doing Yeah, so we can just. create
1:08:57 something that will benefit everyone. Of course, with their approval. Yeah, not for sure. No, that's how. I think that's exactly how it should work. Or this, again, it never ceases to amaze
1:09:08 me. How quick these go. I'm doing real quick. Jump into the speed round. We'll do a couple real fast.
1:09:19 Favorite. Let's do favorite book or podcast that you either recently listened to or just really like in general. They can be energy data done there.
1:09:31 Yeah, for sure I've listened to the guest
1:09:35 podcast. Thanks. Appreciate it. Yeah. And then you need to bring the big night back to
1:09:45 you soon. We take early next year. We take the fall into fall and winter months off, mostly because a lot of people travel, they're hunting, there's just holidays, all of that stuff.
1:09:56 I've been reading recently a book called Designing Machine Learning
1:10:02 Systems, a y-by.
1:10:07 It's a very famous book.
1:10:10 It basically takes you through the steps of
1:10:15 designing machine learning systems on an entropy scale and all these precautions that you need to take and stuff like an eye. It doesn't have luck code There was another
1:10:29 book called
1:10:32 The Data Science from Business Perspective and it basically focuses on
1:10:39 why do you need to hold on the coding first before you start your machine learning project.
1:10:47 It basically tells you the coding is a very small part of the whole problem. You don't need to jump right into the book. The kind of designing the hypothesis and what you're gonna test and what the
1:10:59 outcomes desire are and everything. You need to kind of like understand first, what kind of answer you're looking for, and then maybe if you can want to fly like the - And the business impact and
1:11:11 the effort is gonna take to get there, I mean, 'cause at the end of the day, everything comes out of the money. I mean, with enough money, we could figure this problem out, but is it worth it?
1:11:20 Is it the two things that you've got one? For me, well, it's not all I got related. I have two favorite podcasts. One is The Exorcist Files. Interesting. That's nice. And the other one is
1:11:31 Teton Daily. Yeah, I got it. I got it, Teton Daily. And
1:11:35 favorite book is, now you see it. It's about, yeah, that's a great book here.
1:11:42 Nice. Favorite open source library?
1:11:46 Everyone loves this question. Well, some people either don't code don't use them at all or other people like. So overwhelming, I love them all. Yeah, it's like picking your favorite child. I
1:11:56 can, well, I can, yeah, I can mention like one library per, I guess, per task or like per area. So I'm able to flow
1:12:07 that. And then there's an open source platform called me. Yes, yeah, it's like a data engineering kind of. Yeah, it's like a pipeline. It's similar to like a similar to an air flow or a DAG
1:12:20 store or some of that I've heard very good things about it. How do you spell it? M-A-G-E. Is there any other things? Tommy Dang or some of that is like developer. I always see him on LinkedIn and
1:12:31 stuff. Yeah, I've heard very good things about it. I think he robs over. Nice. Yeah, I'm going to have to poke around in there. Yeah. For me, the three JS library, two organizations. Yeah.
1:12:43 That was the foundation of all the modern visualization like while we built on that and everything. And then another one that is probably built top of that one is echarts. Because it's an open
1:12:54 source library, and it has tons of visualizations that you can customize with basically minimal effort, yeah. Because D3 is, you gotta really get into the details. Yeah, yeah, yeah. I mean,
1:13:06 that's why it's super sensible. Of course, of course, yeah. All right, last one. Most importantly, favorite video or board game.
1:13:21 Okay, if it's Candyland, yeah, I mean we played Bluey last week, so you don't have to get to I like to play Or speak with my son. Yeah, I don't get no, that's perfect. Yeah, we love We play I
1:13:37 son loves to hunt and he loves to he loves the radio. So we've been playing PBR on the we
1:13:44 Yeah, I can imagine that looks like it's exactly so it's you're like controlling the rider with the stick with a really shitty old gyroscope Okay, we and it's just like he loves it. So you set
1:13:57 there and do it nice For me is penta. I don't know if it's a board game with with Beats or pebbles and you have to make rows of five you make a row five and you then you win He has to be straight and
1:14:11 there are other other ways to win, but that's like an Asian game And I've been playing that game with my wife when I met her a
1:14:22 1994. Yeah, he was my friend by then, but we've been playing since then. It's good. Good strategy. I know you said one more, but I have both of these guys here and they're in Houston. What's
1:14:33 your favorite restaurant in Houston area? Oh, that's a good one.
1:14:40 That we went to go on Capitol Grill. Capitol Grill, yeah. And there's, I was right above our, we're, we're off is right above Capitol Grill. Oh, okay. Yeah. And then there's, there's a
1:14:52 brisket place called Pinkertons. Hi, Pinkertons. Very good. Shout out to Grant Pinkerton. He's a, we, we cater Pinkertons at our events and stuff. Oh, okay. Yeah. Grains are great. Yeah.
1:15:01 Yeah. Yeah. Yeah. Yeah. Capitol Grill, I mean, I live in the KDA area and I like two restaurants in that area. One is Antoine's It's in Hamilton and the other one is a Brown and White. which
1:15:17 was the chef from Anthony, or one of the chefs right from Anthony's that opened up Pearl and Vine. Oh, I didn't know that part, okay. Makes a lot of sense then, right? Yeah, no, makes sense.
1:15:25 I'm an old kitty as well. And we started going, when we first moved out there, we'd go to Anthony's and then Pearl and Vine opened and then somebody told who, one of our friends or whatever, knew
1:15:37 Anthony or knows Anthony and was like, Oh yeah, he was one of the bean set chefs or sue chefs at Anthony's. And it's funny because the menus also have a lot of, like I love their greens. They
1:15:47 have really good color greens, Anthony's does. And Roland Vine has a very similar recipe, but they also have a great categio if anybody likes those, so.
1:15:59 Yeah, I love a categ. I say it wrong 'cause I can't roll my arse, but they're delicious.
1:16:06 Well, guys, thanks so much. This flew by. Yeah, thank you so much for inviting us. Yeah, thank you. Thanks so much for inviting us. Yeah, we definitely have you guys on. Where can people
1:16:13 find you? What's your YouTube channel? How do they, how do they get in touch if they don't? They can just look up Dr. Spottfire on YouTube. And you guys are on like the mic times, right? Yeah,
1:16:22 like yeah. Yeah, and everything related is for Spottfire is communityspottfirecom or justspottfirecom and there are tons of videos for learning, asking questions and engaging. Yeah, awesome.
1:16:34 Guys, appreciate it, Bobby. Thanks for being back, I'm glad you've made the track. Glad you've transitioned. On that note, guys, thanks for everything If you like what we're doing, please
1:16:34 give us a like. Make sure you subscribe and
1:16:49 we'll see y'all next time.
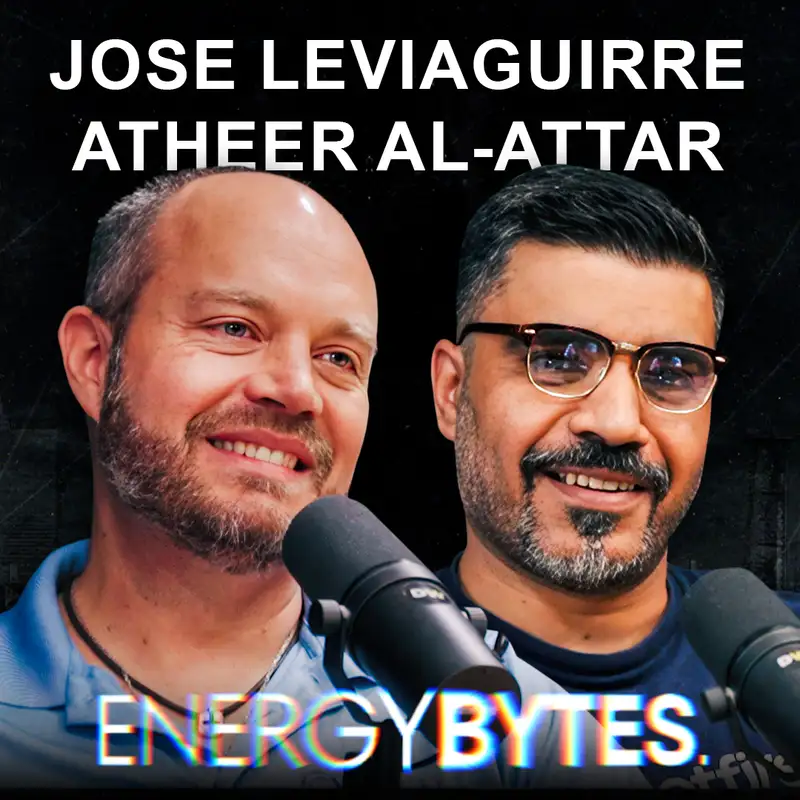