EP 55: Hatem Nasr from Softserve
0:00 Welcome back, everyone, to another, another week at Energy Bites here, Raddad in the house, John Kalfayan. Bobby's daughter went home sick today, so Bobby is out today, but today, I'm super
0:13 excited to to be here with us, him, Dr. Nasser from Softserve. Appreciate you coming. Pleasure. I'm excited to be with the wildcatters. Same, and we're happy to have you. Yes. We've kind of,
0:27 you know, I tried to minimize the conversation before, because that's always the good, juicy stuff. But, you know, tell people a little about what you're currently doing, what at Softserve,
0:38 and kind of get into some of that. And then we'll, like I said, we'll work backwards from there. You've got a really fun, cool kind of resume. And you've done a bunch of different stuff all over
0:47 the world. So I'm very sure I have been, I carry with Softserve is, you know, relatively young, a little bit over two years, and it is, And I'll tell you very quickly why I joined Softserve
1:03 because I've been working in the oil and gas. I spent the last 15 years dedicated my career pretty much to one subject, which is digital oil field. I mean, that has consumed me. And one area that
1:16 I have always had a tremendous amount of passion is how to build intelligence system, right? I mean,
1:24 so when COVID came, I was doing a lot of work in the Middle East, working with all the big operators, the big IOCs there. I spent probably 15 years in the Middle East, and then I stopped
1:35 traveling there because of COVID. So then I had several opportunities, and I wanted to join a company that has leadership and data science and AI. And so this is why I joined, and this is exactly
1:48 what I've been doing. So I've been focusing great deal. And it's not about, I'm not about the trend.
1:57 talk a little bit about generative AI. So that has been a huge focus for me this year. And there are a lot of reasons why I have great belief in the technology. There is a great promise, and I can
2:11 share with you all my reasoning and my rationale, but that's what we work on. And the company,
2:19 we work across multiple versions. So energy is one of them, right? Boiling gas is one of them, we have a big group or a manufacturing on healthcare on all sorts of areas. We have few areas we
2:34 focus on. We don't do everything. We work on subsurface, smart using AI to do subsurface data processing, seismic
2:45 data specifically. We work with a lot of big clients. We work a lot on intelligent production management, like production optimizations. Scata. More on the software side, how you can do
3:00 production optimization using AI, specifically for artificially lifted systems, electrical submersible palm, gas lift, those kinds of things. I've been focusing great deals since last year on the
3:14 area of methane data management since this is a huge issue for us in industry. We work a lot on worker safety and on the generative AI is applications across multiple areas, whether it's subsurface,
3:30 surface data processing, even including using gen AI to analyze images, videos, those kinds. Yeah, no, I'm right there with you. I'm super bullish on gen, gen AI for a lot of stuff. Um, I've
3:47 actually, it took me like, just thinking through a lot of this stuff. I feel like gen AI, like people think it's, yeah, it's new. And it's very interesting. But I'm coming to the conclusion
4:02 personally, at least, that it's almost a,
4:07 it's a derivative because it had to happen because we have so much data humans, like we have so much data, we can't do it. We've been using ML for that on the numerical side for a few, you know,
4:19 for a while now. And so now we've, okay, well, in order to process all this text data that we now have, this is the next iteration, this is kind of the next step that had to cut something had to
4:28 come, because the volume of data that we have now is insurmountable, basically, for most humans. And so admirable. And if you've been like, I have been in this business for a long time, just to
4:39 give you an idea, I myself have been involved in three major initiatives, knowledge management, for the last 25 years, you know, the work on knowledge management in our industry starting in 90s.
4:54 Shell, Schlumberger, Halibert, and they had major initiatives. The idea was to capture knowledge, but the technology then was very primitive. It was all about indexing, stuff, so that was very
5:06 disappointing. Actually I did work on some projects in the late '90s. I worked on a project in 2005, I was over the project with Shell, and I did a project when I was in the Middle East, also
5:18 around
5:21 2014, 2015 So frankly, all of them sort of, I hate to say it, but they ended up sort of going nowhere.
5:30 So now, Genevieve is giving us this incredible opportunity to do this thing right. Right. The other thing that I worry about, and I'm a, I'm a,
5:43 I read so much about statistics on our business. I'm a student of history If you look at the last 5th, 10 to 12 years. All right, started actually, if you go back to 2014, 2015, the number of
5:60 graduates and petroleum engineering and the last 10 years alone has gone down by 70. That's seven zero. All right. Yeah, no, I believe it. The enrollment in
6:15 petroleum engineering, this is in the United States, has gone down by 76, 77 Starling, we have a crisis that a lot of people don't realize. It's a knowledge-drained crisis. And one thing that
6:31 you said is absolutely spot on, the amount of data. You know, we are very unique as an industry. We don't throw away anything. Engineers don't like to throw stuff away. No, it's not only that,
6:42 it's mandated. If I'd really well today, 2024,
6:49 and I get some cores from that well, I have to keep those quarters forever. The logs have to be kept forever, the drilling data. So what are we doing with all of this, you know? So this amends
7:01 this amount of information that we're not leveraging.
7:05 I usually, I write an annual report that is usually published in January of every year that our annual sort of state of the union. And I usually takes me a while because it takes a lot of work.
7:19 Usually about 25, 30 pages long and I get a lot of help, our editorial staff and mark, they fix it up, make it look beautiful. But I'm picking the title. I started working on it last week and
7:33 it's gonna be, Can Jane AI raise the IQ of the oil and gas? And the answer is definitely yes. That's awesome. Now that is exactly, I mean, that's why we started Digital Walk. One of the reasons
7:46 we started Digital Walk Matters is because this whole great crew change thing is a very real thing, right? How do we capture this knowledge transfer from everyone that's retiring, leaving the
7:59 industry? Because we, like you just mentioned, the stats are shocking. Shocking. And, you know, I mean, there was already, that's the weird thing is it's almost, like it's happening again,
8:09 essentially, what happened between the 80s and the 2000s where there was basically next to no petroleum engineers because the market was so bad and everything, you know, peak oil, et cetera And
8:20 then you had a 20-year gap, then my generation came in in the mid-early 2000s, and there was nobody really between them, and now you're kind of seeing it almost play out again with this next
8:31 generation where it's like, yes, 77 that's crazy. We forget the amount of the number of people that we have laid off in 2014, 2015 bloodbath that happened in our industry. We lost hundreds of
8:47 thousands of people then COVID came, right?
8:53 just looking at 10 years. I'm not going back, I work at Bigger Hughes. I was in charge of advanced drilling technology. I had a big team there in the late '90s, early 2000s, and we had a crisis,
9:10 oil went sharply down, and I laid off almost half of my team. So we're constantly, and so how do we compensate for that? I'm not saying that Gen. Debyei is the universal panacea, but it is a
9:23 tremendous tool, and I hope that our industry is patient enough, 'cause we're not patient, right? We're not patient, and we risk averse, right? Very. That we are patient enough to cultivate
9:38 and invest in this technology, because we're not gonna see the results in six months or one year. Yeah. This is gonna be further down the line. Yeah, no, I think that's almost one of the traps
9:50 Absolutely falls into it's like we're one of the first people to look in half the time it seemingly invent the technology a lot of times Right, but then it takes another decade almost for people to
10:02 start actually adopting it But I think some of that is due to you know Just that technology hype cycle curve where it's like you have all these promises And then you go through the trough of despair
10:13 and then you come back somewhere in between the two is the reality of the situation And I feel like that's because I mean you saw what we've seen what happened with like the ML stuff Five ten years
10:22 ago, right where it initially came out everyone was hyping it and then everyone's like well You know is it is it really good? And then of course they're like oh well We need really good clean data
10:32 to do this and we don't have that we have data But it's not structured or you know worth of shit. So then they spend two years I tell people I tell people the story about about speaking
10:45 You're right on about the story of the lag between the time technology is developed and the time you see, I tell the story of George Mitchell in the '80s, when he was investing hundreds of millions
10:60 of dollars out of his own pocket. This man, I love him. He's a visionary in, you know, fracturing, right, in shale, right, and hydraulic fracturing. And people told him, You're an idiot for
11:14 a moron. And then when we started to really, you know, discover all of this in the 2000s. So this is a long-term game. And I believe, and the reason I was telling you about this IQ title, it
11:30 has a meaning because as an industry, we have to raise our intelligence level. People don't realize that if you don't do that, if you don't move very aggressively into these technologies, The
11:45 chance of being irrelevant. Very high and we saw that in our industry now. We are becoming more relevant because our industry Is making money and we know how to you know not to spend it, you know,
11:59 unwisely and all of the stuff but then when I look at Open AI Right speaking of a recent story. Yeah, you know, talk about open AI doing, you know raising funds Right. It raised about six seven
12:13 billion dollars at 150 billion
12:19 dollar market cap, you know, let's see how many oil companies have a 150 billion dollar market cap, right? Yeah. I mean, this is a company that's making less than, you know, five billion
12:30 dollars. So now people say, oh, this is nonsense. You know, this is hype. Well, heck, you know, look at NVIDIA, you know, it's over three trillion dollars. So it is not hype Let's get real.
12:45 Let's get on the bandwagon. Let's invest. Let's adopt. Let's take it beyond a curiosity. Yeah, no, it reminds me just when I remember when I first played with, I don't even remember which model
12:57 it was, it was either two or three, but when it first came out and I started playing with it and I was like, man, this reminds me very much so of the first time I used the internet. Like that,
13:10 you can see how this is going to completely change things for everyone. And so it was one of those things where it's like, man, this is cool, but the flip side of that is I, like hearing people
13:23 talk about it that aren't as up to speed on it or whatever, it reminds me of that. There's a Today Show clip of Katie Kirk and Matt Lauer and Al Roker talking about email from '95
13:37 or something '94, like early '90s, and like, what? They're just going to send a message. It goes to their magic inbox, and people are just going to communicate Like they were just mocking the
13:46 shit out of it, right? 25 years later, look what happened. Absolutely.
13:54 I remember I was right here not far from your place. We're very close partners with NVIDIA as a company. We do a lot of work. We are actually one of the premier partners of NVIDIA. So I was making
14:09 a presentation at their big event They had here in the western hotel. When Jensen Wong was making a speech about January VI. and all the things this was about a year and a half ago, he said it's
14:25 the iPhone moment for AI. It really hit me. It is the iPhone. I remember when
14:33 I first got my iPhone how I was like completely blown away. We are at this cross moment in our careers, in our lifetime, in our, it's a generational shift. and this is gonna change a lot of
14:50 things. I'm not sure that everybody understands it. We're looking at it, oh, this is another drink technology. This is another gizmo. This is another sensor. This is another down-hole gauge.
15:01 Oh, this is a new
15:05 ESP system or whatever. It's a little bit beyond that. Yeah, no, I agree. It's, well, 'cause it's so dynamic to all the things that you, you know what I mean? It's not like
15:16 when machine learning models first came out, you know, it was clear for me at least that a lot of, they had a lot of really interesting practical use cases for, you know, production data and like
15:27 we have these big data sets and these static assets and there's things going on all the time and we just need to optimize those things for whatever variables.
15:37 But I also think because they're so flexible, it also becomes hard for people initially to understand all the possibilities of it, right? I do think it's interesting. or like I've bitched for
15:50 years about how all of the important data in the oil field goes into a PDF somewhere to die instead of into a database, you know, it's all your post job reports. It's all these reports we've got.
16:00 We have our generative AI lab in our company. We have a big team dedicated just, you know, doing solutions across multiple. By the way, we work across all the different So we are very close
16:14 partners, we're premier partners with AWS, same thing with Microsoft. So we work with, you know, all of them with Google as well. So, so we, so I have, you know, work with my team to develop
16:29 applications for oil and gas. So we've used everything from JAT, GPT, Gemini to cloud to all of them. So we have a platform, our own platform called talk to data I was just curious, I said, I'm
16:45 gonna do something. So I had a bunch of documents, reports on electrical submersible pumps, failures. Right. So I went in, uploaded documents, it takes a while for the large language model to
17:02 digest all of this. But, you know, it was a half an hour. Yeah, it's not, it's not created. I didn't upload like a zillion documents. And literally I started after half an hour asking a
17:13 question. What about this? What about that? What if I have an overcurrent? What have a, you know, more overload? What, what happened? What should I do? And this thing was giving me some
17:22 serious good answers. You know, of course, I, I know a lot about a lot of stuff, but it told me some things that, oh, shoot, I forgot about that. You know, so, so things like this, what
17:36 are we doing? Like speaking about the PDF document, by the way, those documents, I upload a word PDF document. What are we doing with all of that? Exactly. Where's all of that going? Yeah,
17:46 you mean to tell me. And by the way, as an industry, we are among many industries. If you take all different industries, we have the highest age and the 55 to 65 bracket. We have the highest
18:04 percentage among most industries, right? Almost they estimate close to 15 to 18, almost some people estimate almost 20 out of within two years of retirement. So those people that know so much and
18:22 they left so much knowledge, I'm not sure, I mean, I love young people, they are brilliant, but knowledge matters, and experience matters. Well, especially, you know, I'm a perfect example,
18:36 right? I came in during the shale boom. Almost all of my experience the first three, four years was exclusively US onshore
18:45 shale then I started working, then I went to work for another frat company that. almost exclusively focused on vertical work, just conventional. I learned way more about frack in the nine months
18:56 that I worked for that company right before the 2015 downturn than I ever did the three, four years before that working on conventional's because, unconventional's, there's plenty of science that
19:08 happens in the office on the back end. But once it gets to the field level, it's almost like copy and paste the same design every single frack stage you do when you're in the field. And so it was
19:19 one of those things where it's like, man, you know, like for example, perfect example, on a conventional well, you would get your attitude out if you over flush by half of a barrel when you
19:29 flush on a frack job. I over flushed intentionally every single stage because the company man told me to on all of my show wells that I ever fractured. It was like completely different methodologies.
19:41 And had I never worked for that conventional company, I would have never known that And that's the scariest part for me. is that
19:51 we might have equal amount of times focusing on the unconventional stuff, but there's this whole other conventional world that a lot of people that came in in the after 2000 don't really, most of
20:03 them at least, don't have much experience with unless they worked internationally or offshore, right? And so it's like, that's a shit ton of knowledge that's also incredibly important, is not
20:15 going away anytime soon, that is going to be, a lot of it is going to be lost because of - A lot of it is going to be, believe me, and not only that,
20:25 let me tell you something that, when I talk to people, they ask me, it's a very simple and honest and, it's not a dumb question, why AI? What is the big deal, and why generative AI? And I go
20:42 back to, so I used to work for Baker Hughes, right? My job. I was senior director of advanced drilling technology, right? My job, my team, we were about 150 people, half of them are PhDs, to
20:59 design the latest and greatest drilling tool.
21:04 To come, so our business was to build MWDs, advanced drilling systems, all of this kind of stuffs. To build a new MWD, measure mobile drilling or logging while drilling tool A new brand new one.
21:19 We're talking about five to six years. This is to build it, to develop the technology. Then from there on, as a drilling service company, we had to roll it out. As a service company, you gotta
21:31 send it to Malaysia, you gotta send it to Abu Dhabi, you gotta send it to Nigeria. I mean, it's rolling around the technology. Look at the difference now. Do you have a piece of software, right?
21:46 we can develop it a lot faster. It's a lot cheaper, right? And it scales. And it scales, and you deploy it a lot faster. I can deploy systems all over the world with a click off a button, you
21:58 know? And then doesn't mean that we don't need your new drilling systems. If you have an ESP,
22:06 electrical submersible pumps, you know, probably the estimate is more than 55 of the world oil come from wells with the ESP. It is a hugely important system in our business.
22:22 It is like running a Ferrari in the ground, right? Hundreds of horsepower. And by the way, you cannot fix it. You cannot repair an ESP. You run it until it dies. Now, is it wise to use some
22:34 smart things to optimize, to prolong the life of this ESP, instead of, you know, having a run for three years, maybe you can run it for three and a half years
22:46 Imagine what advanced and intelligent software and AI can help you with. And by the way, I worked on a lot of companies. One day we did a study in a previous company I worked for. And we found out
23:01 that almost at any point in time, less know you,, producing be could that well a let's about talking I'm, mean I. optimal, Optimal. back revenues the also there's then but, side cost the about
23:05 talking were you 'Cause. Yeah Meaning. say gonna was I. production optimal with running were ESPs our of 60 than just throw
23:22 a number, 1, 000 barrels a day. Right. And it is only based on the efficiency of the pump based, well based on this, based on that, based on the models, but it's only producing 750. So you're
23:35 wasting 250 barrels a day, maybe by just changing the frequency from, you know, 58 hertz to 62 hertz. Right. this is where the technology comes in, which I mean, that specific thing, right?
23:49 Just that incremental production is literally the difference in being profitable or not on these unconventional levels in most cases. Let me tell you what worries me right now. I have a near-term to
24:01 mid-term worry about our industry, speaking of recent development. And I'm going to bring you to why our technology like AI and advanced and digitalization really are so critical.
24:15 Today, the break even costs of existing wells and the Permian somewhere around38 a barrel, 3839 a barrel. This is according to Dallas Federal Reserve.
24:29 The new wells is somewhere around65. I read a
24:34 miracle this week. They're going out. 65. In some of the shale wells is close to 70 Where is oil prices right now? It's not that far. But what worries me is. Because right now, the Saudis and
24:47 OPEC decided they are going to give up their production, you know,
24:55 hold on production. So they're going to pump more oil. And if oil prices go down to the60 range and below 60 in the 50s, we're going to start to see some pain. So the lesson of the day is you're
25:11 going to take care of your existing wells Because that's where the gold is, that's where most of your production is coming from. So how do you do it? Intelligent system, smart systems. I'm not
25:25 saying AI is going to give you all the solution, but definitely it's one of the recipe. So let's get into that. What are some use cases, some examples of some stuff that you guys have deployed?
25:37 We're doing on the deployment side, so we have two things We have things that we do. with clients we have things that we are so we invest a lot of money internally so on the deployments how we're
25:50 using extensively AI to do you know subsurface data process accelerate the process things that you take you days or weeks time is like seismic data seismic data we can do it in seconds one of our
26:05 hallmark cases that we have done in in the last recently the last year and a half year so one of our clients vital energy which has been investing tremendously in digitalization and AI we were talking
26:19 about ESPs earlier so we have deployed
26:23 AI you know data driven systems to help them monitor properly model you know the production and the goal is to give them recommendations to optimize our production. We have deployed on hundreds of
26:42 wells. I mean, they're achieving results that are astronomical in terms of increasing production. It's all AI-based, you know, where we model the well, we model the production, we do predictive
26:56 modeling, we say, okay, now you're well. There are two things that you can control the well
27:03 with. One is the frequency of the pump and the well-held pressure. So we give them recommendation We say change the frequency of the pump from point A to point B. So now we're not automating the
27:15 process of the control yet, back to the well, but the operators look at this and say, well, it makes sense. So next day, when they go to the well, they change the frequency. And by the way,
27:26 you can fully automate the process. So it's human in the loop for, I mean, that's how it must - But by the way, but they're going toward full automation. You gotta get them comfortable with them.
27:34 Absolutely So we work on those kind of applications a lot. We're investing a lot in generative AI. all right now and on methane but we have a big initiative called methane IQ for methane data
27:48 management. So methane as you know is a huge headache right for the industry. When a problem becomes also goes beyond a technical problem to be politicized but it's politicized with becoming social
28:04 problem you know becomes you start to as an industry you start to get a lot of bad wrap. So now there's a lot of new technologies that are coming to the scene before we used to use what we call
28:15 optical gas imaging cameras. We go around we do the survey now we're using satellites we're using drones so we're developing a lot of computer vision. Yeah technologies to analyze satellite data to
28:28 drone data and also to automate the reporting to the EPA so we can take your data wherever it is congregated and automate let's say for example I'm not going to get to to buzzwordy
28:42 Let's say the EPA wants a part W or quite OB report, and it's usually in a particular XML format. So we prepare, we automate all of that. So you don't even have to touch anything. Right, whereas
28:54 before you would have to hire analysts to, that was their full-time job, right? No, not only that, because once you capture the data and we get it from SCADA, we get it from OGI, and then you
29:04 have to transform it, there is another Excel sheet, and then there is this, and you have to go look at the meta file by hand, and you have to transform I mean, it was a nightmare. Yeah. The
29:14 area that I mentioned earlier is Gen AI. Gen AI for me is going beyond fascination. So for example, we've developed several use cases for Gen AI. We're talking about methane.
29:29 We have a Gen AI system that is connected to the EPA. All right? Life connection, right? We have an API connecting to the EPA.
29:42 413 documents, okay, 413, some of them are 2, 000 pages, believe me,
29:53 but yes, this is an upstream oil and gas, you know, all of our greenhouse gases. So we got all of these documents connected to this gen AI system, okay, and then the system checks like if we can
30:08 have a check every day or every week or every month for a new update for a new regulation, and then you can go to this gen AI and say, you know what, under the new rules, okay, am I allowed to
30:20 flare gas, right, and the system will go and tell you, here it is, none of this, do this, do that, and here's the document, here's specifically the page where you can get this information,
30:30 we're using also gen AI, for example, speaking of ESP, we were talking about ESP, for instance, let's just say, you have a diagnostic system that you're looking at, your motor current, your
30:44 motor temperature, your
30:47 input pressure, discharge pressure, variety, and this is going too high, this is going too low. So then you're sitting there and let's just say you're a junior engineer. I have no idea what the
30:58 hell is going on, right? And then you can click on a button, right there on the screen and say, Hey, I see these symptoms tell me what could be the problem, right? Now, this doesn't mean that
31:15 this is, you're gonna take this as a Bible, right? But this is a preliminary assessment and this is based on a bunch of reports. I was gonna say, this is, I wanna unpack this 'cause I think this
31:26 is where people, like the line between it being, like the average person sees something like this and they're like, Oh, it understands. Like they think they're getting in that AGI train of
31:39 thought almost, even if they don't know what AGI means, right? Like they're, they think this, these models can comprehend almost, right? Like because the results are so good, but the reality
31:48 of the situation is, if you have, that's why like I'm right there with you is with so, there are so many things that we do in our, and probably every industry, but in our industry specifically
32:00 that are like repe, 99 of it is repetitive and then 1 of it changes Absolutely. And so that's exactly what these models are perfect for because they're statistical recommendations based off what
32:13 they know. And what they know is only those procedure documents, it's going to be able to tell you historically, this is what it should say or it has happened. Yeah, I mean, this is exactly,
32:25 you said 99 is repetitive, the information is there, it looked at a whole bunch of reports, and this is what we're telling clients right now, Going back to what we were talking about earlier. all
32:38 this stuff that you have been storing in PDF files, your failure, reliability report, all of this. Where is that information? Are you leveraging it? Is it making your enterprise smarter? You
32:50 have to, because there is no question. This is your goal, right? Yeah, that's where most of the important data lives there. You got oil in the ground, knowledge is gold. That's your other
33:02 asset, that's your other reservoir. We have a reservoir of, Yeah, physical oil, physical, you know, and then we have a knowledge reservoir. So another area that we're working on in general,
33:16 and we have a fascinating demonstration. So in oil and gas companies, you know, we have cameras all over the place. And we use cameras near the wells, we have them in facilities, in refining,
33:30 in upstream, downstream, you know, midstream, not as much because pipelines are far. So, so here we do it for safety or people's safety making sure they're not doing stupid things that they're
33:45 you know, especially also sometimes in environmental events, the company I used to work for, we were paranoid about H2S problems. We've had people die in the past, so you want to know in case
34:00 there is an hydrogen sulfide, you know, a mission, are people at the is there anybody there out there that should not be there? We use it for security, right, for perimeter security, and you
34:13 know, we're a little bit paranoid, and you know, some parts of the world are extremely, you know, we've seen a lot of sabotage, and also for material delivery. Let's say you have a fracking job,
34:24 right? Did my truck arrive? Did this arrive? Am I, am I expecting so? So we connected, this is actually, we're working with one of our clients, we connected to a whole bunch of their cameras.
34:37 CCTV cameras. And then we asked Jenny, I did my truck arrive yesterday. All
34:44 right.
34:46 That is the job, you know,
34:49 you see any bulldozers moving in this area. You see any people moving around in this site. Have you seen anybody? You should not be there. And the system will tell you, here's what I saw, and
35:01 we'll show you the exact frames when it saw that information, you know. So you can train these tentative AI systems to do a whole lot of things, not only with text, but with video, with images.
35:14 I was going to say that's another point here, is that, you know, everyone think because they're large language models, everyone thinks of them as almost exclusively around written text. But with
35:26 how fast all of this is moved and continues to move, the multimodal stuff is just fascinating, right? Like, this is that this is the next This is the next horizon. This is, we're going way
35:36 beyond text. We are going to be using all sorts of forms of whether it's voice, it's text, it's images, it's videos. This guy is the limit limit. It's a very exciting arena, and I'm hoping,
35:54 and I'm going back to, I've seen in the industry sometimes, take too much of a conservative, oh, well, we're curious about Jenny, I let's think about it. Well, you've got to go beyond thinking
36:07 about it. Yeah, no, I mean, that's
36:11 always my advice. If you're interested in it, go play with it. Go use, go force yourself to use chat GBC or whichever one is your favorite for a week straight, for as many tasks as you possibly
36:24 can. All the way down to like the simplest, I need to cook dinner tonight. So what am I going to cook for dinner? Well, take a picture of what's in your pantry to make your recipe and it can do
36:34 that. I mean, there's, there's, even like, 'cause before, you know, the knowledge transfer thing is a big thing on our end as well. And I think this is a home run for JNAI stuff.
36:47 But you think about, you know, this
36:50 historically in the oil field, the way that you train people is you went and you rotated them. They went and worked drilling for six months. They went and worked completions for six months. They
36:59 went and worked production our year, however long it is, right? That's right But how does that work when these cycles get shorter, the industry cycles get shorter? And we have this faster up and
37:09 down cycle. Like you can't scale companies over years by hiring and training. You have to have people that can sit down and hit the ground running, especially with a lot of private equity, oil and
37:23 gas companies and stuff like that. And so like these language models are perfect examples of that and using the multi-modal, I think people don't think about the fact AR meetings, for example,
37:34 this is a perfect use case. Every major company does AR meetings after pretty much everything they do. No one records them. They have a note taker, but no one records them. I don't know why, but
37:45 even historically, if they did, you'd still have to send that off and have someone transcribe it and do all this stuff. Now, if you just were, you take out your phone, set it up in the room. It
37:55 doesn't even have to have the video, just the audio, and then you can feed it into a whisper. There's a number of them now that will transcribe it incredibly accurately, very cheaply. Co-pilot
38:05 does it beautifully. And then it goes into your vector database, and now it's there forever. Yeah, if you're on team, you can do with co-pilot, you don't have to record anything anymore, you
38:14 can transcribe the whole thing. But listen, I mentioned to you my concerns and yes, and
38:26 this is what happened, we have a tendency for somebody like me for a long time, There's always this tendency to be a little bit euphoric. Like I say, for example, right now we're producing 132
38:37 million barrels of fantastic record level. And by the way, we've achieved that through incredible advancement and drilling, no question about it. We've achieved it through also advancement in
38:55 hydraulic fracturing, all of this. But let me tell you The reason is there are 132 million barrels a day. We're not drilling as much. The number of rigs is significantly down a few years ago. So
39:09 the question that people ask me is like, how is that possible? It's all from efficiency and drilling. I said, no, well, in COVID, we'll drill a lot of wells which we did not complete. All the
39:19 ducts. So those are coming online. But the reason I'm concerned and we need to push technology on the digital side, on the AI,
39:28 on the generative AI.
39:31 because we have a lot of problems, you know, our, our, you look at the Permian,
39:37 we have several issues that people need to pay attention to. When you drill a well, your peak production, right? You know, a few months later, you know, you reach a peak production and then you
39:49 have the production. If you look at the production, the client curve,
39:53 and the last two and a half years, has gone down by almost 10, right? That peak Yeah. So that's telling us something. We are drilling longer, more lateral wells, and we're not getting as much
40:07 out of - A sparrows per foot. So also the enhanced recovery from our reservoir. And by the way, we still had almost a little bit, less than 40 in terms of reservoir recovery. I mean, that's on
40:22 the high side too, right? That's on the high side. Your people are arguing a bunch lower than that. That's on the high side. You have to go to some places in the Middle East to find out better
40:29 recovery, which is somewhat I work. So you have to, and then the last thing I wanna mention, in terms of new discoveries, we're at eight decade low. The biggest discovery in the last 10 years,
40:46 12 years, has most of it has come largest one in Guiana, almost 12 to 14 of the new discovery from one country. So we need to understand that things are becoming more challenged The honeymoon is
41:01 not going to be forever. Right. So when we talk about technology, we talk about it as a technologist. It's my life, my life dedication, that technology has
41:15 to prepare you for the challenges of the future. Yeah. And we have to invest in those kinds of things. Again, it's not only about AI or generative AI. There's a whole range of things we need.
41:27 Right And that's why I've always been very critical. me working in a senior role in some of the oil companies, is that we got to invest a little bit more in RD. As an industry, we don't invest
41:40 enough,
41:42 compared to pharmaceutical, of course. It's a
41:47 weird industry because innovation, in my experience, innovation comes when prices are low, which is when no one has the budgets to innovate. And then when prices are too. There's a sweet spot.
42:00 The last, I don't know, five, seven years have been in
42:05 this60 to90 range. If you get too high above that, then the mentality changes. The mentality when I came out of school directly into the field was get it out of the ground as quickly as we can
42:16 before prices go back down. If you boiled it all the way down, that's ultimately basically what it was. Never forget my first first year in the field. I vividly remember a job sitting in the van,
42:31 we were shut down because we had screened out and there's like six, eight, 10 sand trucks sitting there, part charging me to merge, to merge just because no one informed the sand haulers that we
42:44 were shut down because we've, and so now you have all these compounding things that happen, but no one gave a shit, even though they were charging us20, 000 a day or whatever the hell it was, no
42:53 one cared. But then when prices get back below a certain point, then everyone hyper focuses on how do we do more with less, because we got to lower our break even, our lifting costs and all the
43:02 stuff. But then there's no budget normally for it. It's such a weird, this is what I was mentioning earlier. I do see in, it's not going to be like this forever, but I do see a period where
43:15 maybe it might happen 2025 because of the OPEC increase in production that might hit the oil prices. So I have to do better in getting our margins better. How do you improve your margins? is
43:31 because you're producing things a lot more efficiently. How do you produce more efficiently? You have to deploy technology.
43:38 You have to do smart, you have to do it in a smarter way. It's not like it's a crazy grand plan either, right? Like that's literally the point of technology is to make things more efficient. It's
43:49 not a grand plan. It's about the
43:53 state of readiness. So we have a job to do And I do a lot of, you know, as much as I focus on technology and solutions and building stuff, my job is a lot of times as I preach about these things.
44:09 And some people don't get it. Like what the hell is this guy talking about? I'll tell them, I've been there. Exactly what you said. All of a sudden when the price goes on, it was scrambling,
44:18 you know, and then we started laying off people again, right? Then we lay everybody off. Then we need to do more with less. Then we need to innovate, but we don't have budgets to innovate with.
44:27 So then how the hell are we supposed to innovate?
44:32 Yeah, the industry has done well, and I give them a lot of credit, of course, in terms of managing capital expenditures, being more responsible than how they spend the money. I don't think
44:44 they're going crazy on the drilling side, but we have to get to a point. I think this consolidation, by the way, and the industry is going to
44:56 help, kind of do a lot of restraining The big companies don't want to go crazy with production. Because, ultimately, it's not good for anybody. Yeah, the bigger the company, the longer the game,
45:07 they're trying to play, right? So it's a lot easier for them to. It's, you know, I mean, a lot of people, when they ask me, say, Well, now that they, you know, korako Phillips, it's like
45:16 with Shena XO Mobile, the chevron, you know, this, oil production. I said, No, precisely because of the op. Mm-hmm.
45:27 Right, they're buying the assets. Right, they're buying the resurrecting of the reserves. They're not thinking about, oh, we're gonna do this acquisition, we're gonna increase production. As a
45:38 matter of fact, if you combine two productions of the two companies, you know, Chevron has, right? And the future might be less than100, yeah, 100. One plus one is not gonna be two. Might be
45:50 a little bit less than two. Yeah, no, I think that's another weird part about our industry, right, is that
45:57 you get to a point at a company's, at least at an operator's life cycle, where you either have to buy or
46:06 you have to set, like you can't, you always have to be buying to grow if you're an oil and gas company, because it's a depreciating asset, or a depleting asset, essentially, right? So once you
46:14 buy it, the minute you start producing, it's depleting. And so if you're trying to grow revenue and company value, that's part of your asset so you just buy more of it. Yeah, I mean, some of
46:26 these. Positions are precisely for for a particular reason, you know, like I have a lot of You know offshore Guyana Suriname all of these discoveries that are happening there This is this is a hot
46:39 part of the world used to be it used to be West Africa Brazil is still pretty active. Yeah, Gulf, Mexico. It's kind of cooling down a little bit a US is still has a lot of oil you know It is going
46:55 to become more challenging to produce it. It's not an easy thing It's becoming more and more difficult in some some parts, but but every
47:07 And here's the thing, you know, the world is gonna need more oil. Yeah, you know, I mean You know, I mean OPEC and the I a they have differences, but but I definitely think and You know now
47:19 we're about a hundred and one hundred two million barrels a day We soon gonna need a hundred ten million Now to get 8 million barrels more, you know, I work, you know, I spent several years
47:33 working at Kuwait oil company
47:37 before I joined. So
47:42 it is one of the most prolific parts of the world, Kuwait, Saudi Arabia, Iraq, you know, you know,
47:51 we started to see alarming threats You know, there are some areas where water production was increasing in six months, you know, from having 15 water cut to 30 water cut. This is in some of the
48:09 best reservoirs in the world, you know, so you start to see production challenges even in some of the best places in the world. So if it happens there, you know, it's going to happen here Yeah,
48:23 well, that's it. interesting to think about in, you know, the terms that you just outlined, right? Where it's not only, okay, you're, you're producing now 15 less oil. So revenues should be
48:35 going down because of that. But then you also have the incremental new cost of getting rid of that water as well. So it hits it all the way a lot of, a lot of people don't realize it on our
48:45 business. We produce water, you know, water that no one wants. And you can't really do much. No, no one wants. We produce a lot of water, you know, so, I mean, you know, some, some places
48:54 you're producing 80, 90 water cut, you know, so, so, yeah. And then, and then you're, you got 10 oil, you know, so, so, yeah. And then you have to do something with the water. Yeah.
49:04 Well, yeah, people, again, people don't think about it just from like, uh, a, uh.
49:12 A revenue perspective, right? So okay, I increase my water cut, which means I'm producing less oil or I have to produce more volume of fluid to produce the same amount of oil. Yes So my revenue
49:22 goes down, but then because I'm also producing more water. the cost to produce those same barrels of oil goes up. So now my margins are squeezing the water. And water, that water is a bad news
49:34 because that water is, you have to take care of it, you have to clean it up. It has environmental consequences.
49:41 There has been many, many incidents where some serious penalties have been levied because of spilled produced water. Yeah, well now you've got to be careful here with the earthquakes, about how
49:52 we're going to - Talking, I was talking to a friend of mine who,
49:57 you know, amazing, you know, satellite technology company called Sederlytics. So they do a lot of emission monitoring, right? But also they monitor other things. They monitor mutation, they
50:09 monitor water, you know. But he was telling me, this was shocked me. I was talking to the CEO of one of the largest insurance companies in the country And he told him, he said, said, you're
50:23 worried about methane, you know? methane is a problem. But he said, insurance companies, we are a lot more concerned about
50:33 produce water incidents than methane, because of the environmental effect of it. So yes, we have to pay. So water is not good news. It's not people think it's drinking water No, yeah, that's
50:49 the, I know that's such a weird element of our industry is, oh, we produce water, but it's water that is terrible.
50:59 I did want to touch on kind of your only because Bobby and I both worked in the kind of IoT space as well. I wanted to touch on some of your IoT experience and just how that has kind of evolved over
51:12 the decade. It has evolved. It has evolved a lot I spent many, so I think I told you, so I dedicated a good deal of my career day on digital oil fields.
51:25 Initiatives I worked on, some of the projects were massive, massive projects. And what I mean by massive, I mean, I'll give you a perspective. I was in charge of three major initiative projects
51:40 in Kuwait. The budget that we set aside for 10 years was in excess of300 million, so it was massive. And there, we were talking about Complete Tree Hall. Starting through the well, all the
51:54 sensors or the
51:56 IOTs, on the surface, on the subsurface, but on the surface side, having sensors, having wireless sensors, in many cases, we deployed wireless sensors. So the push for wireless is big. Yeah.
52:10 So as you know, you've been in the IOT business, so well, you
52:17 could put
52:19 a pressure sensor. Pressure, yeah. And by the way, for your information, Oh
52:26 In Texas, the cost of putting the sensors, right? Installing the sensors, running the cable, running the cable is more expensive than the sensor, right? Because it's a nightmare. And by the
52:39 way, trucks run over the cable and all sorts of mess. So we've been pushing extensively and I've been pushing extensively for wireless sensors, right? Wireless IoT's to transmit the data That's
52:53 evolving really rapidly. Now, what's happening is
53:00 we've seen the technology evolve, where you have the point to most of the point, kind of bringing it into a gateway, right? And from the gateway, you have your telemetry, your long-haul
53:12 telemetry. What I'm really excited about, and I started some projects there
53:19 when I was in Kuwait, Because this issue of, you know, getting the data long haul back. right? And even here, because you have to pay to transmit the data, right? So you cannot be putting
53:32 sometimes
53:34 SIM, you know, communication, you know, whether satellite or using the cellular service on every world. So why don't we congregate a bunch of wells? Okay, the sensors sending their data into
53:47 one hub and then transmitting it. But the challenge was a lot of the early generation IOTs, while the sensors though, they did only a few meters, right? So I got a really excited when I
54:00 discovered Laura. I was gonna ask you about Laura Wayne. I think Laura Wayne is a game changer. As a true game changer, because, and so let me give you an example of how we did it. So we had
54:16 several wells, you know, within several miles, you know, dispersed. And then what we do is we wireless sensor, lower sensors to one gateway. So now I'm using one gateway, all right. Let's
54:32 just give you just run a number for 10 wells. Right, instead of one per well. Instead of one per well. And then I had to set up now. And that project we were using a broadband telemetry back to
54:47 the main control center. But those broadband, you know, they cost a lot of money to install and this and that. Or you could be using maybe the cellular communication, could maybe using ATT or
55:00 Verizon or whatever. So it's a lot cheaper, it's a lot more cost effective. And Laura when is extremely reliable. And it transmits for miles too. It transmits for miles, you could set it up. So
55:13 that I think is the next in terms of communication in terms of sensors, IOTs On the other hand. you were asking me about IoT in terms of intelligence at the edge, I think
55:28 in some areas, in some applications, we definitely need the edge, you know, because right now the reason we transmit data every 10, 15 minutes from many wells or locations is because of the
55:42 communication cost, right? But if you can do things at the edge, right, we're working with our partners in video, just to give you an idea. So we are very close to relationships. So now in
55:55 Vidya, they have a Jetson, which is a tiny GPU, right? This thing used to be probably not a long time ago. It was
56:05 a10, 000, you know, a thing. Now it's about1, 500. Yeah, they're cheap.
56:11 Imagine what you can do with a Jetson GPU at the edge processing your data, where you don't have to transmit all the you can do maybe smart control, you know, so the technology is evolving. Now
56:25 it's people like, for example, I was in a meeting yesterday talking about some edge technology with our, but we at software, we prepared a package offering with NVIDIA, you know, an edge
56:38 offering, an IoT edge offering, right, for and specifically we're doing it for analysis of video cameras, right. So you want, you want to deploy it, very cheap, very cost effective, you know,
56:52 video is a bet, one of the best use cases. Yeah, because video, video, you're crushing video, you're not big data. Yeah, you have a lot of pixels. Well, and the other interesting thing, so
57:04 before I worked at DW, I worked for a edge startup for a year. So I love talking edge because I think it's a really fascinating space, but
57:17 one of the
57:20 I'm just completely blanked on my thought, but the
57:24 video, the really fascinating thing we were taught, we had conversations with Walmart, right? And they were talking about, we were talking about edge appointments with them. And they said
57:34 something along the lines of, we've got however many cameras per store and they've got thousands of stores all over the world, right? But then they said, you know, give or take 10 to 12 hours per
57:45 day of that camera footage data is basically nothing It's empty aisles and it's like, historically they would be sending that data to a server somewhere for it to do what? Literally nothing because
57:57 there's nothing of value that can come from that half of the data set. The other half of the data set is very important, right? There's people walking around, they want it for insurance and
58:06 liability reasons, they want it for all kinds of stuff, understanding how people walk through the stores and the flow through the store and all this stuff. But just from that perspective of like,
58:14 literally I can cut out half of the data, half of my data transfer costs, Half of my data storage costs. after my compute cause like it just goes away. It's, so you think like, okay, let's just
58:27 say, you know, you have hundreds of thousands of cameras. Oh, I'm going to transmit all this video. It's going to sit on a service. Are you serious? Right, who's going to do it? Who's going
58:36 to do it? What are they going to do it? And by the way, storing video, a lot of people don't understand, you know, because I've done, you know, work a lot of image and image processing
58:45 computer vision You have an image, you know, 512 by 512 pixels. By the way, this is like a really low resolution trap, you know, versus, you know, mega pixels, right? Where each pixel in a
58:59 color video is, you know, could be, you know, 8-bit, 64-bit. I mean, you're talking about a lot of bits, man, that all of a sudden, and by the way, cameras, they capture a lot of them, 30
59:13 frames a second, right? Or some of them even more Yeah, some of them even more frames per second. So imagine now you have to transmit all this video versus processing it right there on the edge,
59:26 extracting whatever information, sending only the results back, and then people can look at it. We are working as a
59:36 departure from oil and gas application. We have a huge project in, believe it or not, an Ecuador with NVIDIA as a partner. So in Ecuador, they had a lot of security pro The country has been
59:49 facing a lot of security, kidnapping, this, that, all sorts of things. And they had cameras already all over the city and we installed more cameras. So now we have this very intelligent system
1:00:02 that analyzes the videos. So they say, there is a kidnapping that might be happening. Literally the system will tell you will give you a warning or there is an incident, there is that, and they
1:00:13 will analyze people's behavior as well It's not only about getting, you know, cars, you know, license blade and that kind of stuff. So we're deploying these things at a large scale and it's
1:00:25 really proving and now they started with a key to the capital now, again, now they're going to roll it out across the whole country. Man, that's what is that maybe minority report? But yeah,
1:00:36 it's crazy. I was, I was exactly. Well, there's, I remember those minority reports. Yes. With, uh,
1:00:44 with, uh, the tonkers. Tom Cruise. Yeah. Yes. Uh, I remember years ago But somebody, like a family friend, told me about this company to look at, just stock trading stuff, and it's called
1:00:54 Shot Spotter, and all of it, all of it is, I won't oversimplify it, but it's similar in a lot of senses about this, but it's around, uh, they basically deploy acoustic arrays and they can
1:01:07 identify gunshots from ambient sound for miles or whatever. So they started deploying these in cities, tying it back to the police station so that if one of those triggers it can alert someone to go.
1:01:18 go to it. But
1:01:21 you, when I first heard that, I was like, Oh, man, that's kind of getting creepy. Just like, yes, I want, I don't want people, you know, I want cops deployed where people are there gunfire.
1:01:29 But then you layer things like this on top of it. And I actually, a fun recent news article that we could talk about is someone I saw video yesterday, someone has now taken the new meta glasses,
1:01:41 the ones that Zuckerberg has taken that and then added a basically a reverse image lookup. So like, I could walk up to you, it will identify it will use computer vision, find your face, and then
1:01:57 take a picture of your face and go reverse image lookup your face to see if it can find who you are all in the display. And so this guy was just walking up to strangers and being like, are you so
1:02:07 and so doctor at this? And it's like, man, like that could be really cool. But that's also super creepy That is cute for your hair. Yeah, and of course it's like, you can only imagine what.
1:02:18 what all the governments have that we don't there is obviously, you know, like when we're talking about video analysis with AI, Jenny, I, there's a lot of caution with that because there's a lot
1:02:30 of privacy issues. And the case of the project we're doing in Ecuador, it's the country is reeling from all this, this, this violence. And so they figured, you know, we got to do something now,
1:02:44 probably something like this may not work in the United States, then you're really, you know, nation of people's rights, et cetera. But there are some things, even when we are deploying computer
1:02:56 vision for worker safety, there is a lot of, you know, so, like, for example, only can do, you know, like the, you know, are they wearing the gear, wearing the helmet, you know, the PPE.
1:03:07 You know, the PPE. So you don't go into like, who is doing, and because you got to get really sensitive, because people may say you, know, Hey, are you monitoring me, are you following me?
1:03:17 Well, but like, the flip side of that too, and I thought, I didn't think about this until, you know, after I left the field and got married and had kids and stuff, but it's like, shit, man,
1:03:27 outside of my cell phone and me literally writing my name down on a piece of paper on location that could literally burn in a fire if something happened. You could, you could. No one would have any
1:03:36 idea whether I was on that location or not. Literally, no one would know. I was supposed to be, but how would they know if that piece of paper was gone, right? And it's like, there's all these
1:03:45 additional things And I, as someone who loves technology, I think it is effectively a corporate responsibility of oil and gas companies, both operator and service side, two leverage technologies
1:03:60 to keep their employees as safe as possible because - Hey, I have been, I am a living profile because, like I said, in Kuwait, on some of the wells, we had an H2S problem and it's a silent
1:04:14 killer I mean, that thing will. kill you and we're not talking about it in hours, in minutes. So we had a responsibility. That's why we deployed a lot of cameras, make sure that in case there is
1:04:27 an incident, immediately there is people out of out of there and you deploy your, you know, your, you know, your ambulances, your, your support team and all of this to get them out of there.
1:04:40 Yeah. So yeah, I mean, this is, it's not even an option for us. If you, if you don't work in offshore environment, it's, it's very, very serious business. On short environments, I have, I
1:04:51 have seen some people doing stupid things and getting hurt. Well, it's, it's just crazy to me because it's like, okay, you're required, you know, when I first started,
1:04:60 you had to wear FRs, you had to wear a hard hat and safety glasses and steel toes.
1:05:07 Shortly before that, you didn't even have to wear FRs, right? You just needed a hard hat, steel toes and glasses. And so it's one of those where it's like, okay, you require all these.
1:05:20 safety precautions in case something happens, but then why aren't we actively making sure that people aren't putting themselves knowingly or unknowingly in unsafe situations for computer vision and
1:05:33 things like that. It is the biggest, it is the single biggest nightmare of a company, oil company, I know working as a company Or somebody, one of your people killed in the field. That is,
1:05:51 you don't understand the impact. So that's why if you work for, let's say, every company that I work, first thing, when you go to a meeting and you don't see that in any other industry, right?
1:06:01 Right, the safety moment. The safety moment, here are your exits, here's this, here's that, they tell you everything or everything is in case this, in case that. You don't see that in too many
1:06:12 industries I'm sorry, I didn't mean to say we're the only industry. you don't go to a banking, finance, you know, meeting and you see that. So we do care about, HSC is something we take very
1:06:25 serious. Yeah, no, I mean, it's, again, it's one of those where, in my opinion, there really shouldn't be, like we should leverage technology to eliminate onsite, injuries, fatalities, all
1:06:40 of this stuff, because a lot of the time it is preventable if the tech is in place And so I think that's a, yeah, yet another one. And that's also one of those that isn't like, well, if we
1:06:52 implement this and it's not perfect,
1:06:56 the world's going to end kind of thing, right? Like that's always the thing. Yeah, I agree with you. I mean, of course, everything we wanna perfect it has to be work 100. No, if you can
1:07:07 deploy it and whatever imperfection it has, it's not hurtful. It's not harmful. Exactly. It's okay, and they get better as all things do over time. Even if it is 80 of the time accurate, then
1:07:21 20 is not hurting anybody. Use a deploy it, leverage it. Now that's when I was working for the Edge Company, Hivesault, one of the use cases for computer vision that I would put in there was, I
1:07:36 don't remember which university did the study, but it was the, they had a bunch of doctors analyze mammograms, then they had an AI model analyze the mammograms independently, then they had the
1:07:48 doctors analyze them with in conjunction, or either before or after, I don't remember the AI model. And the doctors by themselves identified it, whatever it was, 60, 70 of the time. The model
1:07:59 by itself identified it roughly the same. Together, they identified it like 80 to 90, 85, 90. And just that, you know, it's not perfect, again,
1:08:12 but that Delta that came up was millions of people that got saved. That Delta is a big deal. Right, like they saved these people's lives. That trivial stuff. It's not trivial at all. So yeah,
1:08:21 when people say 80, they're like, Oh, well, I don't know. If something did 80 of the task that you're currently dreading doing right now, you would be very happy. That's exactly what I tell
1:08:31 people about the gen stuff, right? Like it's not always going to be perfect, but it's a hell of a lot faster than doing a lot of things by hand. And by the way, by the way, I tell them the same
1:08:40 thing those few times where it's going to screw up, it's not going to kill anybody. Yeah, yeah. Well, and that's the thing. That's one of the reasons I want to talk, like I try and talk on the
1:08:50 show about it as much as possible so that people, 'cause if you don't understand it, then you can make that mistake. If you don't understand how these things work and that it's not always going to
1:08:58 be exactly perfect or that you should take it with a grain of salt and you should make sure you double check. That's the case in a lot of the, forget about Jenny I Any of it goes back to my years
1:09:10 and drilling. Like, for example, my team, we developed some of the latest and greatest measurement wild drilling tool. All these sensors in resistivity and porosity and this and that, nuclear,
1:09:24 even we did magnetic resonance imagery. I remember I had a project developing to integrate magnetic resonance, which is like an MRI for the ground in one of the tools. And somebody says, well,
1:09:37 it's telling me to flow Well, it's not going to be super accurate as
1:09:46 I said. If it gives me 70 accuracy, 70, I don't want even 80 or 90. It's telling me a lot more because that's something I see that I didn't see before. Exactly. So we don't have to be always
1:09:59 spot on, all right? The same thing when we were developing a resistivity tool Oh, well, I can do this much penetration and what I'm drilling in terms of seeing in the ground.
1:10:12 You know, well, in the wellboard, well, okay, well, if I don't reach the exact, you know, depth, it's fine. I'm going a lot deeper than what I was going before. So come on, guys. So not
1:10:24 everything has to be perfection. So sometimes we pick a lot more on AI and software because we trust the hardware, right? You know, we're still people, you know, in the industry, you know, we
1:10:35 love, we love steel, we love steel metal. Now that's, that's very true. It's, uh, well, like I said, it's, but then you flip it. It's, I think it's just an engineering problem, right?
1:10:46 Like as an engineer, if I hear 80, I'm like, Oh, that's not great. Yeah. But the, the real world, what 80 means in the real world of the actual application or use cases, when you're the one
1:10:59 who's having to do it is completely different. So for example, I was, I was mentioning to you earlier, so we had these generic cases where the system takes your, some information and this. Okay,
1:11:12 this is what the symptoms based on what you're telling me. This is what could be the problem with your electrical submersible pump. I tell people this is not what you know, you're going to take and
1:11:24 you're going to make decisions right there. Right. The system is going to give you some basic information based on previous report. It's your job to go to your team and say, you know, let's
1:11:34 analyze the situation. It could be one of four or five scenarios. Maybe it missed one scenario. Maybe it made a mistake on one scenario, but it's not the end of the world. So it is just it's an
1:11:47 assistant and it's a helper, you know, it's it's augmenting your your, you know, it's helping you make decisions a little bit faster. Yeah. No, that's exactly. Augment, I think, is the
1:11:57 perfect word, right? Like it upskills you much faster. It augments how much productivity you are able to generate as a person. Right And so that's, no, I think that's, again. wrapping this up
1:12:09 to the whole knowledge base thing, right? Like, that has to happen for the energy industry to be able to keep going forward, in my opinion, especially if we have another boom cycle. Like, I'm
1:12:20 right there with you. Like, I truly do believe that this is a, like, urgent matter. That no one is paying any attention to, and all it's gonna take is one more or one more boom cycle and prices
1:12:34 jump, and there's no one there to hire for us to, and then, so then everyone's mad 'cause oil prices are high, and it's like, well, oil prices are high 'cause you made everyone hate oil and gas,
1:12:44 so no one went to school for oil and gas anymore. That's right, and it's not cheap to hire - No. Go try to hire petroleum engineer master's degree. I was gonna say, experience one. They're
1:12:54 making more money than data scientists. Yeah, I believe it. All right, man. It's a rarer person these days, which is kind of crazy. Doctor, I really appreciate it I love it.
1:13:09 We're definitely going to have you back one day. We'll have you back. We're getting ready to all announce it here, but we're going to start doing some panels and stuff on specific topics,
1:13:18 roundtable discussions, and having a little quiet and stuff. I love to talk to you guys. I love your place here. We appreciate it. We've got some good drinks. Yeah, we'll have a drink next time.
1:13:26 I like it. Well, and on that guys, where can people find you if they want to reach out or get in touch with you? I am on LinkedIn. On LinkedIn? Hottem Nasser, H-A-T-E-M, last name is N-A-S-R.
1:13:39 I think you guys may have misspell you. You put N-A-S-E-R. Here, okay. So I am on LinkedIn. You can reach out to me. I will, you know, my email. Somebody wants to reach out with H. My
1:13:54 initial first name, initial Nasser N-A-S-R at softserveinccom. Offserve like the ice cream Perfect, appreciate it.
1:14:05 Guys, thanks for your time. June and next week, make sure you give us a like or throw us a comment. Thanks. Thanks a lot.
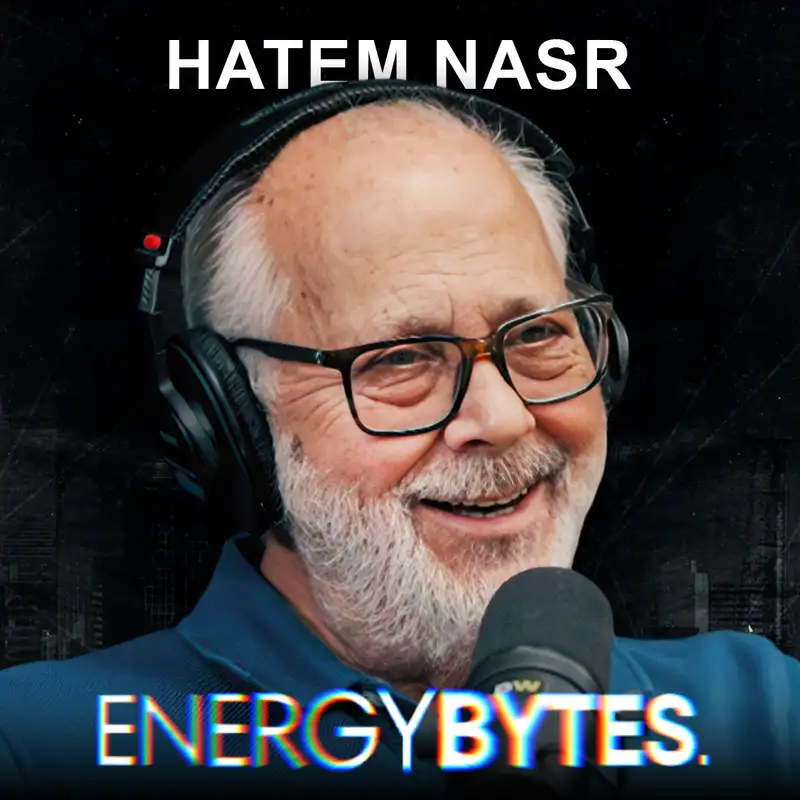