EP 51: Robert Stover from Enverus
0:00 All right, well, welcome to another episode of energy bites. I'm Bobby Neelon. Got the rad dad here, John Kalfayan. How's it going? Hogs have been one-in-one, but pretty competitive. It's not
0:11 a completely lost season yet. A half loop here. Yeah. Well, I was talking to Sydney this week in Calgary, and I was like, is it nice that you just don't have to worry about football? She's a
0:22 Florida State fan. Oh, that's right. She was like, yeah, it actually is. It must be nice that your husband has a different team that you can at least pivot to. He's a Penn State guy. And so
0:32 it's like, at least you get that. Yeah. Me and my wife are just screwed. It's insane. It's an insane house. Bad floor state. Yeah, that's so fast. But
0:41 we got a
0:44 comeback match up this weekend. I hope we can perform on. I don't even remember. It's a no-name school. But you should take your business. Yeah, fair enough. All right, well, cool. Excited.
0:56 We've got Rob Stover here from Invares. It's the senior director of corporate data analytics. So, you know, thanks for joining us. I know you and I've come back and forth on social media and
1:05 Slack channels and stuff quite a bit. I know quite a bit. Yeah. It's nice to kind of finally meet you in person. You guys have been following kind of the podcast a lot. Awesome. There are people
1:12 in it. Yeah. Being in the industry before. So, yeah. Happy to be here. Great. Well, and thanks for making the drive from Austin. Absolutely. Absolutely A lesser man would have just done a.
1:23 It's good to escape some time. Yeah. It's a lot better. I mean, we've had some good ones like virtually, but it's much easier to do a person and just better environment and always get compliments
1:32 on the setup here. So,
1:35 but yeah, I mean, usually, we kind of kick it off with a kind of a current event or something going on and date our attack. And I think I tagged both of you in it, but I kind of quoted it. But I
1:44 think five training, these CEO to the analysis are one of, you know, someone is a George Frazier, I think. Yeah. And so I'll kind of quote this and we can kind of talk about it here. We, you
1:55 know, Some people kind of got it into it in the threads as well, but it was, you know, so they did an analysis on snowflake and redshift, uh, if I come in the query history or whatever, and
2:05 they, they were able to tell that basically of the queries that scan at least one megabyte, the median query scans about 100 megabytes. So that's the 50th percentile, but then the 999th percentile
2:15 scans about 300 gigabytes, you know, but I mean, that basically what that means, like every, almost every query that's done is under 300 gigabytes of data scanned Um, so basically 999 of real,
2:28 real queries could run on a single, you know, large node computer. Like, you know, so do we really need all this distributed compute? You know, is it really necessary? And again, some of this
2:37 is that the technology isn't able, so you don't have to do that, right? But, you know, now we've got duck DB and some of these, you know, really efficient query engines, like now we can push
2:47 more things down, down to the edge. So, um, and I think we've all kind of known, especially in oil and gas, like we We don't have big data, and again, I think even what was big data. 10 years
2:56 ago and I got in the industry is not big because, you know, like, and what more is law and all these things and storage is cheaper. So like, you know, what you can do with data is just so much
3:05 better. But definitely, uh, it's sad to get your take on that and kind of dive into what you're using because, you know, I'd say, probably outside of me or more than me. Like you, you keep up
3:14 with those things more than just about anyone else in industry that I, that I know. So no, absolutely. I mean, it's not surprising. Yeah, no, you're going to, it's not surprising. We're
3:21 going to talk about it, which makes you a little bit closer to the mic It's not surprising that the results are, you know, sort of what they found and what they're seeing. I mean, you know,
3:30 there's just not a lot of companies that are dealing with that kind of scale on a second millisecond basis of data kind of coming in and being, you know, analyzed or aggregated in some sort of way
3:41 for, you know, data applications or, you know, processing and workflow kind of kind of things.
3:48 So yeah, it's just not surprising I mean, to your point, you know.
3:53 analyzing data on the upstream side, you're just not dealing with tons. Unless you were on the accounting side, maybe invoice volume and stuff, which - Yeah, but even like a GL is not big data
4:02 relative to what people - No, definitely not. You know, I'm talking about - There's a lot of Ubers, right? Or Netflix, you know, where you get, you know, daily millions and millions of
4:11 rideshare data, right? That's where, you know, they're building custom, custom in-house kind of things. That's why a lot of those, whether it's ETL tools or, you know, Spark or all these
4:19 things that are born out of these companies, 'cause out of necessity - In a necessity, right? But again, like using, like say, snowflake, but I think even though like, you know, especially
4:29 you're tied in like this, you see these things and you almost, is it an imposter syndrome thing or whatever, but like, I mean, maybe we're just not that important or, you know, it's like, or
4:38 they do it like, again, they do, I think they talked about it in the article too, but like, they do all these benchmarks on 100 terabyte data sets and it's like, well that's great, but I mean -
4:47 It's 100 terabytes, yeah, but that doesn't apply to, probably now 9999, 9 of the customers that are actually, you know, evaluating them. Yeah, yeah, yeah. And so, yeah, so in one of the
5:00 reasons I think by myself and even realizing what kind of data we were working with at Inveris, we're still a B2B
5:08 company, right? With a limited set of customers, you know, 6, 000, 8, 000 logos, annual kind of transaction volumes, right? We're not dealing with like monthly SaaS things You know, coming
5:20 and going and upgrading out of app and doing a lot of digital things, where it's really P, like PLG or product-led growth type of stuff. Yeah. Like I said, the biggest data sets we've got are all
5:31 internal data sets, right? Like we're looking to scale out some information about our customers because they've got, you know, large counts of invoice volumes. So I got to work with open invoice
5:40 data, right? Or I want to take all the prison data that we got, you know, about our customers and say, okay, sweetie, how can we use this to know more about
5:50 market to them better. So that's like where our fun, maybe larger sets of data come from, but still, in that sense, it's just so nice and fit for purpose. Even in that medium data set scale,
6:03 where Mother Duck and DuckDB work really well,
6:10 the performance is just so crisp and clean, and the implementation and architecture or maintenance is just nice and robust It just works.
6:20 You go click a button and within. I think someone was talking about that, too. So the chose who broke in or the guy who's actually all about Databricks, but I think someone was in one of his
6:30 replies talking about just the time to sequel with snowflake. It's been in it. It's five to ten minutes. If you can get data in it, you can start writing sequel. Whereas I remember evaluating
6:42 snowflake and Databricks, and again, I'm sure Databricks has come a long way in the last three years, even. But when I started at GME, I'm like, I have Azure and I still. can't stand up
6:51 Databricks and know where the hell to go to put the, you know, and I just like, I just go sign up for, you know, snowflake in it. It works. I've got an end point. I can connect things and,
7:00 you know, it just flows super fast. Yeah, super fast. So kind of, you know, jumping into that, you know, I like to kind of work backwards through your career, but like, let's talk about what
7:11 you're doing out in a various and various is a massive company at this point, and you've just acquired bid out, you know, former, uh, yeah, I guess, I guess. Shout out to Rodney. So him at
7:21 Calgary this week. No, nice. And, um, but again, you guys have acquired a lot of different people. You have a lot of different product lines, everything. So like, what does that mean for
7:28 what you're doing there? Cause I mean, like, I think a lot of people when they think of embarrassed, they think of prism in the front end and all that kind of stuff. But, uh, just curious where
7:35 you fit on now. So, uh, a little lineage, I guess, you know, where, where my team really, uh, came into the fold and embarrasses is, is before, before I came along and stood up that
7:48 position, that central sort of data corporate. data analytics function, it was very much like a sort of, you know, purely self-service like Wild West, like go grab data from a source system,
7:60 throw it in Excel and like build a model and then there's your source of truth. Okay. Right. I'm going to go grab, you know, deal data out of Salesforce and then go combine it with some stuff
8:11 from the accounting and the ERP system and then I'm going to, you know, create some custom mapping things in Excel and then like that analyst has the thing and that goes up to the board and then
8:18 there's that, right? Okay. And obviously with the size of the company, you know, which is, you know, I think we publicly stated. I mean, it's a large SaaS business, a mature SaaS business
8:29 that's got 40 plus unique applications that we, that we sell,
8:35 you just needed more than that, right, to scale and get the level of insights that you just can't, you can't control and manage and govern the right way in Excel. So I came in and said, hey,
8:43 like, we need to build a proper way of doing this through a, through it, you know, data architecture and, and. system and I think I can build that here for you. It started immediately with the
8:56 need to look at some of our key corporate metrics, which for any B2B SaaS company is annual recurring revenue. It's the subscription metric. All that data lives in something. You can look at it by
9:09 customer and account and product and see who's churned, who's upgraded and downgraded. That was a goal initially. Do it cleanly. Do it in snowflake. Build models that are governed and managed and
9:20 then populate that out to the end user students finance and the like. So, okay. So, I mean, it makes sense when you think about your your title, but so you are about data analytics for your
9:30 internal customers. Absolutely. Yeah. And again, it's just kind of ironic because like obviously you guys have strong data competency on like how you provide data and write applications for like
9:41 your. Well, it was always it was really fun Like the
9:46 guy who hired me was sort of like this. The consternation was, hey, we do analytics really well for our customers and our products, but we don't do it so well for ourselves. Yeah. You know,
9:56 it's a eater beat and kind of world in there, and like we're just all doing things like in isolation. Sure. And so that was really just to catch us up like internally, like let's mimic some of the
10:07 processes and best practices we were doing on the product side
10:13 to allow our own business to just be better. Right, leverage the existing You're helping everyone else's business be better, you know? But then, you know, you're kind of sabotaging yourselves.
10:24 'Cause again, I guess if you have talent too, you're like, it's probably more of a value than putting to the talent right now. Absolutely, we do have a large, obviously tech work. I mean,
10:31 we've got tons of data engineers, tons of SQL experts, you know, most of our backend product teams are in data breaks funny enough. But obviously leveraging or needing to kind of scale out, if we
10:45 need to, looking internally, is a super hazy thing. Sure. Yeah, so. Nice, I wanna talk, I wanna unpack it a minute 'cause I feel like lots of people in our industry would love to go from
10:58 moving away from their spreadsheets into a more structured, organized way. What are some of the kind of the big things that either challenges or like wins that you guys had there and you can have
11:09 pro tips for anybody that's, 'cause that's a, I mean, that's a daunting thing, especially like in our industry, it's not just our industry, but it feels like it's always our industry, right?
11:19 Everyone has a spreadsheet template. They've, you know, it was a base template and they've customized it to the way that they want it, right? And then so it's like, how do you, I've always
11:28 admired this about Bobby too, like how do you get the users out of that thing that they love and are comfortable with into a more formalized process? Yeah, I mean, it's the Aries challenge, right?
11:42 It's like, how do you get an engineer to like, to think differently than what they've been doing for a long time, and that's not to say that we don't still really love and promote like people
11:52 working in Excel. Right, you gotta have both, right? You gotta have both. And there are certainly, and I can talk about it a little bit, I mean, one of the reasons we just went with SQL or
12:01 Snowflake is because we knew like our analytics community internally wasn't like this like, you know, sort of modern analyst who can like just spin up a notebook and start writing Python and then
12:13 build this little custom and be like, okay, sweet, I did the thing to build a model and I published it and then there's an endpoint for it, right? Like I hosted it myself. Now, they're mostly
12:21 still Excel people. Maybe some of them have some SQL skills and they can kind of get into Snowflake and kind of write their own thing and then, you know, and then leverage it in Excel to themselves.
12:32 But, you know, part of obviously just being in SQL and being in a relational data model.
12:39 That was to be ensure that we always capture
12:44 The thing that we had to be conscious of is that what we wanted to break was just this sort of
12:52 natural sort of motion to be like, okay, I'm going to go grab the data I need somewhere, throw it in here, write all my formulas and like key metrics and things in that one thing where it only
13:03 exists. My big rule of thumb is Excel is not a database. And it shouldn't be treated as one Ever, right, ever, please quit doing that for everyone, right? And so the practice we had, the
13:16 culture change we had to start shifting was is that, particularly for the high frequency reports that were super important was, hey, if this data, if the logic and business logic exist in here,
13:29 we got to get it up stream, right? We got to put it in Salesforce. Well, you know,
13:35 the Salesforce is dirty. Well, if it's important that we're looking at, you know, industry values in our Salesforce system and they're not 100 and you're having to clean it up in here.
13:43 Right, we gotta fix that. Right, yeah. Because I can give you, and we do, like the part of like meeting them in the middle and not being like, hey, we're gonna get rid of Excel, and I'm gonna
13:52 put you in Power BI or Tableau, or something else is, they're not gonna change that way. So let's fix the underlying components of how they do their work in Excel, ie. just, hey, here's a nice
14:05 clean set of data and tables at a snowflake that you can then pull in. And then all you're doing is like building pivot tables off of already calculated measures that everyone agrees to, right?
14:16 Like this win rate percentage means this and you don't get to define it here anymore, right? So that was our first task. And we've been pretty successful at doing that. And now we're kind of to
14:29 the point where these users, a lot of our users, main users are like,
14:35 well, teach me now how to fish. Like teach me how to do the thing in Power BI or wherever that I can I can take that model you built. And I can kind of customize it myself, right? And if I need
14:45 something like a new metric, I'll ask you guys to help me curate it and standardize it. But none of this sort of Wild West approach anymore. So it's not a
14:57 hunt and it's, we still battle it all the time. It's some people, you know, or take the pack of these resistance. It's just human nature. Yeah, and so that's where, I mean, I used for Marzane
15:06 what, 'cause like we try to, I mean, I think we use very similar tooling obviously too, but just trying to, like having the support from the top was huge for us too, like where like our C suite
15:19 would be like, if you're doing that there, that's wrong. Like, like, you know, and again, I've always said that Excel or Power BI, whatever, they're great prototyping tools too. Like, I
15:27 mean, I want people to take the data and play with it and like they're the experts in their domain, I want them, but we need to have those, like that cycle and where we're iterating and like, if
15:37 we realize this is something that's like you're saying, it's gonna be used as a, corporate metric or then an answer is being derived from this. It needs to be, we need to institutionalize that
15:45 knowledge upstream. And again, it'll probably be done more efficiently because snowflake can crunch that again, millions of records much faster. And then you just pull it down and select stars a
15:54 lot easier than it is. I mean, in, you know, any helpful tips, right? That you asked that question, um, it, it really does start with, we took, like we're a lot of data teams sometimes fail
16:07 is that they try to be, you know, somewhat of a semi owner of like data. We're not an owner of data. Like a data team shouldn't own any of it. The data, the ownership of the data falls on the
16:18 person who should have a data contract upstream that says, Hey, like the data is going to be of this quality of, of, of this, um, respect. And if it's something that again is, is, is, is
16:30 populating or impacting a, a, a, a KPI of some sort. Then hey, if it's, if it's broken, we'll tell you. like we're just gonna be the ones who alert you of it. Like you have to go fix it and
16:42 we'll make sure that we model it the right way and we deliver it with the right, you know, a cadence to impact the business. Yeah, that's, yeah, I got a very similar path. But I mean, yeah,
16:55 like if it's broken in open wells, you go fix in open wells. Like, you know, I'm not gonna write Band-Aid code in the middle, like, then I have to keep updating a case-win statement to, I know,
17:05 like I should be able to pull from this and it's right You know, and again, there's value in it being right in that source. It is, that's me. So, let's maybe let's talk about that.
17:16 Whether it's like the data, the quality testing and stuff, and then like, how are you capturing that and then kicking it back to those users? Yeah, so, you know, nothing, like, we haven't
17:25 gone full, like, I'd say, like, sort of the data contract approach where, you know, we're giving them sort of strict, you know, building strict tests at the source that completely fails if it
17:37 fails.
17:39 Of course, I say that in some in some regards we are there are certain data sets like our application measurement platforms. I don't know. You've obviously maybe you've you've seen or heard some of
17:52 those like
17:54 like CDPs customer data platforms. So they've got capabilities to basically, you know, put little SDKs inside your application and you can see like when people are doing certain things like in
18:05 Prism or wherever I can see like Bobby, when if you go in a Prism and you export a data set. So right. And so that measurement system
18:15 captures it on the fly and then sends it to Snowflake through a platform. We use Rutter stack as our sort of measurement protocol. And we actually have strict governance there. Like if we expect a
18:25 certain data quality, like certain data quality about either the user or about the thing that they did in the platform, and it doesn't conform to like strict standards, then we reject it and we're
18:37 warning it back up. But like with Snope, like with Salesforce and stuff, like it's mostly just a, we run our standard DBT tests. Right. Make sure that, you know, the main objects coming out of
18:48 there, the main columns are getting so your standard stuff. And then we're just having a regular cadence with RevOps to say, hey, listen, like, here's all the things that failed. Yeah. And
18:58 let's just work to address it. Okay, so let's get in DBT 'cause I don't, actually, yeah, I've talked about it a little bit, but I don't think we've actually had a user on yet We've had just
19:07 conversations we've never really dove into. So
19:12 why don't you go and then I'll fill in if I think there's things that, you know, but like what is, for people to understand, what is DBT, what is it, like what is it handle? Sure, sure. And
19:21 why? When why, yeah, I mean, I guess, you know, sort of the old school approach, right, was if you had a SQL server, you were, you know, building, you know, SQL databases, you'd, you
19:30 know, you'd wanna, you'd have some, you know, sort of dag or procedure of models that we're interconnected, creating materializations
19:38 And those are usually managed by store procedures and, you know, but that DBA or, you know, data engineer, we have to manage all the DDL and all the things about SQL writing that maybe people
19:49 don't like, right? DBT was sort of built, you know, data build tools as a really nice analytics tool for data teams to work with cloud SQL environments to sort of abstract away all the crap that
20:03 people don't like to do and they are managing databases and models in SQL and just let them just write SQL models, right? Select statements and managing, you know, intermediate, you know, sort
20:16 of data transformations from, you know, raw data that was being landed into something that was going to be delivered to BI interface, right?
20:25 And it's, you know, all, you know, started as an open source sort of sort of protocol, really easy to use and it could obviously like any, that starts out open source. They end up having some
20:36 sort of cloud-managed environment. We use that cloud-managed environment now. And it's got, you know, nice orchestration in it. So we've got, you know, we build our analytics models. It's all,
20:46 you know, CICL-managed and GitHub. And we're able to iterate quickly in a development environment. We pass all our tests and, you know, the outputs look good with our stakeholders. We published
20:57 a production and we just manage it like a data product at that point, something that's reusable and easy for the team to collaborate on. Um, it's our, if I had to put a percentage on where most of
21:08 my team works, you know, from data engineering all the way to sort of analysis building, 80 of it's probably in DPT building models. Not for sure. Yeah. Yeah. So many, you definitely hit on
21:20 pretty much all or most of it. I mean, like, so I mean, like the orchestration. So like, again, like, you don't have to club before a lot of people would be used airflow or, I mean,
21:29 I mean, if the most basic people here are listening, you probably think of these as a Windows scheduled tasks that would run on Python, Scripty, or a cron job. But I mean, they handle it, so
21:38 you can, you know, they have a pretty good CLI, you know, language where you can say, DBT build, XYZ, exclude, or select these things, you know, whatever. So again, you can choose how
21:48 often these, you know, pipelines run, but then I think you hit on it previously, but like, say like the testing, I think is a huge thing. It is you So you can write tests and you can, they
21:58 have a lot of them out of the box and there's even like, they have a lot of packages to the people who are saying that's, that was what attracted me to just all the packages that they have. It's
22:06 like, oh, because I originally looked at it with five tran for our like social GA, all that. And it's like, oh, shit, DBT has all these templates that normalize all the social channels and the
22:17 same convention. I'm like, oh, that's all some problems that everyone works with with those standard sources, which is
22:23 really nice. Like I'm using up the first one to come up Exactly. No, that's the most frustrating thing when you're like, I know I'm not the only person doing this. There has to be a library or
22:31 something out here. But I mean, they have the standard ones that are fairly comprehensive, but then there's like the DBT expectations, but
22:40 there's all different kind of things where the data is outside of standard deviations. And normally detection. If there's duplicates, or if the row count equals, if it's just a join, like the row
22:50 count should be the same in the next, if you have a bad join, it'll flag If there's all these things, and you can, again, it's some of us for us. But like, I mean, we started writing the tests
22:59 that tested like the source data, and then I've been using the DBT artifacts, and I built actually like a spot buyer. Yeah, that would be the impact on those downstream tools. Yeah, but we would
23:09 tag them with like the source that we're at failed from. So then we had a dashboard that would go out like a spot for our automation job, and it would go back to the business units every morning and
23:19 be like, There's five records, and I built it where they could click on the row count, and then it would show them the rows that we're failing. Oh, this prop numb is where I need to go, you know,
23:29 of course, it's problems, you know, whatever it is. But like, you know, we go back to our land team, our ego fixes and the ERP or, you know, you know, yeah, we did that a lot. I mean, we
23:40 will do that with certain records, opportunity IDs, things like that with Salesforce data, particular we will, we'll flag back up to a RevOps counterpart and say, Hey, like, you know, this
23:50 contract is missing, like in dates, like that shouldn't be allowed kind of thing. Right You know, we help them out. We try to, we try to participate right in data quality as much as we can.
23:60 Yeah. Cause again, like we're, you know, own it, but you are genuinely familiar with like all the different sources and can write things. We don't own it, but we also see, we still also see it
24:09 through the lens of like being, actually from being used. Yeah. Right. Like it's not through the interface and the application. But you do own it downstream. Yeah. Yeah. You know, again,
24:16 like if, if something's creating duplicates, like in this, you know, really critical model like all of a sudden like people see this in their production values are doubled, like, that's a
24:25 problem, like, and you can't, I
24:29 mean, and it's the one thing you can do with those tests, you can either have them worn, or you can have fail, and that way, like, anything downstream of that's not going to get the bad data.
24:37 And then, you know, you get it and you can go around with it pretty quickly. But, actually, we didn't talk about the documentation either. Documentation piece is nice because, you know, like I
24:46 said, governance and data quality and communicating out like what things mean, especially at a company that size. It's a critical piece to just success as a data order, right? You know, giving
25:00 that person that metadata, that ability to kind of come in and be like, okay, that's what that means there. That's what that unique ID means there. That's what, you know, okay, this is in,
25:09 you know, US. currencies and this is, you know, okay, et cetera, et cetera, right, versus them asking, hey, like, what's this new column mean?
25:18 I don't know, you know, let me find out, right? So. That piece is critical. We're actually just about to expand into an enterprise relationship with DBT where we get much more of the new DBT
25:29 Explorer features. Yeah, they have all the column level lineage now. Column level lineage, which is huge for us. Internally, it's obviously like, sometimes you just don't see the impact of like
25:38 that one column that, hey, someone asks, you know, hey, can we get that one obscure thing that would I only need? And then like, it gets added here, but then it like crashes like a three of
25:46 our models. No, yeah. So ho. Yeah, 'cause I mean, like one thing that DBT's always had that looked, it's really cool. But it was like the table level lineage, but then all of a sudden you get
25:54 in there like, well, if you're really glad column level lineage. And then we even tried, I think it was data fold for a little bit, and it just didn't have enough value. But it was one of the
26:03 only things we could get our hands on two years ago that had column level lineage. And then being early on, it helped one of our analysts help with some of the documentation where he could trace it
26:10 all the way back more easily. But now I mean, DBT's weaving it in and then sequel mesh. There's actually some data governance tools now like governmentary that - So we'll do a lot of the anomaly
26:22 detection and something like the
26:25 more advanced unit testing type stuff on top of DBT, but it also gives you common level lineage too. So if you don't want to go fully scale onto DBT enterprise because you like the open source
26:35 package, then there's lots of tools now that integrate with and let you see a full scan of your metadata on your columns and where things are broken or where not. Yeah, it's super cool
26:49 That is, I mean, people that haven't done ETL just don't understand the nightmare that it can become. Yeah, absolutely. There was actually one, I don't know if you heard Colesse, Colesse is a,
27:00 they had a really awesome one 'cause you could like put a new column in and like the source and then like say, how am I gonna push it to these downstream ones automatically or not? Okay. And it
27:09 would actually go and like say, okay, yeah, propagate everything downstream of your data. Is that a little more gooey-based? It is a little more drag and drop that it was expensive. I said, No,
27:19 thank you, it's awesome, but I'm not going to. Well, and that's a cool thing. I mean, like you said, DBT is a Python package, but it is, and you can run it for free. But,
27:30 I mean, it sounds like we're both using DBT Cloud and there's some other DBT interface people out there, even I think a astronomer has their own name, which is the people who do air flow. But
27:41 again, you can run it on your laptop. You can't. Well, unless you're me Well, it's been like two days with the DBT. Installing Python and running package, that's its own beast. But
27:54 again, that's where managed services come into play where it's like, it's total cost of ownership. You get build for spy argument. Yeah. But yeah, so I was with that. So I'm like, 'cause you
28:04 and I, you started a few months ahead of me, but at Ameris, but I mean, maybe you got to start something greenfield, that sometimes seems like we picked similar thing. Can you go into just the
28:13 core pieces of that stack? Yeah, yeah, yeah Yeah, when I started. It was basically a data team of basically one. Yeah. And so, and we're really quickly, I actually hired a cohort of mine at
28:28 Parsley, who was sort of a data engineer type. Okay. And so I quickly brought him on as sort of my data engineer that kind of helped me out. But we still realized we needed something that easily
28:38 allowed us to scale quickly. Yeah. And that was, you know, with that sort of thought and mind and knowing that, you know, just as a, not necessarily a cost center like that, because I don't
28:50 really truly believe we're a cost center anymore. We had to be pragmatic about the tools we selected and whether we went full, you know, build versus buy. And just, and so with that in mind,
29:02 like we said, okay, hey, like we have some money that Envares is willing to invest in us building a first class, you know, sort of data asset. We went with five train to start out with because
29:12 we, you know, at the time, We were only really connecting Salesforce and like our ERP's to share them. you know, why, why go through the trouble of like writing your own custom API? Like, that
29:23 API is a particular one we didn't, we weren't familiar with, like, you know, because you came out of oil and gas, right? You know, I didn't know what a, you know, what a upstream operator is
29:31 like, you know, I didn't know what the opportunity really was. Like, I didn't, you know, I didn't work in that sort of language, right? And so, hey, five train was there. Like, of course
29:40 we got in before they changed their pricing model. So you're like, oh, hey, this is, this is like, we're getting a lot of value out of this. Yeah. And it works Like, the problem is with five
29:48 train, right, as it just works, it's expensive as hell, but it works. No, I mean, I went through that same thing, like, because like we evaluated five train, Stitch and then Hevo, and it
29:58 was like, Stitch is going to be half the price and Hevo, but I tried to get someone to tell me a bad thing about five train and I couldn't, like they just kept direct. Oh, they had great customer
30:07 service. Yeah. It just freaking works. It just freaking works. They even do like, you know, type two sort of loading on the fly, you know, certain sources make your life easier. It's just,
30:17 no, we haven't already talked about it. Can you talk about, like, type two, and like, it's really kind of old for people. Simple case, right, like, a lot of, a lot of slowly changing data,
30:27 right? Like a lot of, particularly out of transaction systems, transaction systems like a Salesforce or a CRM,
30:35 data components of about a certain entity, a customer or an account or whatever changes over time, right? Bobby might move, you know, live in, Houston, but then he moves to Dallas and so. But
30:46 at the time he lived in Houston. At the time he lived in Houston from this period, this period, or hey, he worked at, you know, he worked at Grayson Mills and now
30:56 Devin, right? So if you only ever pulled the transaction system today, he would show, you know, Devin, right? But from a reporting and analytics standpoint, what do you need, right? Do you
31:06 just need to know that he only works at Devin? Or do you want to know like back when they did the one thing and they had the money then, you know, spending, you know, doing things in your
31:13 applications, that he worked great. So type two, sort of database management, revolving out of the, obviously, the Kimball sort of design processes allows
31:25 you to absurd and manage that data set so that you can capture multiple states about that entity, right? And so, obviously, DBT, it's just convenient 'cause it gives you a lot of convenient tools
31:39 to do that. But you can still gotta write it and you gotta manage it, make sure you're grabbing the right unique keys and time stamps to manage it. Five train was just offering, Hey, we're gonna
31:49 just do it for you, right? On the fly, zip it in there, and here's your type two. And our practice, typically, is we only really, if it's a, you know, a core entity, a customer, whatever,
32:00 a dimensional entity, we're always, we only work in type twos. 'Cause you can always get to the current version and historical version. No reason to just keep a type one. Yeah, that's a whole
32:10 point of a data warehouse, right? I think we have to see, yeah, you can have every - And it's crazy, like our type two database of our Salesforce accounts object is over a billion rows of data
32:24 now. That's over 8, 000 accounts. You can imagine how many times the owner changes and the account segment changes and write all the little characterizations about how you profile them. Sure. It
32:34 moves a lot, so, yeah. I believe that, I mean, one of the things I was going to set up through 5Tran was our HubSpot instance And then I realized I had it set up. And then, of course, it was
32:46 double any other source each month. I'm like, Well, maybe we don't need a full on backup of this in 5 and snowflake. Yeah, but you do pay for it, right? 'Cause it's like, obviously, 10 times
32:58 your normal Mar, but I think it's worth it. So, yeah, 5Tran, although we are getting a bit more pragmatic now, like we are moving into experimenting and testing out more, Uh, you know, Home
33:12 brew kind of kind of data pipelines through snow pipe. Sure. For example, this is no pipe I mean snowflake. I think naturally is obviously kind of working on building their own just straight
33:21 connectors to go to places, right? You can get it from Google analytics. You can get it from a Service now like there is a salesforce one now, right? There is there is but you do have to have
33:31 like that. You have to have a certain
33:35 Salesforce data cloud like like a plantation to do this sort of okay where they call CDC or mirroring right? So but we do have that so we are actually in the process of implementing that nice We just
33:46 expanded into that aspect of Salesforce recently But DLT is a new one, right? Yeah. Yeah, so that one's actually really interesting. They're library still small But it's like the map like you can
33:58 basically build a full pipeline and like 20 lines of Python Yeah, with that's using their DLT libraries really cool. Who's it? Isn't that basic? Is that a Daxter or who's? Or are they just
34:09 they're on their own thing I think they're out of France, maybe okay. Yeah Um, so that's a real, I'd encourage people to go look at that one. Cause it's, um, it's open source and it's, uh,
34:18 it's, it's really easy to use. Um, so we are, we are kind of trying to self like the little more pragmatic about where we spend our money. Um, and what value does the ass data asset bring? Okay.
34:30 If it's really important, five transgrade. If it's, if it's like Jira data, which is a lot of volume, but like, yeah, like this is that important to us. I don't know. Maybe not That's the
34:40 hard thing to like, that was probably the hardest thing to swallow. It was like, I was going to pay twice as much for getting the data into my data warehouse as well as spend for it. Right.
34:48 Exactly. Yeah. And like, then there's no inherent value in the data moving, like getting it from here into here, like that doesn't add values. What you do downstream. Yeah. I mean, but now
35:00 you have to have it all centralized or some way to centralize them. Um, but just moving the data, you know, you pay a lot for it and you're not.
35:14 So any value from that? Definitely trying to like definitely try to ship where the money's being allocated and what value would get out of it. That's the average view. But I mean, again, if you
35:17 hired a data engineer, they wouldn't be able to build those pipelines and you'd pay them three times as much as you paid for - You would. That's the trade-off, absolutely. No, that's where five
35:29 train gets you, right? Is it's like, yes, I know this is expensive, but even like an intern, if I hired an intern, that's all that they do. That's all they would do, right? They would
35:39 probably still be more expensive currently than what my five train bill is. It's like, well, okay, five train a day is right. So then everything else then revolves out of Snowflake as a central
35:50 data store and data warehousing solution, analytic solution.
35:55 Obviously we manage DPT on top of that.
35:60 I had mentioned Rutterstack earlier, 'cause we - Where does that sit? I'm not actually not - Yeah, no, Rutterstack, I mean it, You know, because it was such an important data. for ours. I
36:09 mean, we, us being a SaaS company, like us knowing what our users are doing or not doing in each platform is like of hyper importance, right? Because you want to manage churn, you want to, you
36:21 know, decrease your gross retention, you want to find people who are doing things that, oh, hey, maybe I know that, you know, you guys are doing XYZ, we're going to tell the sales team and
36:30 sales team is going to see those indicators to say, hey, yay, we think you should actually buy this new thing, right? Because everything, because then it's just like, you're just guessing where
36:38 they're having to have a conversation with you, that measurement platform where it essentially sits in every app. So every product management team is responsible for, you know, what they want to
36:53 track, working it through RutterStack, RutterStack, then it's just being a routing device that delivers it to Snowflake, so then we can like model it and say, hey, this is our daily active users
37:01 in Prism, right? And no, by the way, like these are
37:06 the ones that are doing custom fusion data sets and these are the ones that who are onboarding and then they sort of died off. So let's try to remediate any unhealthier at risk customers, right? So
37:17 that's probably, we are just wrapping up that project. It's probably the most important project we've done this year because just having that level of insight about our customers is how we grow the
37:29 business and how we keep them in house, right? So that sits, again, it's
37:36 most traditional CDPs, like a segment, they would have had their own database basically in the cloud and like they would have managed it and you had to integrate
37:47 it and batch it into Snowflake. This is what you would call, I mean, I'm missing the term, but it's just a service, right, that doesn't store any of the data, it just delivers it straight to
37:56 Snowflake, like composable, that's where it was, composable CDP, right? So that worked really well for us because it was cheaper, you know, basically I'm just paying for a VIN volume at that
38:06 point.
38:08 So that, those are like the two, the two core pieces about the data that we manage and collect. So you can think about like the data pipeline being event streams through RutterStack and then like
38:17 application, you know, batch jobs. Okay.
38:21 I talked about DBT. We're in the process of now thinking about like the semantic layer kind of like, you know, governance and like DBT semantic layer Yeah, I think that's, you know, probably
38:35 we'll end up going just because it's there and it's, it's, they're improving it quickly. What I found out recently is that Snowflake is going to be able to handle those like semantic layer objects
38:47 in their warehouse. Okay. So like, say if you had this semantic model that said, Hey, this is like, this is your revenue model, right? And here's customers and here's, you know, your
38:56 calendar information And it's all just one big yaml file, right? Just one big readable like thing. Like, this is what, you know, this is what profit means, right? This is et cetera, et cetera.
39:05 You just upload that to Snowflake. It's still like already knows where the tables are. And then you can, you know, you want to put a chat bot on it. Hey, like tell me, you know, tell me my top
39:12 10 customers in the operator segment by ARR. Okay. Yeah. Conoco did it. Right. And like it just knows. Cause it has that semantic knowledge that, that human readable knowledge that says, well
39:24 don't just take a wild guess about where, what SQL you need to write to get to the answer. Like where I'm telling you where to find the answer. Okay. You can bounce on it or whatever. Yeah, put
39:30 bounds on it. So we, you know, that's something, we have our eyes set for 2025. So one thing there, like I've been a little hazy on the semantic layer. So a, I think first, but maybe
39:41 something back, semantic layer really isn't a new concept. It's a cube for essentially, right? Like, like, and that's really what's going on under the hood in Power BI even, right? Yeah. But
39:52 like how does, like, I mean, where my heartburns been and maybe it's just ignorance, but like, all right, so I've got a DBT semantic model. Like what can read that? Yeah. You know what I mean?
40:02 analytics tools, I can even read from that. Yeah, there's quite a few now. I mean, actually - But are they mainstream, like? Well, funny enough, you can go onto the Microsoft App Store and in
40:11 Excel download a direct link into the DVT semantic link. Okay. Yeah, and it'll be like, pick your, you know, pick your measure, pick your thing. And if you want to just, as long as it's been
40:21 put into production and then in the spantic model, like I said, maybe you have one for revenue, right, or win rate or pipeline. Okay. And you said, hey, do you have the dimensions that govern
40:29 it? You'll see like, okay, I can look at it by region, by customer, my product, and I picked that thing, and it just pulls into a pivot table. Okay, cool. Like the analyst doesn't do
40:38 anything else, right? We have Hex as a notebooking platform, cloud-based one, we love Hex, like it's one of our
40:47 tools. It is really cool, I mess around with a little bit. It integrates straight to the DBT semantic layer, so again, like if you wanted to be sure that, hey, if someone pulls win rate from
40:54 Excel or Hex, they're gonna get the same answer, right? And it's consistent, and they don't have to think about the sequel they have to write. New Did I? Did I? Did I do that? write SQL that
41:04 you told the semantic layer to run when it submits that query, right? And it says, okay, I know how to pull that together. And abstracts away all the SQL that you would have written to build that
41:14 metric or that table or that join or whatnot. So, the hex is really cool because anyway, we can dive into that for people. But like it's, like I said, it's a notebook thing, but like I can
41:24 write a block in Python and then I can reference that block in a SQL statement below it and then I can do another Python or two SQL's to, you know, like, and you can chain them all together and
41:34 then you can use that to orchestrate some stuff. Well, applications too, right? 'Cause it's got Markdown. It's got, they have their own proprietary like visualizations and like table like cells.
41:43 So like you could be like, hey, here's this Python and then you build a data frame. And then like you say, okay, I want to bar chart and you can parameterize all the things about the data frame
41:51 like if it was like a, you know, metric A and B and C and then time and then some thing, parameterize it, add as a dropdown or whatnot or filter Here's that, here's that table. Yeah. And then
42:04 it's like a BI tool at that point. So we, you know, again, you might say, well, why do you have that in Power BI and all these things? You know, there's, I think for us, there's definitely a
42:14 very much like, we want to methodically limit the amount of dashboards that exist in the organization. Because if you don't, if you're not careful, you'll end up with 8, 000, right? Yeah. My
42:26 sweet spot that we're working for is like, you shouldn't have more than 50 or 60. Like total Okay, yeah, across the company. Probably any functional groups have two or three. Yeah. Yeah. And
42:35 so work with them to say, hey, what are the, what are the metrics that you care about? The drivers of those metrics. What's your 80, 20? Yeah. And let's build the 50 or 60 that, you know,
42:45 those metrics resolve up to, whether it's pipeline or whether it's, you know, some sort of support ticket or customer success, like, you know, tree of metrics, those are the dashboards, right?
42:56 That's them. Don't ask, don't like, there's no need for anything else. If you're telling me this is what the growth model like for the company. right? For those cases where they're like, well,
43:05 you know what, I really want to look at some really interesting like correlation, you know, analysis, and it's kind of a one off thing. Maybe I do this by, by annually. Sweet. Like, there's
43:15 this things that BI tools can't answer easily. Yeah. Because you have to conform the data in a certain way that limits also the analysis as well. But I can do whatever the hell I want in Python,
43:24 right? I can, I can, you know, build a logical, you know, logistics regression and then do the things and like, add a bunch of details. And that's what, like, that's what we use HEX4, right?
43:35 And so we do a lot of that where, you know, if we have a data request or some data product request, that's really obscure. HEX, it is. Like, people in HEXAP and what's awesome at HEX is that
43:46 you, you pay for it per seat. It's on a limited readership. Create an app, you publish it, anyone in the company can look at it. Yeah, it's pretty slick. So, um, and so, yeah, so, but it
43:56 also requires as a data team that, hey, You need sort of most more robust skills. You need someone who not only is like a. an analytic thinker and someone who can think about the what's wise and
44:06 how's about what they need, but also can write Python and write SQL and be creative. And obviously they're building out with Alowam's and chatbots and AI wizards, like they're making it more
44:18 accessible, right? Even like my, I have a couple of people on my team who we call like data product managers, right? Like because we are creating data products, like
44:29 it's really good to have a product manager for that, right? Hey, we built a product for you. We're gonna treat it as such. We're gonna feature, you know, grow it over time. But even they can
44:38 get in and they can play around and ask, you know, that the AI wizard, like, I'm trying to write this thing to do this one thing in Python and they can iterate and they can kind of prototype.
44:48 Sure. So it's really opened up the abilities of our team, you know, to manage an analytics request. That's cool. That's really awesome. I was gonna ask unless you've got more because I know you
45:01 know I can tell her. or vibing right now on that data, the data plan, but it's just going to ask like, how do you, how is your kind of experience that parsley helped you? Yeah, yeah, that's
45:13 where I wanted to get back. No, it's because like, you know, being on the service side is very different than being on the operator side. They both have their pros and cons. But I'm curious,
45:22 just, I mean, I'll say, parsley was by far probably the most, I would say,
45:29 cutting edge or modern, like, operator companies that I work for, like, they just, they had a very robust set of technologies and allowed people to really say, like, you know, hey, what, why
45:39 not, what if we didn't do Aries, right? Or what if we, you know, we did all the, all the financial reporting and Power BI. Yeah, sure. Like, the entrepreneurial, right, go about it, right?
45:48 Now, that doesn't mean like it had the right, I mean, we had, it had a really strong tech tech organization, really strong IT organization, but they were still obviously very focused on, like
45:57 making sure that Open Wells was running right and so I you know we still had to be very entrepreneurial about, okay, well, I gotta go out and learn it myself. And I want to take all this data,
46:08 like we're building forecasts and building operations plans and we're running them in an inner-site in the cloud and they're real large plans. I'm gonna post that into a database. I'm gonna run it
46:16 in Power BI or SpotFire, kick out a standard dashboard for executive leadership to run every time we run a scenario, right? Just being obviously conscious about where I wanted to spend my time,
46:30 right? Versus the old school way of bad, I only put in Excel and then built a really complicated, sort of operational financial model, and so it was kind of a sink or swim, like where I just had
46:39 to get good at it, right? It was, I wanted not to waste half of my day job just managing the spreadsheets, right? So, and it was hard, before varsity, I was at Noble Energy, and everything
46:56 was still in S-base and HyperCube,
46:59 and old school like PA models but just like, for. terrible to use. So I just got good at that and I sort of parlayed it into, you know, having to sort of build my own little like mini data stacks
47:12 like at parsley to kind of do these things into like, hey, let's scale this out. Like I have a vision for it for, for Invarius because, you know, as we talked about that job and role and, you
47:22 know, we just ran with it, right? Like, just we got good at understanding like, well, I know, like, having to build a bunch of dashboards and manage, you know, building reports for the CFO,
47:34 the CEO, the COO, like, I kind of can work backwards and get to the data engineering side of it, which I've been learning over the last couple of years now. Yeah. That's how, I mean, just your
47:44 journey into like data cycle, it looks like you even started at parsley as in with a lot of your previous experience of like planning side. Yeah. And then you were actually the Residence and your
47:53 manager for a little bit Yeah, yeah, it's like a reservoir like cleaning. Oh yeah, okay, like the planning stuff, yep. Okay, so a lot of the planning stuff and then like, I guess basically
48:05 like you, something like you were starting to use a lot of data stuff to make sure you have better with that. And that's how that got parlayed into becoming like the data. We're where I'm in now,
48:13 yeah, absolutely. Yeah, I can mean, I think as I mentioned, like, you know, just it was a, it was a, it was a function of, of just making my life easier. Yeah. Right. That's what were
48:23 some things at that time that you, you know, you graduated from Excel to this, to this. I mean, what were some things along the way that - Well, I'd say it was kind of getting into the Power BI
48:31 world. Okay. A little bit, we, it ended up starting like, hey, let's just take data from a source, whether it was open wells or some output from a model and building some nice like, you know,
48:44 power query like flows that have like managed all like the data transformations and click, you know, click refresh and run. And then everything would just populate, right? And we eventually got
48:53 into, hey, you know, this data is getting a bit too big. Let's work with IT. And again, like parsley made it super easy be like, Hey, we need this one thing to scale this out to a larger per
49:02 size. Okay, we'll spin something up. We're actually looking at Informatica or Snowflake, and that's where I was like, Oh, okay, what is that? Like, it started doing some research in and of
49:10 being, they think they just built something in-house. Are they using a lot of SQL Server? Yeah, yeah, yeah. So they'd spin up some SQL Server's for us that we'd work with a DBA to get open wells
49:21 data in, so we had to like better cost data, so we can do our capital planning better, right? So we can integrate that into the planning process And so it's all the little things that I think even
49:29 probably like
49:32 all the inefficient processes about when we were doing like reservoir planning and like ops planning. We've taken this data from here to this data from here and this data from here. I'm like
49:38 building a model. It's always just like manual, right? Sure. But we got to the point, I get parsley where it was, you know, we connected those systems, flow it in, tweak our inputs, run it,
49:50 output was in the cloud, pull it down into Power BI And then, like, you know, then you're really spending most of time doing efficient things Scenarios and planning exercises and like not having
50:01 to do they're 50 of Well, let's wait for you know, and for the excel to stop spinning, right? So
50:09 That that's kind of where Again, we that's where it parsley really loud. I think that skill set and maybe curiosity of like technological curiosity kind of
50:21 Push me into a state of like understanding like okay I just actually fun like that part was actually really cool and then obviously when when pioneer Acquired parsley, I we just weren't in a position
50:31 to want to move to Dallas Yeah, we liked Austin my family liked Austin and Fortune enough like I was like oil and gas adjacent and they're sweet like I'd love to do that so It's thankful that they
50:43 gave me that opportunity. I wasn't like I was coming like to you know I was some data engineer from from you know some other SaaS business like yeah, I think they appreciated the it's gonna say
50:53 you've got the domain You know, get domain expertise, right? Very hard to train that someone on that. I think that helped a little bit, 'cause I understood the customers, understood the products,
51:02 understood all the little things. 'Cause I was gonna say from that same one, but it's like, it is more like, you know, serving a SaaS company. Yeah, yeah. But I mean, how much does that
51:09 domain next? If you get out of it. Well, it's funny enough, like if you think about a SaaS business, right, you've got a bunch of customers paying you something and there's a finite, there's
51:18 like,
51:20 it's some point that subscription or their contract ends, right, and you either renew them or they go away And if you never renew any pipeline, ie. drilled any of your, you know, pud wells, you
51:32 just got this blow down curve of revenue, right? It's your PDP base, essentially. You're broke. You're broke. And so it's very much a very similar business. You've got products that you've got
51:42 some sort of white space about, right? You think you know that Conoco could potentially pay you this because you have all these products that fit their, you know, customer profile, right? So
51:52 maybe they're paying you X, but you know, it's X times two that you could go after. Sure. Every customer has. some amount of captureable white space out there. And so we build models to kind of,
52:03 actually one of the cool tools we're doing now, these data app tools like Retool, I don't know if you've ever heard of it. A lot of it, yeah. We actually built some really cool custom apps to
52:12 allow our sales team and our segment leadership teams to better forecast and predict their white space based off their current buying behavior, right? So 'cause you can look at all the population of
52:23 similar accounts, and we built a really cool app It's like the combination of like a GUI interface with Python and post-grace databases that connect to Snowflake, and you can write these scripts and
52:34 like an application base, right? It allows them to go in and like it calculates a distribution and does a bunch of math in the back end. And it says, hey, like we think that Prism has this much
52:45 available out there, right? From our current customer base, right? So,
52:52 but having that knowledge like understand, to right,,
52:55 I don't think that they would actually buy that, right? Or, you know, I know what the data kind of looks like when I'm looking at a user
53:01 doing workflows in Prism, I can kind of be like, okay, that's important. That's not important. Let's build a model for understanding like churn behavior. Like why would a geologist or a land
53:13 person or a company of, you know, of X size or has this many wells remaining? Why would they not or not want to expand in Prism or just not hate it, right? Yeah. So having that kind of context
53:25 because I've done it and I've worked, I've used, you know, IHS and I've used in various or Prism in the past, you know, at a former life. I just kind of knew it. Sure, yeah, no, it's totally
53:37 better. It's fun building tools where you were at one point, the end user potentially, right? Like I'd do this very similar stuff with Collide and it's like, they're asking me or on the marketing
53:48 side, like what podcast or webinar should we set up next? like what's a good topic. Yeah.
53:55 you're our audience to tell us. Yeah, and it's like, well, this is a lot easier than coming in cold. And yeah, you also have like, again, that learning curve of if they were to hired someone
54:05 that was strong, you know, just a data person, but didn't know the front end on product that is so tricky because you can solve the problem that you think you were trying to solve without actually
54:17 adding any value to the customer, what they actually wanted, right? It's like, if you've ever hired consulting developers, you know exactly what I'm talking about. It's like, hey, I want it to
54:26 do this. And then they give it back. And it does exactly what you told it to do, but not in the way that you wanted it to happen or in any kind of intuitive way. And then it's like, okay, well,
54:35 this sucks. Yeah, yeah, but. So, me really the biggest thing, the learning curve was just understanding kind of just SAS terminology, how the business model works a little bit, but it's not
54:48 overly complicated at the end of the day So, um, that parts, that parts, uh, relatively straightforward now. Nice, yeah. Okay, so coming back to the data stack a little bit, 'cause I think I
55:00 was talking to Zach Warren, we had him on him a few months ago. And he's like, so are you gonna do a post mortem on like your data stack at GME? He's like, that's not a bad idea. It is a good
55:12 idea. And it's just, even just for me personally, I think he said he did it at another job to wherever, and he never published it, but like even just if you wrote it for yourself, it says no, I
55:22 can only imagine how good that would be just for internal, all the shit that you would have done to really hear not done or recommended not to do. But I mean, obviously I very fortunate to have had
55:33 a chance to do it greenfield when I did it, and you know, chose and I wouldn't regret what I chose at the time, but if I was gonna do it today, would those be the exact components that I would
55:42 choose? I mean, or if I said you had to change all three, like if you had to change snowflake, if you had to change DBT and you had to change 5-tran, how would you do it? I don't think about it.
55:55 That's probably the problem with SaaS, right? Is that there's so many damn options, right? And do they all really have any differentiating barriers? Not, you know, maybe I'm pricing for the
56:05 most part, but I'm assuming y'all are grandfathering into DBT
56:10 as well. Like DBT cloud, the pricing, 'cause remember there? Yeah, we were, and I, at the time, they were just gonna change everyone, right? And I said, uh-uh, no, no. I went right at
56:18 Tristan too, and the Slack channel. Yeah, yeah, I know. But I don't think I'm gonna go, I mean, I think, fortunately, even they've changed their pricing model a little bit, where you can get
56:28 to their enterprise, and you can choose to pay for the semantic layer, which only charges you if you use something, right? So as long as you're not going crazy, and again, you need to be good
56:42 steward about your data model because you have 8, 000 models, and you're running them on power increments. That's on you.
56:50 Do you really need that kind of frequency? Better run at midnight Probably not, right? Um, but if I had to go back, I mean, you know, I think, I think, or if you had to do it now, like,
56:60 yeah, yeah, you know, not change it then, but like, let's say you got an opportunity. Same exact thing. Now, would you choose the same things or if I forced you to change, what would you
57:08 force me to change? Yeah. I want to keep this question in the rotation. I think it's a good question. Um, I still pick DBT, uh, you know, um, say versus like, say it's equal mesh. I mean,
57:19 I guess I have, it's not mature enough probably. It's not mature enough I mean, it's also hard to say if you'd never test or play with, like that's the, that's the kind of girl. Well, so the
57:27 interesting thing about SQL mesh is that you can, it can import a DBT project and it talks DBT, but then it's, they do things a little bit different. It's really different. Yeah. It's just not
57:35 as mature yet. It's not mature. I mean, the whole virtual environment thing is kind of interesting, but like I think, you know, we're, my team is probably not fixing it to really get the, get
57:44 the impact of that or probably work with the right kind of models and data to really even care about needing a virtual, several virtual environments, right, that they offer. and now that they vote
57:53 to Columbia and he's just like, what are you really getting? Yeah. So it'd be hard not to just say. I mean, you know, there's so many things to support DBT. Yeah. Similarly, so many things
58:03 are tied into snowflake. Yeah, it'd be hard to not go that direction because it is so tightly coupled with so many important components of the business. Although I do keep fascinating or thinking
58:16 about like, Mother Duck, because I have - It depends on what Mother Duck is, just a few people Yeah, so DuckDB is obviously like this, this sort of compute program or language around - Open
58:27 source, open source. And saw Python R, whatever. And you can work with, even SQL, it works really well with like blob storage location. So a lot of people who are moving more towards like a,
58:38 like house architecture, right? Where everything's just stored in file formats, right? And S3 or Azure Blob or the like.
58:45 It works really well
58:48 with that type of architecture but at the same time gives you. just sequel at the end of the day. But what they, but obviously the, the promise of it or the ethos, right, is that data's medium.
58:60 Data's not large. Yeah, like we started off talking about it. Yeah, like we started off talking, right? Like even, even us, we don't have one
59:08 compute cluster running and stuff like that's bigger than an extra small. Yeah. Just don't need it. Yeah. But, and so obviously like there's tons of people whose computers are very ridiculously
59:19 good. Yeah. But even better than some of the things you would might have provisioned. Provisioning the question. Right? And so it pushes all that down when it can to the local, you know,
59:26 compute engine and runs the query there and it's optimized and it's, you know, it's extremely performant. Right. And
59:34 allows you to develop in a way that's again super quick and easy, right? Now mother duck though,
59:47 it took that concept and they managed services, right? Hey, we got you sort of a managed service database environment, like a snowflake so that you can do standard analytics. architecture things
59:51 that you would at scale. Whereas like, you know, DuckDB might be more like, you know, home brew type of things, right? People who just, you know, who are analytics-turious, right? Yeah, I
1:00:01 mean, for some people that aren't, I mean, DuckDB is basically SQLite, but for analytics, it's like a columnar, you know, O-LAP kind of engine, but super fast and perform it. Super fast,
1:00:10 perform it. So I would think about it. I mean, I, you know, Yeah, for me, it's like with mother duck, like, how would I connect Spotfire or Power BI too?
1:00:21 Yeah, it is a little limited still. And even with Databricks, that was the thing. When I was trying, it was like some Simba ODBC driver thing. Like, but it was like, I couldn't connect easily
1:00:29 to Databricks in like the stuff like driver work. Yeah, so I think that'll work. So, you know, there's, I do think about it a ton 'cause I do like the cost model quite a bit. Yeah. It's nice
1:00:42 that you can sort of individually prescribe certain users a certain amount of like compute. Okay. Right? So you can say,
1:00:50 heavy intents and you can allocate them different amounts of credits versus others. So I think you can be super efficient with that cost model and pay for only what you use, right? But again, so
1:01:01 everything is just so tight in to snowflake. It's difficult to think about going in another direction. Even Databricks, our Databricks head guy is always like, When am I gonna get you over to
1:01:11 Databricks? I'm like, I don't know why I would. Yeah.
1:01:15 Just because, again, my use case, the analytics use case is just so nice with that I get why they use it. Yeah. You know, and they got a bunch of Spark engineers. No, I mean, it's - And
1:01:23 they've just been doing that for a long time. It's using the right tool for the shop, right? Like, that's what it will look like. What's funny enough is that my data engineer just started using
1:01:31 snowflake notebooks as like, Man, these things are great. Yeah, no, we started using a couple of them. 'Cause you can deploy a pipeline, like in Snowflake, but like chain together, like
1:01:41 Python or SQL again, like similar to HEX, where I can reference the Python sell above through SQL. And boom, boom, boom, and I guess basically now it's like, probably a short procedure. Yeah,
1:01:50 absolutely. And I get to get pushed into the thing. That's what we were talking to Stephen. Everything is compiled down to the same bytecode. And you can go ahead and write. And other things I
1:01:59 think that I obviously, I think that Snowflake has, and even DuckDB's got some really cool ones, but they sort of push the edge on what you can do with SQL. Data bricks isn't like doing anything
1:02:07 cool with SQL. Like there's just standard SQL. Like Snowflake's got some really awesome Snowflake only. Yeah, the SQL type of trigger and everything Yeah, you know, qualify statements and like a
1:02:17 min buys, max buys like some really cool things that like would have taken you triple the amount of like SQL code to pull off and they minimize it. Or, you know, even DuckDB, I think you can do
1:02:27 like function chaining like you wouldn't Python right in SQL. So there's just like really cool things that both of those platforms are doing. Yeah. So I think, you know, I think I'd probably
1:02:35 still pick Snowflake just because of again, our use case and you know, thinking about. Well, that even was like the governance and like the replication They make so many things so easy that you
1:02:45 don't have to manage it.
1:02:48 And I'm still thinking about the end users, right? Most of my end users still are very much like, give it to me in Excel. I mean, let me just like put me to the right data, data set, I'm gonna
1:02:56 pull it down, I'm gonna play with it. And to your point, like mother duck still is like growing, I think they're sort of outputs and integrations. It's like even then I probably be like, Hey,
1:03:07 you know, it's not, we're not fully like often, even Excel. Like even though I had a demo yesterday of equals, like when it's over the cloud-based like spreadsheet, like functions that have all
1:03:17 the Excel primitives. Similar to like Sigma. Sigma, yes, similar to those. And so like I keep thinking about those opportunities because the only thing I don't love about the Excel relationship
1:03:27 with Snowflake and Power BI is like that. Analyze an Excel feature, like the O-WAP connectors, which, you know, the ODBC drivers are just so old and inefficient, like when it has to like push
1:03:40 back like an Excel query into the Power BI service, like those query skin just like the amount of optimization to do is just so janky.
1:03:47 When are we, when is there, like, why are we still using ODB? ODB. So that's a good question. Cause I wanted to get in a little bit is like, there is now, and it's not going to happen fast
1:03:59 enough, but like there is a new like ODBC is what, how far was Oracle database? Yeah. But like, but ODBC, JDBC passes data in rows, which is how OLTP systems work and work. And now we're doing
1:04:12 these columnar things. It makes no, it literally makes no sense. Cause we're running things columnar here Yeah. And then we're sending it across the wire and rows back to be transformed back in a
1:04:22 columnar. So there
1:04:24 is now the arrow database, ADBC, basically. Cause Apache Arrow is like a columnar format for passing data around. So now, I mean, there is an ADBC, there are ADBC drivers now. And I think say
1:04:37 like snowflake Python driver connector uses that to pass the, you know, but again, can we get Spotify or can we get Power BI? Microsoft? Yeah, Microsoft. Guys, come on. I mean, even like
1:04:48 trying to like, we have a couple of product teams who like want some data out of Snowflake and they want to put it back into their like provisioning system, which is a MySQL's like database. And
1:04:58 then it's like, I got to connect you through Python, but it's all ODBC and it's like it takes forever. Yeah. You know, so it's my solution always like, well, it's just like find an alternative
1:05:10 route, right? And so if, you know, do people really just want a spreadsheet? Right. If there's something that just doesn't, that does what Excel does, but, you know, handles volume at, you
1:05:20 know, volumes of data out of the cloud that, you know, that's the main reason on that crap. Probably has an infinite number of rows. Yeah, I know it does, they called it like infinite query.
1:05:29 Yeah. Right, and so we work, I mean, you know, I don't know if like, because we are a Microsoft organization, we got teams, we got everything. And Excel you, know, obviously it's just fair.
1:05:40 and it's very, it's bomb practically free. Well, and for me, I want things reproducible. So like going back to what you were saying originally, as long as you're not pulling a CSV out of the ERP
1:05:48 and another one here and putting them in the Excel, and they do that, you know, once a month and they recreate that process, like if we can just give them that output, bam, right into a table,
1:05:57 it's probably good enough for the most cases. And then they can build their pivot tables or even form those off of that. No, I mean, I asked because our current collide dashboard is down because
1:06:07 Microsoft is having ODBC We had it too, we had it too, yeah. With our dashboard and are actually earlier this week, my one drive, that's a whole nother. But my one drive started shooting the bad,
1:06:17 but it's like in order for me to sync my dashboard from my Power BI file on my laptop to the cloud so that everybody gets to see it. I've got to set up an ODBC server, like this is so stupid. And
1:06:33 certainly like,
1:06:37 I looked and experimented heavily
1:06:40 It just isn't there yet. I think they're doing great things on the analysis side, particularly with being able to do right and do things with just
1:06:50 file formats. You can almost directly query Power BI really efficiently, just the file format schema. That's pretty cool, but getting data into fabric and working efficiently, they still want you
1:07:03 to use Azure data factory stuff or data flows Don't they like some SQL mirroring stuff now, though? They do have mirroring, like, so you want to have the data. It could be in Snowflake, or it
1:07:15 could be like an S3. Is that like a parquet situation? Well, no, no, this is like, I'm still fuzzy on, but they like SQL server mirroring, which I think is huge, because it's a complete
1:07:24 virtual replication, right? It's like the data is just there. So it's just an instantaneous feature? Yeah, yeah. So you don't have to do, like, if you did the data staging up, like, in the
1:07:34 alternative space, like in Snowflake or whatnot, you could write that down But you know, then you're paying for a couple of different things, which is not the end of the world. Well, but I think
1:07:43 this lake house thing is getting more and more interesting and maybe if I were to do it again, now, because even I think Snowflake apparently, at least if you believe the sales stuff, gets really
1:07:51 good performance on, especially on their managed iceberg and stuff like
1:07:55 that. But now there's
1:08:09 the one like integration with Snowflake and where you can read the one lake. So you could have, again, the whole promise of the lake house things that you have in these catalogs, data lakes
1:08:10 forever, but then I can hit it as whatever computer engine I want and I can hit it from Snowflake Databricks, DuckDB, Spark, whatever. And I thought about that too.
1:08:17 My opinion was, well, my realization was, is that
1:08:22 the storage benefit is not really all met. No, storage is nothing, right? But our analytics community probably isn't that robust to where it's like they have a bunch of different tools, like let
1:08:36 them like pick their own tool and compute with their own tool. Yeah, we're just not, we're not into that state where they're like, I got the one person a day to bracing at the one person with
1:08:44 their custom notebooks. And well, I think, well, I think George Frater maybe talked about it in that article. We talked about to start, but, you know, five Tran writes to blobs now, or they
1:08:53 write to the S3 or the Azure blob and stuff. So now if they're managing the creation of that, you know, those tables and like catalog and everything in an efficient way, like, because then you're
1:09:01 not paying because, you know, especially if they say with at least snowflake, you're paying for ingest. Yeah, you are. And again, there's no value in that compute you're paying for. It's a
1:09:11 it's the actual transformation logic. You are paying for the row. Yeah, the active row. But you're not getting double. Because like right now I'm paying for the row and I'm paying snowflake
1:09:20 compute. Yeah. Whereas like this, you know, just writing to the after blah, blah, blah, blah. Which is what I kind of alluded to is like, kind of, you know, they want you to pick those
1:09:28 transformations in five train because they're not going to do the most efficient way. Yeah. Like we tried some of those things out. And then I saw my I compute spike up, like I have normally.
1:09:39 killed it immediately and I built the same thing in DBT with like
1:09:41 a similar package and it's like barely noticed it. So I was like, what are y'all doing? I know there's like a quid pro quo thing where you're like, yeah, hey, come dust and we'll do this thing.
1:09:51 And, you know, it's just all works. And then snowflake is like gotcha, you know? And like, I don't know if they got cutbacks or anything, but they probably do. For sure. But at least that
1:10:00 gives you some of the portability or, you know, or if you could do, you could actually write tests themselves writing it with like a duck DB and then maybe push those more to like, so that's
1:10:10 definitely something that I would think about. But in reality, I probably wouldn't change anything. I think the only thing I would change is like, just being a bit more methodical about how we
1:10:17 built our DBT models. Yeah. Getting a bit more organized, trying to be a bit more pragmatic about saying no to people, right? Find out what maybe I'm value and what wasn't. As you had more
1:10:27 developers, too, like keeping a certain standard. Yeah. You know, like make sure there's not, 'cause the whole idea of DBT also is that you don't, the dry code, right? You don't repeat
1:10:35 yourself, right? And then like, but if you get multiple people, always risk that you've ridden the same thing in a couple of different places, right? So we definitely have to go back quite often
1:10:43 and clean up a little tech debt and just always think about like, you know, trying to do the quickest thing that you have to do to get that one thing built. Let's be sure we're following the right
1:10:56 domain model that we think needs to exist for the business and keep it very clean. And so that's probably where I would have sketched out a complete domain model first of the business. But that's
1:11:07 the problem. Like speed at the time of the implementation, it's always the hugest value that I think we gave our company, especially, you know, was like we,, like between five trends,
1:11:16 snowflake and DT, we were stood up. I mean, literally, I mean, when we decided what we're going to use within weeks, like, yeah, I can, I mean, even anything about what data warehouse
1:11:25 implementations used to take and the money and that's caught, I mean, just absurd, you know, like, but with that speed comes the trade off. So like, you know, we didn't think about a day. I
1:11:35 mean, I would have built maybe more dimensional models for certain domains and then built it, you know, but that do it take work to go back and do now. But that's the you don't know what you don't
1:11:45 know until it's too late. Yeah, absolutely. But shit, man, that was a quick hour. Yeah, real fast. It was good stuff though. Yeah.
1:11:56 Typically off topic though, what you guys normally covered. But I mean, it's this is more what we tried to like. This is why we want to go with it where people are learning about different tools
1:12:03 and stuff they can use. No, we want to geek out, man. Yeah. We'll jump into the the speed round here at the end. I've got we were talking about earlier. What's your favorite video game or board
1:12:15 game? Either one since you're wearing the kangaroo future. I got to ask. Man, you know,
1:12:21 I cut my teeth and spend a lot of time or something like on the old, you know, college football, you know, games living in building dynasties and creative characters. And so hard for me not to
1:12:31 buy the new. And I haven't yet because, you know it's sort of like is it a present for myself or my like, you know, like, you know, like, you know, like, daddy said, we can't play. And
1:12:39 we're doing it like, it's got a good, you know, um, so I haven't, you know, that might be, I don't know, might be a Christmas present or something, and I'll, I like scribble my name at the
1:12:49 very bottom. Yeah. Uh, I'd probably, probably that, you know, just, just sports games in general. Yeah. I never, like, I, if I do, if I did, like, I did this thing, because we did, I
1:13:00 grew up in the video game age and my wife doesn't understand it. But, um, whenever we, we were going through, like, raising our kids and they were infants, like, I would, I would be like,
1:13:09 Hey, I'll take the nighttime duty. Cause like from nine to midnight, and I knew they were going to wake up. I'm like, I'm going to get some gaming in, but it would always be like just like one
1:13:15 player single story games. Cause I'm just like, I just need like a start and an end. Yeah. I don't need something that like, I have to come back to all the time. So like, um, I would usually
1:13:23 find myself with some of those kind of things too, but mostly just mostly college football or, you know, Halo back in the days, we did that, right? We jiggle up all the, all the, all the land
1:13:34 connections and like you get by the Xbox is in the house and a bunch of. Man, that's, I'll tell you what, that is the thing that I feel like they have really screwed up on with the new games, is
1:13:44 that you cannot co-op on the same console anymore. And it's like, this is what my whole child is. Like we used to play 64, four controllers D on the same TV, or like, and then now you can't do
1:13:58 that anymore. What are you gonna do with it? Yeah, golden right, you know.
1:14:02 I know Yeah, anyway, that's, yeah, like I get. I love the online gaming part, like God and all this stuff. And then I'm fascinated, I have not played a sports game that is online yet, that
1:14:15 has like, dynasty online and things like that. So I'm very curious if I
1:14:19 do end up getting it, how that's gonna shake out, but what you got? Let's say open source package that you're really excited about.
1:14:27 Oh shit. I know that's a heart on the spot one, because we all look at shit all the time but.
1:14:37 or even one that you would recommend other people check that you've had a lot of success out there. Well, yeah, I think you brought it up early. I think the great expectations one is really is
1:14:43 really super powerful to get with really early. If you're going down this,
1:14:50 you know, path, right? Because I think data quality is super important to get right early, and but it's hard. It's hard to look at that much information and like see where it's dirt, like where
1:15:01 it's messy, right? So you just need something, you need to watch dog, and you need things that can tell you very simply, hey this is in compliance or in tests or not, and in great expectations
1:15:12 is really
1:15:15 nifty. There's one off the top of my head, I can't remember the name of it, but there's a couple that help you actually build, sort of
1:15:25 automate sort of this source and staging process, like
1:15:28 getting all your source files built out and stuff like that, which is something you never think about doing until after the fact that you don't want to do it. Hey, what's the description of that
1:15:37 call? I
1:15:39 can't remember the name of that package, but I would say covering that base, covering the data quality
1:15:48 base, get in that place early, right? And there's great open source packages to help you with that. So you just don't do it. It's a way for me to use that. You just can't ever come back to it.
1:15:58 There's always things to do after the first. And they're always more important than documentation. They are, yeah Unfortunately, unfortunately, which is where, hopefully, again, the promise of
1:16:09 LLMs will help a lot with that. Yeah, no, for sure. What's great now, what I typically end up doing myself is that if I have raw data in Snowflake and I have a table, it gives you obviously the
1:16:18 schema, right? It gives you all the information. Here's the columns and here's the types they are. And if I go in a chat GPT and I say, hey, here's my schema, it helped me build a thing from
1:16:28 this data set, and here's where it came from. It came from Stripe Okay, cool, different things, man, it'll give you, like. this like sourcey ammo and it would like to start everything. And I
1:16:37 had to do it and then I'm just like sweet, copy, paste, save, run. I feel the same way with, uh, with, uh, putting notes in the code, right? Yeah. I can take my script and dump it into GPT
1:16:48 and say document this. Yeah. Add error handling and add rate limiting. And actually this one of the things I actually really liked about mother deck was there like fix it, like it'll do that, but
1:17:00 also add like the commentary and a little bit about that. And it's really well. GitHub co-pilot is also extremely good, extremely good at it. Like it's almost scary good when I'm like writing
1:17:10 something and then it'll like pop up like it'll suggest. I'm like the whole thing exactly what I want to be like, I can't what tab. Yeah, tab go like it was it's insane. Like so it's definitely
1:17:23 it's made life extremely easy. Yeah, that's another pro tip though. I don't feel like enough people realize that if you give GPT the like Language models are all about context. So if you give it
1:17:34 the context of the table or the data set that you're trying to transform or do something with, it gives you a way better answer. And it can even give you files. Like I backed up our entire bubble
1:17:46 database. I exported it all to Excel, took the Excel, gave some sample rows and columns to GPT and said, okay, give me the SQL file. And it just gave me the SQL file. I'm like, holy shit, I
1:17:59 didn't even have to write anything. I didn't write anything Yeah, and there's sometimes where I'll, if I'm like just beating my head about some model, I need some output and I'm just like, I'm
1:18:08 out of my depth here. I'll just be like, hey, this is where I started in a CSV table and I'm like, this is where I wanna be. And I get like, hey, here's where I started. Here's where I'm in
1:18:18 debt. And they're like, oh yeah, I see what you're trying to do. And it just like does it. And I'm like, am I gonna try this? Oh, yeah. Where'd that actually work? And I'm like, then I
1:18:25 learned something. I'm like, okay, I could have done that sweet. There's a design pattern Right. So. Need to get you on the cursor train. Yeah, no, me and me and one of my
1:18:36 tech cohorts that helps my team, we started looking into cursor. We just signed up for the enterprise last week and have an active, we've deployed it to all our developers, we will only be hiring
1:18:48 developers in the future, not for cursor, but under the, it will be in the job description. We are hiring developers that are comfortable using AI to augment the speed of their performance or
1:19:00 whatever, just because we're so small that like 10xing a junior dev is very impactful for us versus, you know, Yeah, absolutely, absolutely, no. And we, we're looking into that too. Although
1:19:11 I guess we are GitHub enterprise company, we have co-pilot, enterprise code space is really cool, but for nothing that really awesome thing about cursor. It's pretty slick, man. And the
1:19:22 enterprise, by default, everything's on private. There's no like open anything. It's really fascinating I barely scratched the surface with it, devs love it because you can reference anything,
1:19:34 you can reference the files, you can reference functions, you can everything all right there. And so it's, it's pretty slick. Yeah. But last one, you want to do the honors? What's your
1:19:44 favorite place to stop between Austin and Houston? Oh, that's a good one, Bobby.
1:19:50 What's your favorite Kalashi? You know, it's funny. I hadn't stopped here yet, but that new press six or whatever. Yeah, that they're kind of like buckies is kind of like that. That's actually
1:19:60 really nice. Yeah. I really like going in there. It's like, it's like, it's like buckies without the, with the convenience, you know, the music parks sort of scene. Yep. Of course. I,
1:20:12 which way, which way do you come to 90 or 71 usually? I usually come to 90 because I usually end up coming to this side of town. When we do go, my boys play a lot of travel baseball. A lot of
1:20:26 times we get to go to Tomball for Houston tournaments, and so we will go.
1:20:30 I'll usually go to Bastrop and then cut through that, like, nice, like, scenic route, and then hit the top side. I said, 290, nine minutes, 71. Usually comes up. It's anyone to 10, but
1:20:39 then I will come to 90. Okay. I'm gonna go that route just 'cause it's a more pleasant drive. Yeah, I know exactly. It's chill. Well, I tend between, I want to see the CO8 Acadia just
1:20:49 absolutely miserable. Like, 'cause when I come from like Sugar Land area, I cut through like Eagle Lake and go up and down. I barely go north to 10 and then over, or you come around it. I go
1:20:57 like highway 90, like alternate, whatever, back to the East Bernard and Eagle Lake. And then like, you just take this back arrow and also then it's busy out and then you're on I-10 for like,
1:21:05 like, you're on I-10 on like two exits. Yeah, there's sometimes where there was like, either a major wreck and we just ended up doing that. Sometimes you have to go to Rosenberg for baseball and
1:21:14 we'll take it's Eagle Lake. Let me know if you go to Rosenberg. You could have Diamonds of Daily or Nice. Which is like, maybe one of the best baseball parks. It's very awesome. In Texas, I
1:21:22 think. That's awesome. Yeah, I like, I like Hrschka's on 71 right there. Yeah, so that, I was up to say like, we, parents always lived in Austin and we were when I was in Houston, we would
1:21:32 go back and forth a ton. It was always just roost good stuff, right? Like just pop in there and get a collage here too. And, you know, that was where we typically start. Yeah. I feel like
1:21:41 there's more persecs or however you say it, persecs, I think. Yeah, there's more they've been popping up. They're not going after that. You know, they're trying to Pepsi it, you know? Yeah.
1:21:51 Awesome, man. As it should. No. All right. So yeah, well, I mean, just thanks for joining us. Absolutely. I'm glad to come down and I'm glad to put a, you know, a real face. I know,
1:22:03 right? Yeah, absolutely. Yeah. Absolutely. And this is good. I mean, catch up against sometimes. Yeah, we should. Absolutely. I mean, it's like, I said, if you're in town for baseball,
1:22:11 I mean, I will. Bobby will probably be on the fields. Yeah, my daughter has some tournaments that time of the day this year or so. This, you know, the thing about this being in this job is that
1:22:20 like you get whiplash with how quick it changes. Yeah. So I'm sure if we did this in like six months, like, please have it. I'm physically different topic about it. I don't understand. So. It
1:22:30 keeps you on your toes, at least, at least that. Absolutely. And I'm generally, I like that part. I'm naturally just like. I guess one thing we didn't know about, where can people find you?
1:22:39 Uh, just LinkedIn. LinkedIn, LinkedIn. Yeah, you know, I don't, I don't socials much. Yeah. Yeah, that's fair. That's awesome, man. Thanks so much for joining us. Yeah, thanks guys.
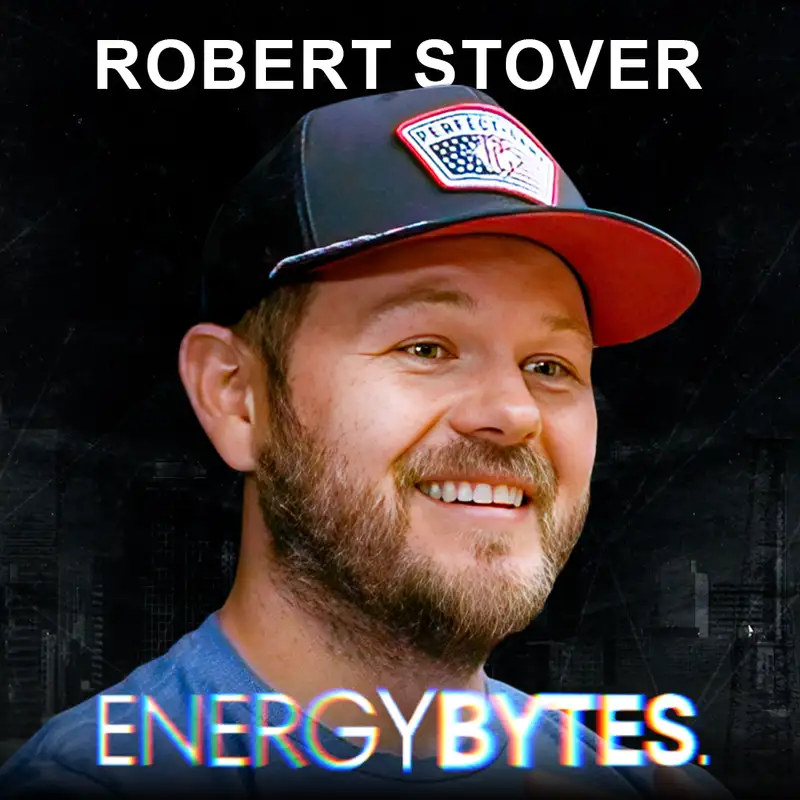